Building Large-Scale Registries from Unstructured Clinical Notes using a Low-Resource Natural Language Processing Pipeline
medrxiv(2022)
摘要
Building clinical registries is an important step in improving the quality and safety of patient care. With the growing size of medical records, manual abstraction becomes more and more infeasible and impractical. On the other hand, Natural Language Processing Techniques have shown promising results in extracting valuable information from unstructured clinical notes. However, the structure and nature of clinical notes are very different from regular text that state-of-the-art NLP models are trained and tested on and they have their own set of challenges. In this study, we propose SE-K, an efficient and interpretable classification approach for extracting information from clinical notes, and show that it outperforms current state-of-the-art models in text classification. We use this approach to generate a 20-year comprehensive registry of anterior cruciate ligament reconstruction operations, one of the most common orthopedics operations among children and young adults. This registry can help us better understand the outcomes of this surgery and identify potential areas for improvement which can ultimately lead to better treatment outcomes.
### Competing Interest Statement
The authors have declared no competing interest.
### Funding Statement
The study was funded by the Children's Orthopaedic Surgery Foundation (AMK) and Boston Children's Hospital Research Faculty Council (AMK). We also received hardware support from the NVIDIA Basic Research Accelerator Program (AMK)
### Author Declarations
I confirm all relevant ethical guidelines have been followed, and any necessary IRB and/or ethics committee approvals have been obtained.
Yes
The details of the IRB/oversight body that provided approval or exemption for the research described are given below:
IRB of Boston Children Hospital gave ethical approval for this work
I confirm that all necessary patient/participant consent has been obtained and the appropriate institutional forms have been archived, and that any patient/participant/sample identifiers included were not known to anyone (e.g., hospital staff, patients or participants themselves) outside the research group so cannot be used to identify individuals.
Yes
I understand that all clinical trials and any other prospective interventional studies must be registered with an ICMJE-approved registry, such as ClinicalTrials.gov. I confirm that any such study reported in the manuscript has been registered and the trial registration ID is provided (note: if posting a prospective study registered retrospectively, please provide a statement in the trial ID field explaining why the study was not registered in advance).
Yes
I have followed all appropriate research reporting guidelines and uploaded the relevant EQUATOR Network research reporting checklist(s) and other pertinent material as supplementary files, if applicable.
Yes
The data used in this study cannot be publicly shared due to privacy concerns. Data can be access upon reasonable request pending approvals from Boston Children's Hospital.
更多查看译文
关键词
unstructured clinical notes,natural language processing,large-scale,low-resource
AI 理解论文
溯源树
样例
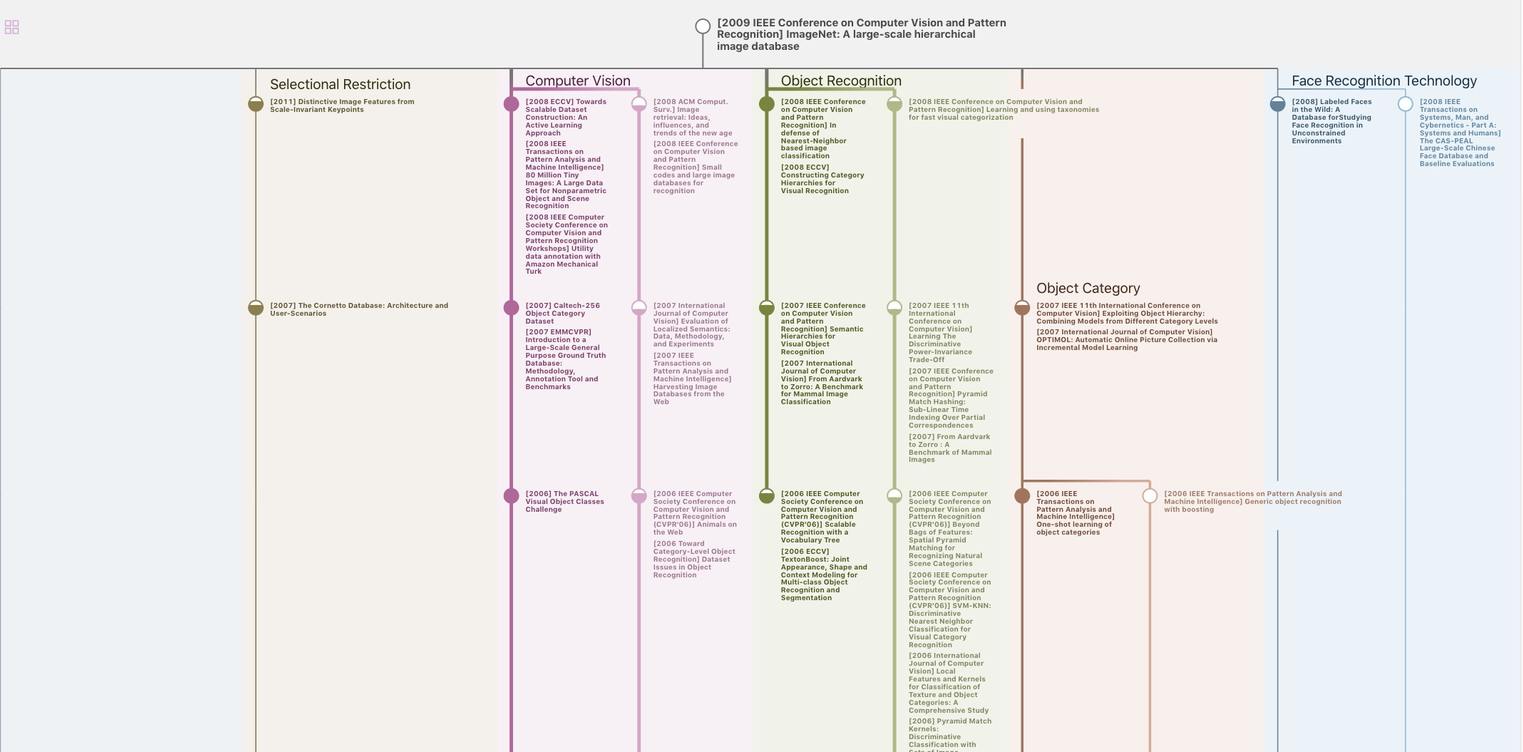
生成溯源树,研究论文发展脉络
Chat Paper
正在生成论文摘要