Development and evaluation of an open-source, standards-based approach to explainable artificial intelligence for managing co-morbidity and clinical guidelines using argumentation techniques and the Transition-based Medical Recommendation model
medRxiv (Cold Spring Harbor Laboratory)(2022)
摘要
Objective Clinical Decision Support (CDS) systems (CDSSs) that integrate clinical guidelines need to reflect real-world co-morbidity. In patient-specific clinical contexts, transparent recommendations that allow for contraindications and other conflicts arising from co-morbidity are a requirement. We aimed to develop and evaluate a non-proprietary, standards-based approach to the deployment of computable guidelines with explainable argumentation, integrated with a commercial Electronic Health Record (EHR) system in a middle-income country.
Materials and Methods We used an ontological framework, the Transition-based Medical Recommendation (TMR) model, to represent, and reason about, guideline concepts, and chose the 2017 International Global Initiative for Chronic Obstructive Lung Disease (GOLD) guideline and a Serbian hospital as the deployment and evaluation site, respectively. To mitigate potential guideline conflicts, we used a TMR-based implementation of the Assumptions-Based Argumentation framework extended with preferences and Goals (ABA+G). Remote EHR integration of computable guidelines was via a microservice architecture based on HL7 FHIR and CDS Hooks. A prototype integration was developed to manage COPD with comorbid cardiovascular or chronic kidney diseases, and a mixed-methods evaluation was conducted with 20 simulated cases and five pulmonologists.
Results Pulmonologists agreed 97% of the time with the GOLD-based COPD symptom severity assessment assigned to each patient by the CDSS, and 98% of the time with one of the proposed COPD care plans. Comments were favourable on the principles of explainable argumentation; inclusion of additional co-morbidities were suggested in the future along with customisation of the level of explanation with expertise.
Conclusion An ontological model provided a flexible means of providing argumentation and explainable artificial intelligence for a long-term condition. Extension to other guidelines and multiple co-morbidities is needed to test the approach further.
Funding The project was funded by the British government through the Engineering and Physical Sciences Research Council (EPSRC) – Global Challenges Research Fund.[1][1]
### Competing Interest Statement
The authors have declared no competing interest.
### Funding Statement
The study was funded by the Engineering and Physical Sciences Research Council Global Challenges Research Fund, the UK Research and Innovation, and Health Data Research UK. The authors gratefully acknowledge infrastructure support from the National Institute for Health and Care Research (NIHR) Imperial Patient Safety Translational Research Centre, the NIHR Imperial Biomedical Research Centre.
### Author Declarations
I confirm all relevant ethical guidelines have been followed, and any necessary IRB and/or ethics committee approvals have been obtained.
Yes
I confirm that all necessary patient/participant consent has been obtained and the appropriate institutional forms have been archived, and that any patient/participant/sample identifiers included were not known to anyone (e.g., hospital staff, patients or participants themselves) outside the research group so cannot be used to identify individuals.
Yes
I understand that all clinical trials and any other prospective interventional studies must be registered with an ICMJE-approved registry, such as ClinicalTrials.gov. I confirm that any such study reported in the manuscript has been registered and the trial registration ID is provided (note: if posting a prospective study registered retrospectively, please provide a statement in the trial ID field explaining why the study was not registered in advance).
Yes
I have followed all appropriate research reporting guidelines and uploaded the relevant EQUATOR Network research reporting checklist(s) and other pertinent material as supplementary files, if applicable.
Yes
All data produced are available online at
[https:github/susoDominguez/COPD-CDS.git][2]
[1]: #ref-1
[2]: https://github/susoDominguez/COPD-CDS.git
更多查看译文
关键词
medical recommendation model,explainable artificial intelligence,clinical guidelines,argumentation techniques,open-source,standards-based,co-morbidity,transition-based
AI 理解论文
溯源树
样例
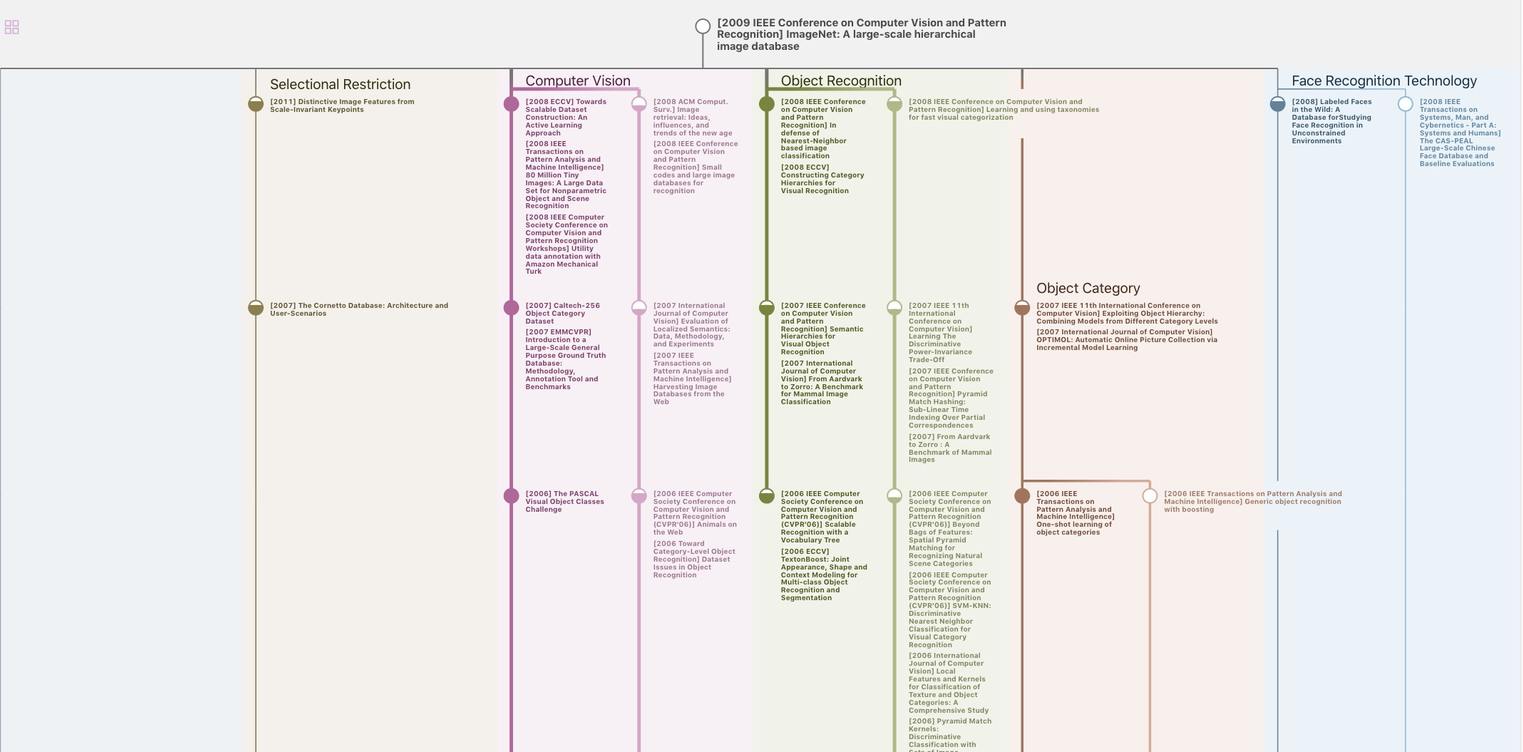
生成溯源树,研究论文发展脉络
Chat Paper
正在生成论文摘要