Encrypted federated learning for secure decentralized collaboration in cancer image analysis
MEDICAL IMAGE ANALYSIS(2024)
摘要
Artificial intelligence (AI) has a multitude of applications in cancer research and oncology. However, the training of AI systems is impeded by the limited availability of large datasets due to data protection requirements and other regulatory obstacles. Federated and swarm learning represent possible solutions to this problem by collaboratively training AI models while avoiding data transfer. However, in these decentralized methods, weight updates are still transferred to the aggregation server for merging the models. This leaves the possibility for a breach of data privacy, for example by model inversion or membership inference attacks by untrusted servers. Somewhat-homomorphically-encrypted federated learning (SHEFL) is a solution to this problem because only encrypted weights are transferred, and model updates are performed in the encrypted space. Here, we demonstrate the first successful implementation of SHEFL in a range of clinically relevant tasks in cancer image analysis on multicentric datasets in radiology and histopathology. We show that SHEFL enables the training of AI models which outperform locally trained models and perform on par with models which are centrally trained. In the future, SHEFL can enable multiple institutions to co-train AI models without forsaking data governance and without ever transmitting any decryptable data to untrusted servers.
更多查看译文
关键词
Federated learning,Homomorphic encryption,Histopathology,Radiology,Artificial intelligence,Privacy-preserving deep learning
AI 理解论文
溯源树
样例
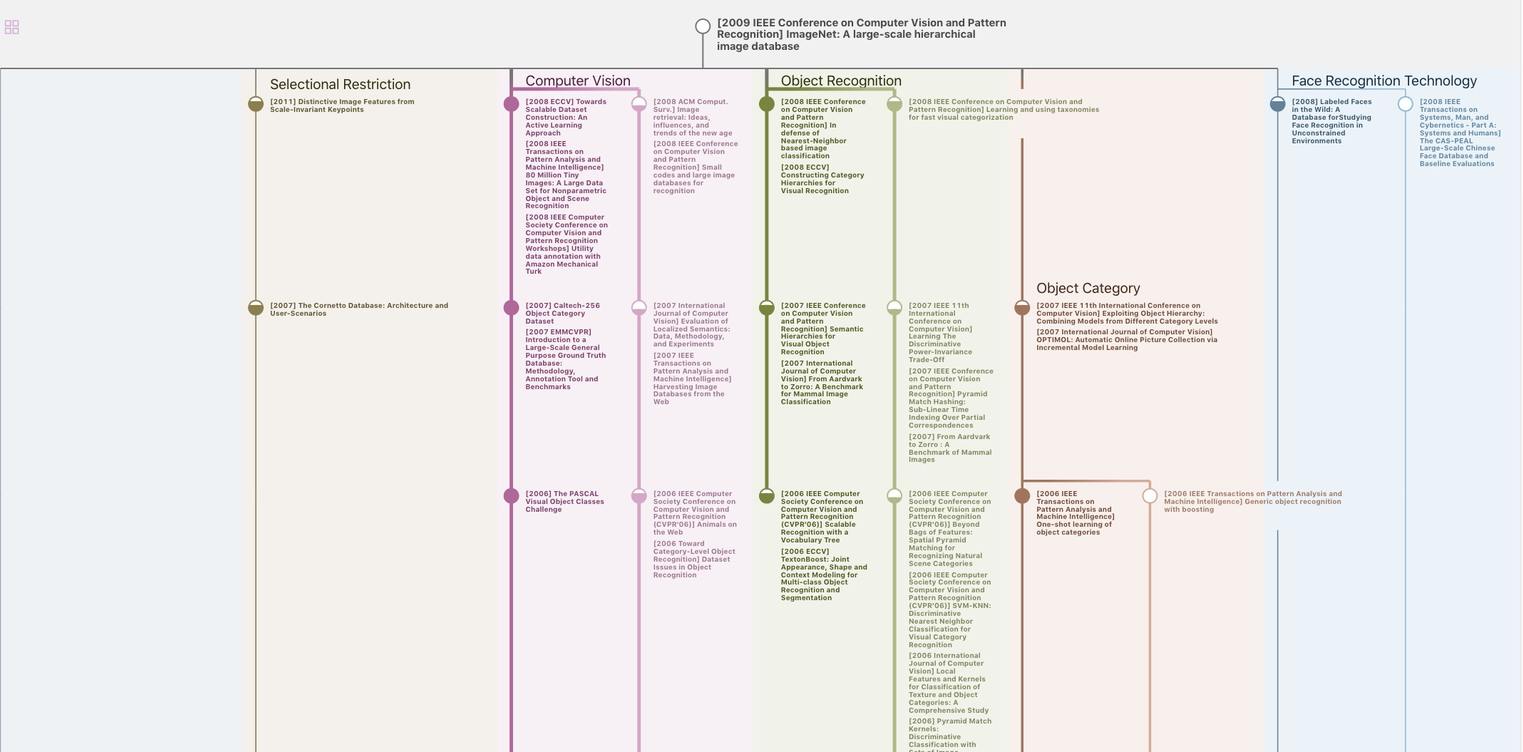
生成溯源树,研究论文发展脉络
Chat Paper
正在生成论文摘要