Deep Learning for Automatic Segmentation of Vestibular Schwannoma: A Retrospective Study from Multi-Centre Routine MRI
medrxiv(2022)
摘要
Objective Automatic segmentation of vestibular schwannoma (VS) from routine clinical MRI can improve clinical workflow, facilitate treatment decisions, and assist patient management. Previously, excellent automatic segmentation results were achieved on datasets of standardised MRI images acquired for stereotactic surgery planning. However, diagnostic clinical datasets are generally more diverse and pose a larger challenge to automatic segmentation algorithms. Here, we show that automatic segmentation of VS on such datasets is also possible with high accuracy.
Methods We acquired a large multi-centre routine clinical (MC-RC) dataset of 168 patients with a single sporadic VS who were referred from 10 medical sites and consecutively seen at a single centre. Up to three longitudinal MRI exams were selected for each patient. Selection rules based on image modality, resolution orientation, and acquisition timepoint were defined to automatically select contrast-enhanced T1-weighted (ceT1w) images (n=130) and T2-weighted images (n=379). Manual ground truth segmentations were obtained in an iterative process in which segmentations were: 1) produced or amended by a specialized company; and 2) reviewed by one of three trained radiologists; and 3) validated by an expert team. Inter- and intra-observer reliability was assessed on a subset of 10 ceT1w and 41 T2w images. The MC-RC dataset was split randomly into 3 nonoverlapping sets for model training, hyperparameter-tuning and testing in proportions 70/10/20%. We applied deep learning to train our VS segmentation model, based on convolutional neural networks (CNN) within the nnU-Net framework.
Results Our model achieved excellent Dice scores when evaluated on the MC-RC testing set as well as the public testing set. On the MC-RC testing set, Dice scores were 90.8±4.5% for ceT1w, 86.1±11.6% for T2w and 82.3±18.4% for a combined ceT1w+T2w input.
Conclusions We developed a model for automatic VS segmentation on diverse multi-centre clinical datasets. The results show that the performance of the framework is comparable to that of human annotators. In contrast, a model trained a publicly available dataset acquired for Gamma Knife stereotactic radiosurgery did not perform well on the MC-RC testing set. The application of our model has the potential to greatly facilitate the management of patients in clinical practice. Our pre-trained segmentation models are made available online. Moreover, we are in the process of making the MC-RC dataset publicly available.
### Competing Interest Statement
Funding was provided by Medtronic. SO is co-founder and shareholder of BrainMiner Ltd, UK.
### Funding Statement
This work was supported by Wellcome Trust (203145Z/16/Z, 203148/Z/16/Z, WT106882), EPSRC (NS/A000050/1, NS/A000049/1) and MRC (MC/PC/180520) funding. Additional funding was provided by Medtronic. TV is also supported by a Medtronic/Royal Academy of Engineering Research Chair (RCSRF1819/7/34).
### Author Declarations
I confirm all relevant ethical guidelines have been followed, and any necessary IRB and/or ethics committee approvals have been obtained.
Yes
The details of the IRB/oversight body that provided approval or exemption for the research described are given below:
This study was approved by the NHS Health Research Authority and Research Ethics Committee (18/LO/0532).
I confirm that all necessary patient/participant consent has been obtained and the appropriate institutional forms have been archived, and that any patient/participant/sample identifiers included were not known to anyone (e.g., hospital staff, patients or participants themselves) outside the research group so cannot be used to identify individuals.
Yes
I understand that all clinical trials and any other prospective interventional studies must be registered with an ICMJE-approved registry, such as ClinicalTrials.gov. I confirm that any such study reported in the manuscript has been registered and the trial registration ID is provided (note: if posting a prospective study registered retrospectively, please provide a statement in the trial ID field explaining why the study was not registered in advance).
Yes
I have followed all appropriate research reporting guidelines and uploaded the relevant EQUATOR Network research reporting checklist(s) and other pertinent material as supplementary files, if applicable.
Yes
Our pre-trained segmentation models are made available online. Moreover, we are in the process of making the MC-RC dataset publicly available.
更多查看译文
关键词
vestibular schwannoma,deep learning,mri,automatic segmentation,multi-centre
AI 理解论文
溯源树
样例
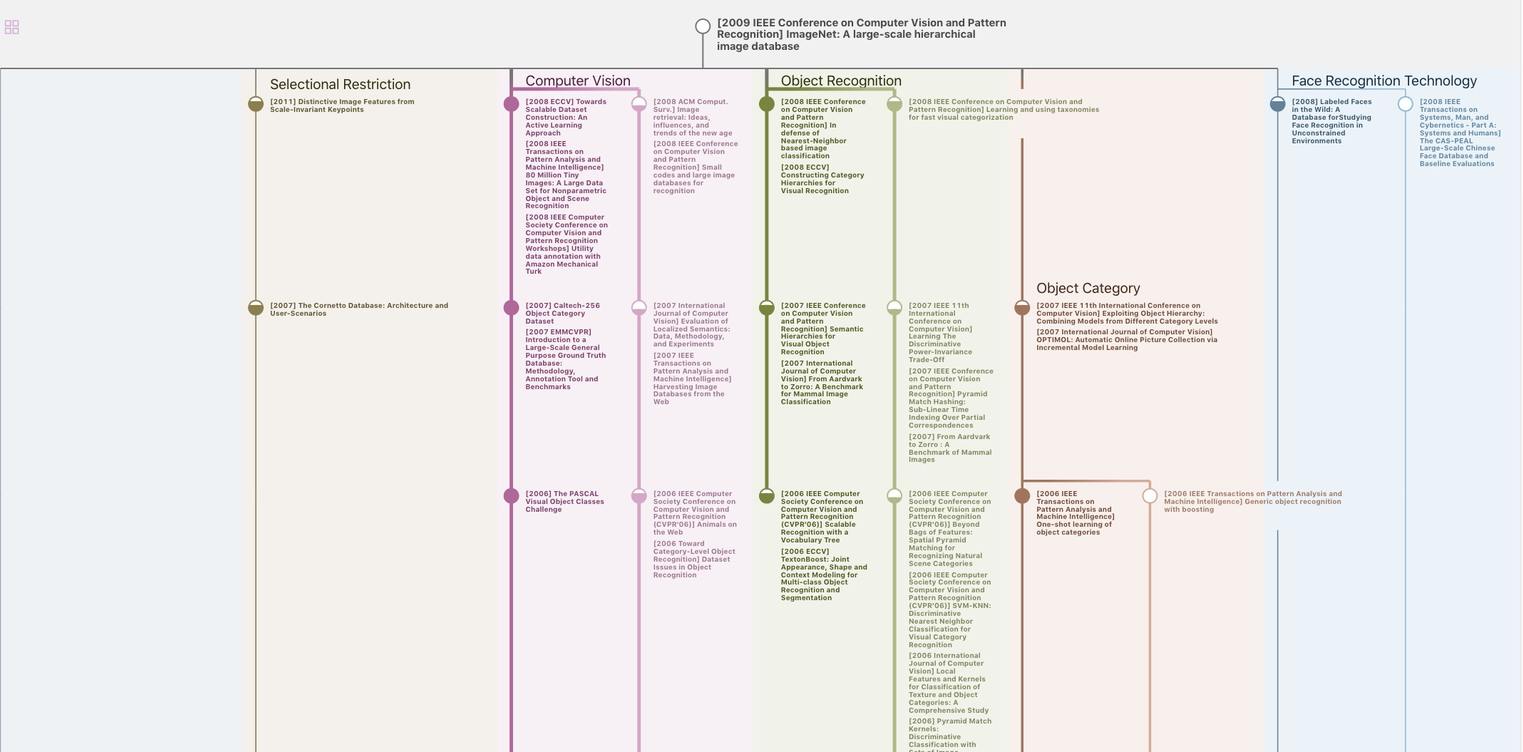
生成溯源树,研究论文发展脉络
Chat Paper
正在生成论文摘要