A novel clustering algorithm based on multi-layer features and graph attention networks
Soft Computing(2023)
Abstract
Clustering is a fundamental task in the field of data analysis. With the development of deep learning, deep clustering focuses on learning meaningful representation with neural networks. Ensemble clustering algorithms combine multiple base partitions into a robust and better consensus clustering. Current deep ensemble clustering algorithms usually neglect shallow and original features. Besides, rarel algorithms use graph attention networks to explore clustering structures. This paper proposes a novel Clustering algorithm based on Multi-layer Features and Graph attention Networks (CMFGN). CMFGN obtains multi-layer features through the hierarchical convolutional layers. Moreover, CMFGN combines the co-association matrix with original features as the Graph Attention Networks (GAT) input to obtain consensus clustering, which reuses original information and leverages GAT to inherit a good clustering structure. Extensive experimental results show that CMFGN remarkably outputs competitive methods on four challenging image datasets. In particular, CMFGN achieves the ACC of 82.14% on the Digits dataset, which is almost up to 6% performance improvement compared with the best baseline.
MoreTranslated text
Key words
Graph attention networks,Deep ensemble clustering,Neural network,Unsupervised representation learning,Feature extraction
AI Read Science
Must-Reading Tree
Example
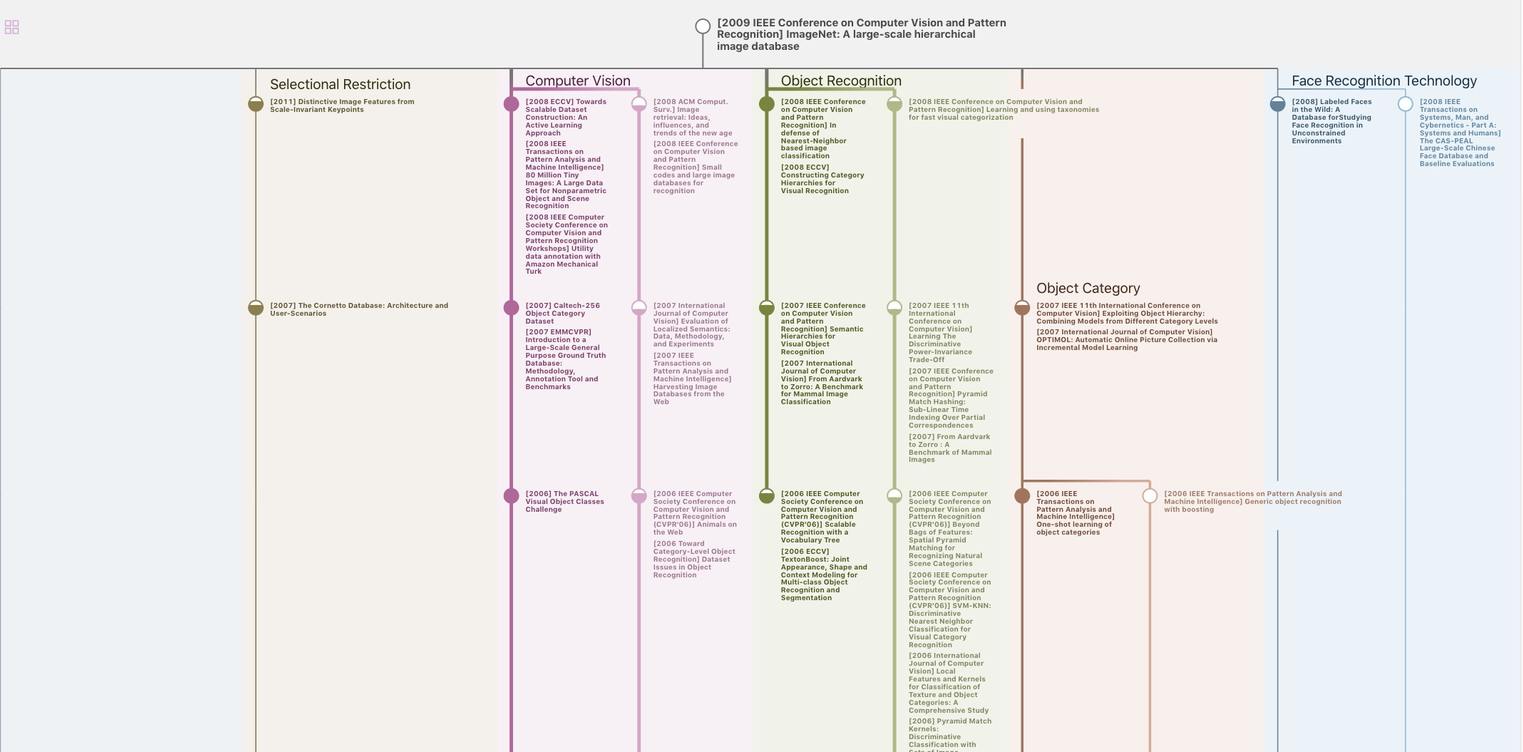
Generate MRT to find the research sequence of this paper
Chat Paper
Summary is being generated by the instructions you defined