Predicting COVID-19 case status from self-reported symptoms and behaviors using data from a massive online survey
medRxiv (Cold Spring Harbor Laboratory)(2023)
摘要
With the varying availability of RT-PCR testing for COVID-19 across time and location, there is a need for alternative methods of predicting COVID-19 case status. In this study, multiple machine learning (ML) models were trained and assessed for their ability to accurately predict the COVID-19 case status using US COVID-19 Trends and Impact Survey (CTIS) data. The CTIS includes information on testing, symptoms, demographics, behaviors, and vaccination status. The best performing model was XGBoost, which achieved an F1 score of ≈ 94% in predicting whether an individual was COVID-19 positive or negative. This is a notable improvement on existing models for predicting COVID-19 case status and demonstrates the potential for ML methods to provide policy-relevant estimates.
### Competing Interest Statement
The authors have declared no competing interest.
### Funding Statement
This material is based upon work supported by Facebook (unrestricted gift) and a cooperative agreement from the Centers for Disease Control and Prevention (U01IP001121). Any opinions, findings and conclusions or recommendations expressed in this material are those of the authors and do not necessarily reflect the views of Facebook or the Centers for Disease Control and Prevention. Role of the Funder: Facebook was involved in the design and conduct of the study. The CDC provided funding only. Neither Facebook nor the Centers for Disease Control and Prevention had a role in the collection, management, analysis, and interpretation of the data; preparation, review, or approval of the manuscript; or decision to submit the manuscript for publication.
### Author Declarations
I confirm all relevant ethical guidelines have been followed, and any necessary IRB and/or ethics committee approvals have been obtained.
Yes
The details of the IRB/oversight body that provided approval or exemption for the research described are given below:
The COVID-19 Trends & Impact Survey was approved by the Carnegie Mellon University (CMU) Institutional Review Board, under protocol STUDY2020 00000162. All respondents gave informed consent before participating in the survey. The data acquisition design safeguards respondent privacy by ensuring that researchers at CMU do not receive an identifying information about respondents and Meta (formerly Facebook) does not see survey microdata.
I confirm that all necessary patient/participant consent has been obtained and the appropriate institutional forms have been archived, and that any patient/participant/sample identifiers included were not known to anyone (e.g., hospital staff, patients or participants themselves) outside the research group so cannot be used to identify individuals.
Yes
I understand that all clinical trials and any other prospective interventional studies must be registered with an ICMJE-approved registry, such as ClinicalTrials.gov. I confirm that any such study reported in the manuscript has been registered and the trial registration ID is provided (note: if posting a prospective study registered retrospectively, please provide a statement in the trial ID field explaining why the study was not registered in advance).
Yes
I have followed all appropriate research reporting guidelines and uploaded the relevant EQUATOR Network research reporting checklist(s) and other pertinent material as supplementary files, if applicable.
Yes
Survey microdata are not publicly available because survey participants only consented to public disclosure of aggregate data, and because the legal agreement with Facebook governing operation of the survey prohibits disclosure of microdata without confidentiality protections for respondents. Deidentified microdata are available to researchers under a Data Use Agreement that protects the confidentiality of respondents. Access can be requested online (). Requests are reviewed by the Carnegie Mellon University Office of Sponsored Programs and Facebook Data for Good. County and state-level aggregates of key variables are publicly available in the COVIDcast API, and are presented in an interactive online dashboard ().
更多查看译文
关键词
massive online survey,symptoms,data,self-reported
AI 理解论文
溯源树
样例
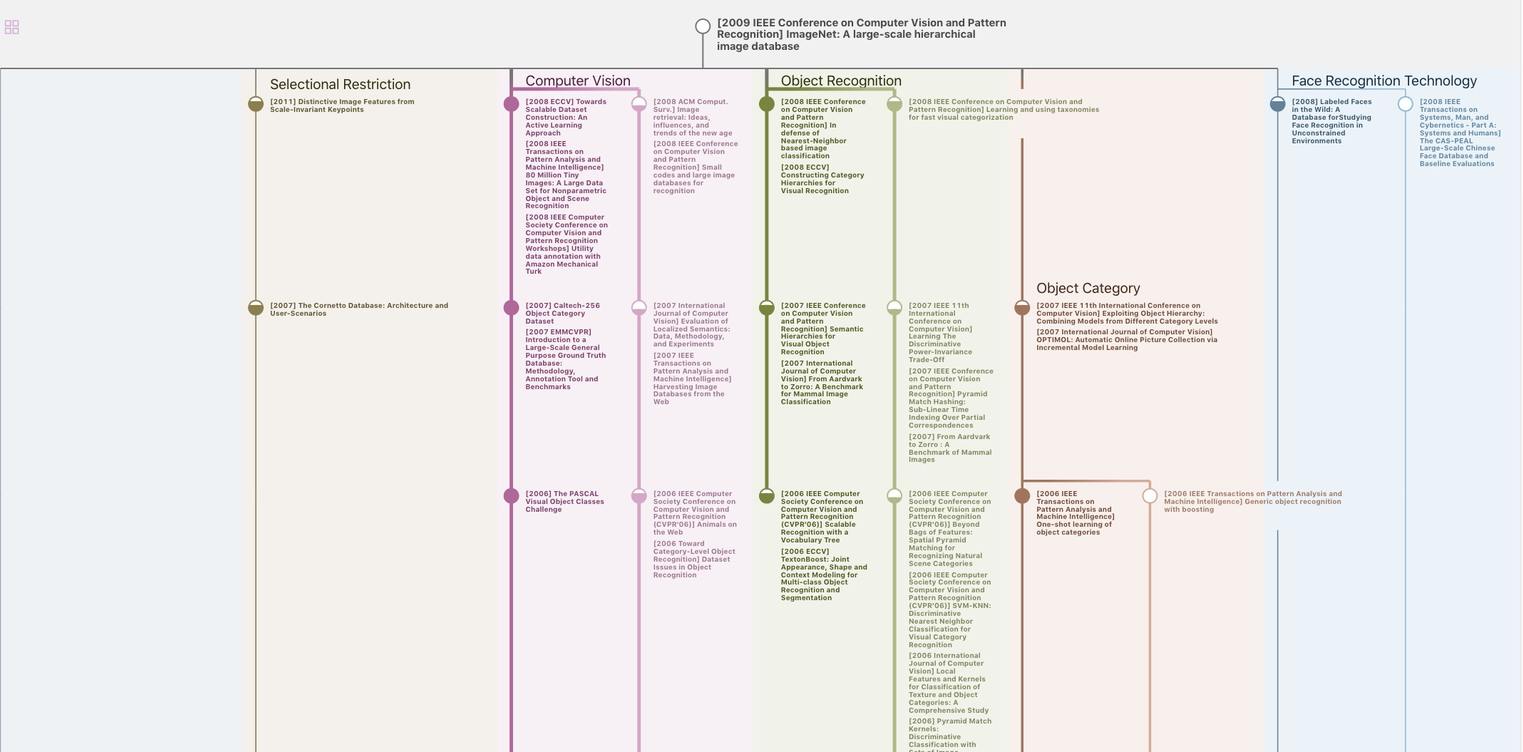
生成溯源树,研究论文发展脉络
Chat Paper
正在生成论文摘要