ChatGPT- versus human-generated answers to frequently asked questions about diabetes: a Turing test-inspired survey among employees of a Danish diabetes center
PloS one(2023)
摘要
Background Large language models have received enormous attention recently with some studies demonstrating their potential clinical value, despite not being trained specifically for this domain. We aimed to investigate whether ChatGPT, a language model optimized for dialogue, can answer frequently asked questions about diabetes.
Methods We conducted a closed e-survey among employees of a large Danish diabetes center. The study design was inspired by the Turing test and non-inferiority trials. Our survey included ten questions with two answers each. One of these was written by a human expert, while the other was generated by ChatGPT. Participants had the task to identify the ChatGPT-generated answer. Data was analyzed at the question-level using logistic regression with robust variance estimation with clustering at participant level. In secondary analyses, we investigated the effect of participant characteristics on the outcome. A 55% non-inferiority margin was pre-defined based on precision simulations and had been published as part of the study protocol before data collection began.
Findings Among 311 invited individuals, 183 participated in the survey (59% response rate). 64% had heard of ChatGPT before, and 19% had tried it. Overall, participants could identify ChatGPT-generated answers 59.5% (95% CI: 57.0, 62.0) of the time. Among participant characteristics, previous ChatGPT use had the strongest association with the outcome (odds ratio: 1.52 (1.16, 2.00), p=0.003). Previous users answered 67.4% (61.7, 72.7) of the questions correctly, versus non-users’ 57.6% (54.9, 60.3).
Interpretation Participants could distinguish between ChatGPT-generated and human-written answers somewhat better than flipping a fair coin. However, our results suggest a stronger predictive value of linguistic features rather than the actual content. Rigorously planned studies are needed to elucidate the risks and benefits of integrating such technologies in routine clinical practice.
Evidence before this study ChatGPT (OpenAI, San Francisco, CA) was released on 30th of November, 2022. A PubMed search for ‘ChatGPT’ conducted on 5th of February, 2023, returned 21 results. All of these were either editorials, commentaries or investigated educational perspectives of the technology. We also searched medRxiv, which returned seven preprints on the topic. Two studies investigated ChatGPT ‘s performance on the United States Medical Licensing Exam and reported that it passed some components of the exam. Other studies investigated ChatGPT ‘s ability to answer questions in specific medical specialties, including ophthalmology, genetics, musculoskeletal disorders, with encouraging results, but often expressing the need for further specialization. We identified one study where participants had to distinguish between chatbot- and human-generated answers to patient-healthcare provider interactions extracted from electronic health records. Chatbot-generated responses were identified 65% of the time, suggesting that they were weakly distinguishable from human-generated answers.
Added value of this study Our study is among the first ones to assess the capabilities of ChatGPT from the patients’ perspective instead of focusing on retrieval of scientific knowledge. We did so in a rigorously designed study inspired by the Turing test and non-inferiority trials. Among all participants, 64% had heard of ChatGPT before, and 19% had tried it. These proportions were even higher among men (87% and 48%). Overall, participants could identify ChatGPT-generated answers (versus human) 60% of the time. We found that individuals who had previously used ChatGPT could distinguish ChatGPT-generated answers from human answers more often, while having contact with patients was not as strong a discriminator. This may suggest a stronger predictive value of linguistic features rather than the actual content.
Implications of all available evidence After ChatGPT, a general-purpose large language model optimized for dialogue, demonstrated its capabilities to the general public, an enormous interest arose in how large language models can support medical research and clinical tasks. Despite not being specifically trained for this, ChatGPT not only has clinical knowledge, but also encodes information about disease management and practical aspects relevant to patients’ everyday lives. Large language models optimized for healthcare use are warranted, but rigorously planned studies are needed to elucidate the risks and benefits of integrating such technologies in patient care.
### Competing Interest Statement
The authors have declared no competing interest.
### Clinical Protocols
### Funding Statement
AH, OLD, JFR, KN, HS, and TKH are employed at Steno Diabetes Center Aarhus that is partly funded by a donation from the Novo Nordisk Foundation. AH is supported by a Data Science Emerging Investigator grant (no. NNF22OC0076725) by the Novo Nordisk Foundation. The funders had no role in the design of the study.
### Author Declarations
I confirm all relevant ethical guidelines have been followed, and any necessary IRB and/or ethics committee approvals have been obtained.
Yes
The details of the IRB/oversight body that provided approval or exemption for the research described are given below:
Ethical approval was not necessary according to Danish legislation as the study only included survey-based data collection. The study was registered in the database of research projects in the Central Denmark Region (no. 1-16-02-35-23).
I confirm that all necessary patient/participant consent has been obtained and the appropriate institutional forms have been archived, and that any patient/participant/sample identifiers included were not known to anyone (e.g., hospital staff, patients or participants themselves) outside the research group so cannot be used to identify individuals.
Yes
I understand that all clinical trials and any other prospective interventional studies must be registered with an ICMJE-approved registry, such as ClinicalTrials.gov. I confirm that any such study reported in the manuscript has been registered and the trial registration ID is provided (note: if posting a prospective study registered retrospectively, please provide a statement in the trial ID field explaining why the study was not registered in advance).
Yes
I have followed all appropriate research reporting guidelines and uploaded the relevant EQUATOR Network research reporting checklist(s) and other pertinent material as supplementary files, if applicable.
Yes
Disclosure of the dataset would comprise the privacy of study participants. The code for processing data and generating the results is shared as supplementary matierial. The study protocol has been published previously.
更多查看译文
关键词
diabetes,asked questions,survey,human-generated,test-inspired
AI 理解论文
溯源树
样例
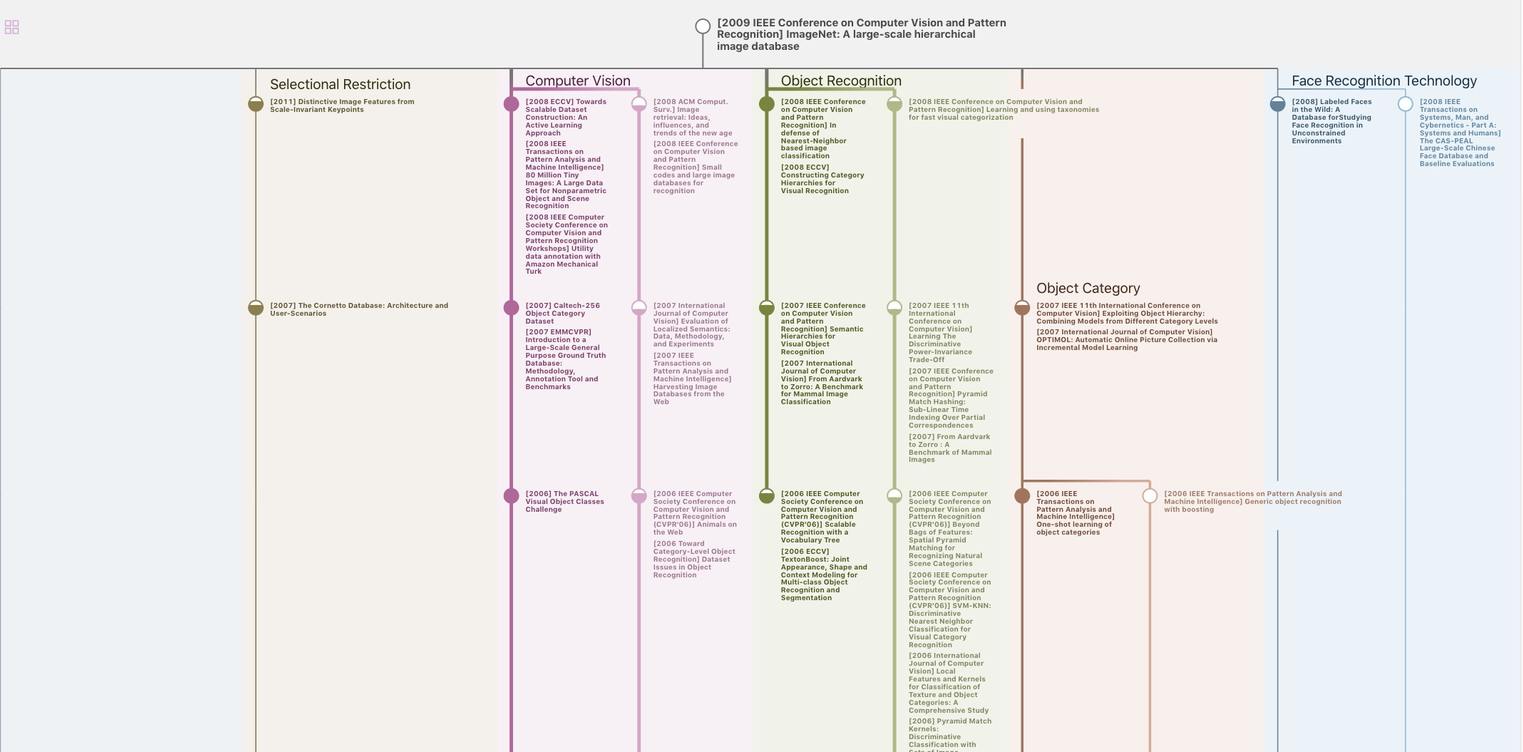
生成溯源树,研究论文发展脉络
Chat Paper
正在生成论文摘要