Improved prediction of blood biomarkers using deep learning
medrxiv(2022)
摘要
Blood and urine biomarkers are an essential part of modern medicine, not only for diagnosis, but also for their direct influence on disease. Many biomarkers have a genetic component, and they have been studied extensively with genome-wide association studies (GWAS) and methods that compute polygenic scores (PGSs). However, these methods generally assume both an additive allelic model and an additive genetic architecture for the target outcome, and thereby risk not capturing non-linear allelic effects nor epistatic interactions. Here, we trained and evaluated deep-learning (DL) models for PGS prediction of 34 blood and urine biomarkers in the UK Biobank cohort, and compared them to linear methods. For lipid traits, the DL models greatly outperformed the linear methods, which we found to be consistent across diverse populations. Furthermore, the DL models captured non-linear effects in covariates, non-additive genotype (allelic) effects, and epistatic interactions between SNPs. Finally, when using only genome-wide significant SNPs from GWAS, the DL models performed equally well or better for all 34 traits tested. Our findings suggest that DL can serve as a valuable addition to existing methods for genotype-phenotype modelling in the era of increasing data availability.
### Competing Interest Statement
S.B. has ownership in Intomics A/S, Hoba Therapeutics Aps, Novo Nordisk A/S, Lundbeck A/S, and managing board memberships in Proscion A/S and Intomics A/S. BJV is a member of the scientific advisory board for Allelica Inc. The other authors declare no competing interests.
### Funding Statement
S.R. and S.B. were supported by the Novo Nordisk Foundation (grants NNF18SA0034956, NNF14CC0001, and NNF17OC0027594). B.J.V. was supported by a Lundbeck Foundation Fellowship (R335-2019-2339) and by the Danish National Research Foundation (Niels Bohr Professorship to Prof. John McGrath), and by the Lundbeck Foundation Initiative for Integrative Psychiatric Research, iPSYCH (R102-A9118, R155-2014-1724 and R248-2017-2003). O.W. was supported by the Novo Nordisk Foundation through the Center for Basic Machine Learning Research in Life Science (grant no. NNF20OC0062606). This research has been conducted using the UK Biobank Resource application 31823. A.I.S., D.W., Computations described in this paper were performed using the National Life Science Supercomputing Center - Computerome at DTU and UCPH, www.computerome.dk.
### Author Declarations
I confirm all relevant ethical guidelines have been followed, and any necessary IRB and/or ethics committee approvals have been obtained.
Yes
The details of the IRB/oversight body that provided approval or exemption for the research described are given below:
The present analyses were conducted under UK Biobank data application number 31823, which received ethical approval from the NHS National Research Ethics Service North West (11/NW/0382).
I confirm that all necessary patient/participant consent has been obtained and the appropriate institutional forms have been archived, and that any patient/participant/sample identifiers included were not known to anyone (e.g., hospital staff, patients or participants themselves) outside the research group so cannot be used to identify individuals.
Yes
I understand that all clinical trials and any other prospective interventional studies must be registered with an ICMJE-approved registry, such as ClinicalTrials.gov. I confirm that any such study reported in the manuscript has been registered and the trial registration ID is provided (note: if posting a prospective study registered retrospectively, please provide a statement in the trial ID field explaining why the study was not registered in advance).
Yes
I have followed all appropriate research reporting guidelines and uploaded the relevant EQUATOR Network research reporting checklist(s) and other pertinent material as supplementary files, if applicable.
Yes
Scientists can obtain access to individual-level data from the UK Biobank by applying to UK Biobank (https://www.ukbiobank.ac.uk/enable-your-research).
更多查看译文
关键词
blood biomarkers,deep learning,improved prediction
AI 理解论文
溯源树
样例
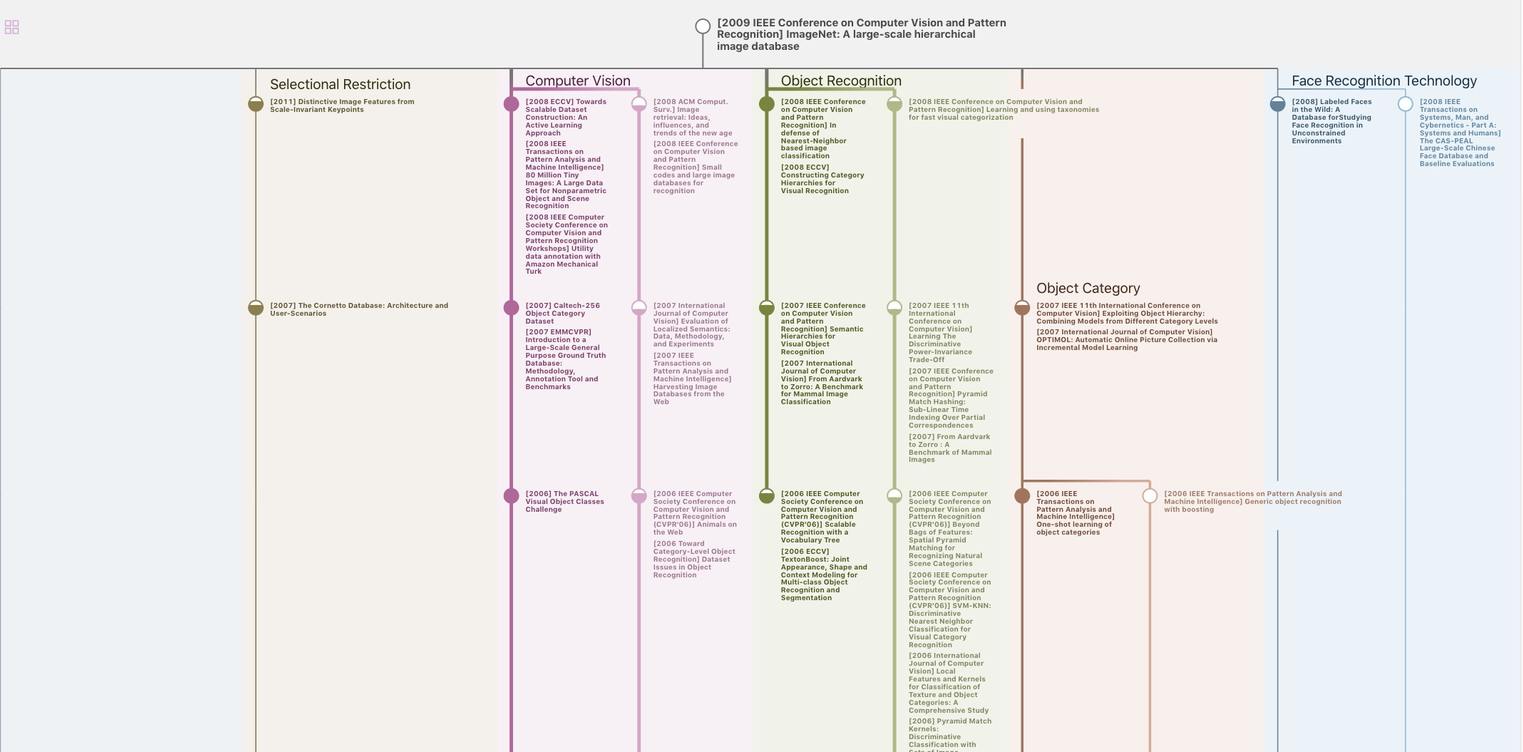
生成溯源树,研究论文发展脉络
Chat Paper
正在生成论文摘要