Predicting Adverse Events in the Cardiothoracic Surgery Intensive Care Unit Using Machine Learning: Results and Challenges
medrxiv(2023)
摘要
It is highly important to anticipate impending problems in patients in the cardiothoracic intensive care unit (CTICU) and be proactive with respect to prediction of adverse events, enabling interventions to prevent them. In order to develop models that predict the occurrence of adverse events after cardiac surgery, a dataset of 9,237 patients was constructed of a single center’s Society of Thoracic Surgeons (STS) internal database. 1,383 of those patients had developed at least one of seven defined adverse events for this analysis. For the control set, we randomly picked 1,383 patients from the group who did not develop any adverse event. The ensemble learning algorithm, random forest, was applied and outperformed the best reported logistic regression models for similar task (c-statistic of ∼0.81), by achieving an AUC of 0.86 with a 95% CI of [0.81-0.90], specificity of 0.72, sensitivity of 0.82, PPV of 0.78 and NPV of 0.77. In the future, we plan to run a similar evaluation process on a multicenter dataset, and then use this static prediction model as a context for using time-evolving data to develop algorithms for real-time feedback to care teams. In acute care settings, such as the operating room and intensive care unit, the ability to anticipate potentially fatal complications will be enhanced by using supervised machine learning algorithms.
### Competing Interest Statement
The authors have declared no competing interest.
### Funding Statement
This study did not receive any funding
### Author Declarations
I confirm all relevant ethical guidelines have been followed, and any necessary IRB and/or ethics committee approvals have been obtained.
Yes
The details of the IRB/oversight body that provided approval or exemption for the research described are given below:
The project was submitted to the Maine Medical Center (MMC) Institutional Review Board (IRB), who determined the project to be "nonresearch" in a letter dated September 11, 2021.
I confirm that all necessary patient/participant consent has been obtained and the appropriate institutional forms have been archived, and that any patient/participant/sample identifiers included were not known to anyone (e.g., hospital staff, patients or participants themselves) outside the research group so cannot be used to identify individuals.
Yes
I understand that all clinical trials and any other prospective interventional studies must be registered with an ICMJE-approved registry, such as ClinicalTrials.gov. I confirm that any such study reported in the manuscript has been registered and the trial registration ID is provided (note: if posting a prospective study registered retrospectively, please provide a statement in the trial ID field explaining why the study was not registered in advance).
Yes
I have followed all appropriate research reporting guidelines and uploaded the relevant EQUATOR Network research reporting checklist(s) and other pertinent material as supplementary files, if applicable.
Yes
All data produced in the present study are available upon reasonable request to the authors
* 1. ACE
: angiotensin-converting enzyme
2. ADP
: adenosine diphosphate
3. ARB
: angiotensin-receptor blocker
4. ASCD
: Adult Cardiac Surgery Database
5. AUC
: Area under the curve
6. AVR
: aortic valve replacement
7. CABG
: coronary artery bypass grafting surgery
8. CAD
: coronary artery disease
9. CBA
: Catheterization-based assist device
10. CI
: Confidence interval
11. CVA
: Cerebrovascular accident
12. CVD
: Cardiovascular disease
13. CTICU
: Cardiothoracic Intensive Care Unit
14. DSWI
: Deep sternal wound infection
15. ECMO
: Extracorporeal membrane oxygenation
16. FTR
: Failure to rescue
17. IABP
: Intra-aortic balloon pump
18. ICD
: Implantable cardioverter-defibrillator
19. IRB
: Institutional Review Board
20. LAD
: Left anterior descending artery
21. MMC
: Maine Medical Center
22. NPV
: Negative predictive value
23. NQF
: National Quality Forum
24. PAD
: Peripheral arterial disease
25. PCI
: percutaneous coronary intervention
26. POLOS
: Postoperative length of stay
27. PPV
: Positive predictive value
28. ROC
: Receiver operating curve
29. STS
: Society of Thoracic Surgeons
30. TIA
: Transient ischemic attack
更多查看译文
关键词
machine learning,intensive care,adverse events
AI 理解论文
溯源树
样例
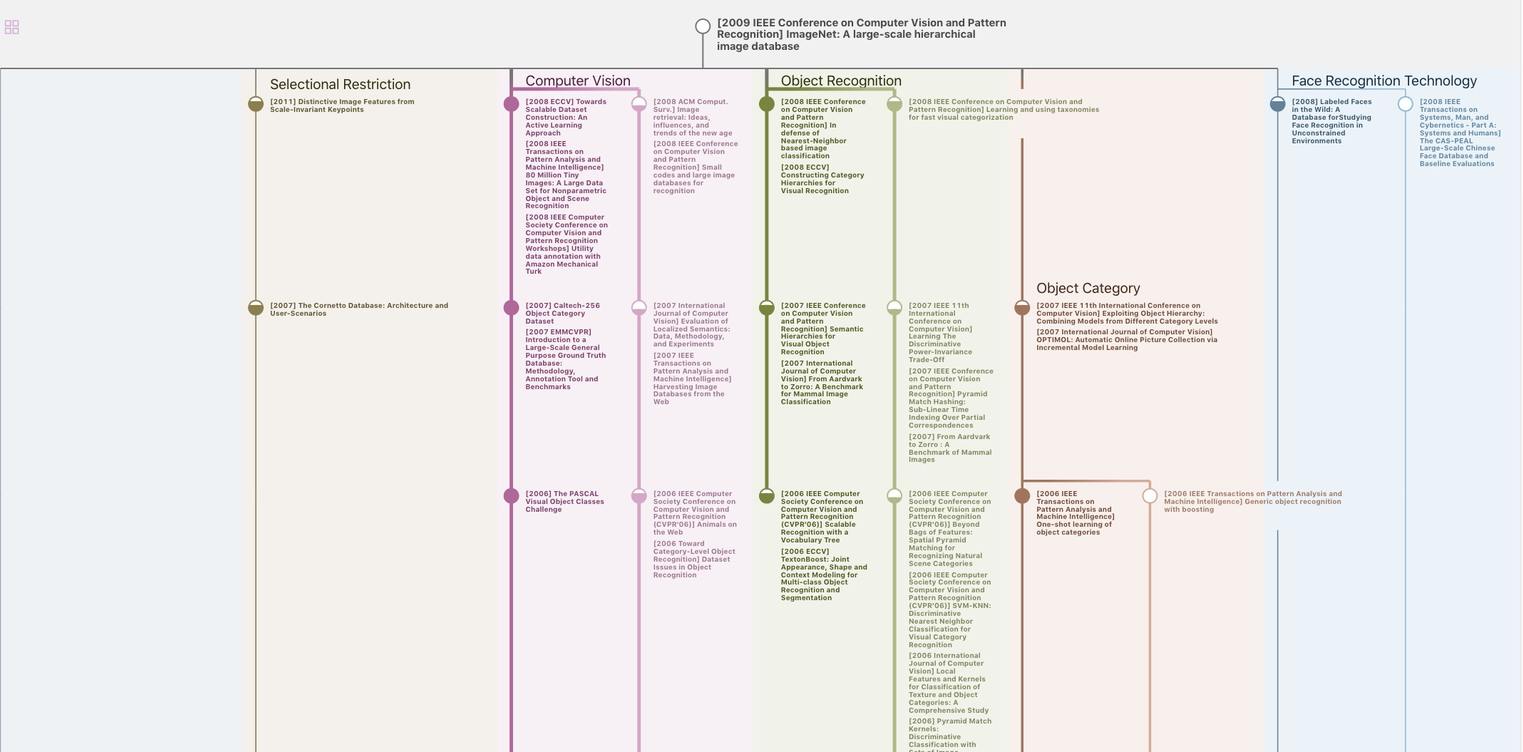
生成溯源树,研究论文发展脉络
Chat Paper
正在生成论文摘要