LiteRev, an Automation Tool to Support Literature Reviews: A Case Study on Acute and Early HIV Infection in Sub-Saharan Africa
medrxiv(2023)
摘要
Background Literature Reviews (LRs) identify, evaluate, and synthesise relevant papers to a particular research question to advance understanding and support decision making. However, LRs, especially traditional systematic reviews are slow, resource intensive, and are outdated quickly.
Objective Using recent Natural Language Processing (NLP) and Unsupervised Machine Learning (UML) methods, this paper presents a tool named LiteRev that supports researchers in conducting LRs.
Methods Based on the user’s query, LiteRev can perform an automated search on different open-access databases and retrieve relevant metadata on the resulting papers. Papers (abstracts or full texts) are text processed and represented as a Term Frequency-Inverse Document Frequency (TF-IDF) matrix. Using dimensionality reduction (PaCMAP) and clustering (HDBSCAN) techniques, the corpus is divided into different topics described by a list of keywords. The user can select one or several topics of interest, enter additional keywords to refine their search, or provide key papers to the research question. Based on these inputs, LiteRev performs an iterative nearest neighbours search, and suggests a list of potentially interesting papers. The user can tag the relevant ones and trigger a new search until no additional paper is suggested for screening. To assess the performance of LiteRev, we ran it in parallel to a manual LR on the burden and care for acute and early HIV infection in sub-Saharan Africa. We assessed the performance of LiteRev using True and False Predictive Values, recall and Work Saved over Sampling.
Results We extracted, text processed and represented into a TF-IDF matrix 631 unique papers from PubMed. The topic modelling module identified 5 main topics and 16 topics (ranging from 13 to 98 papers) and extracted the 10 most important keywords for each. Then, based on 18 key papers, we were able to identify 2 topics of interest with 7 key papers in each of them. Finally, we ran the k-nearest neighbours module and LiteRev suggested first a list of 110 papers for screening, among which 45 papers were confirmed as relevant. From these 45 papers, LiteRev suggested 26 additional papers, out of which 8 were confirmed as relevant. At the end of the iterative process (4 iterations), 193 papers out of 613 papers in total (31.5% of the whole corpus) were suggested by LiteRev. After title/abstract screening, LiteRev identified 64 out of the 87 relevant papers (i.e., recall of 73.6%). After full text screening, LiteRev identified 42 out of the 48 relevant papers (i.e., recall of 87.5%, and Work Saved over Sampling of 56.0%).
Conclusions We presented LiteRev, an automation tool that uses NLP and UML methods to streamline and accelerate LRs and to support researchers in getting quick and in-depth overviews on any topic of interest.
### Competing Interest Statement
The authors have declared no competing interest.
### Funding Statement
We acknowledge the support of the Swiss National Science Foundation (SNF professorship grants 196270 and 202660 to Prof. O. Keiser), which funded this study. The funder had no role in study design, data collection and analysis, decision to publish, or manuscript preparation.
### Author Declarations
I confirm all relevant ethical guidelines have been followed, and any necessary IRB and/or ethics committee approvals have been obtained.
Yes
I confirm that all necessary patient/participant consent has been obtained and the appropriate institutional forms have been archived, and that any patient/participant/sample identifiers included were not known to anyone (e.g., hospital staff, patients or participants themselves) outside the research group so cannot be used to identify individuals.
Yes
I understand that all clinical trials and any other prospective interventional studies must be registered with an ICMJE-approved registry, such as ClinicalTrials.gov. I confirm that any such study reported in the manuscript has been registered and the trial registration ID is provided (note: if posting a prospective study registered retrospectively, please provide a statement in the trial ID field explaining why the study was not registered in advance).
Yes
I have followed all appropriate research reporting guidelines and uploaded the relevant EQUATOR Network research reporting checklist(s) and other pertinent material as supplementary files, if applicable.
Yes
All data produced in the present study are available upon reasonable request to the authors.
* AEHI
: Acute and early hiv infection
API
: Application programming interface
DBCV
: Density-based clustering validation
HDBSCAN
: Hierarchical density-based spatial clustering of applications with noise
HIV
: Human immunodeficiency virus
k-NN
: k-nearest neighbours
LR
: Literature review
NLP
: Natural language processing
PacMAP
: Pairwise controlled manifold approximation
TF-IDF
: Term frequency - Inverse document frequency
UML
: Unsupervised machine learning
WSS
: Work saved over sampling
更多查看译文
关键词
literev,early hiv infection,automation tool,sub-saharan
AI 理解论文
溯源树
样例
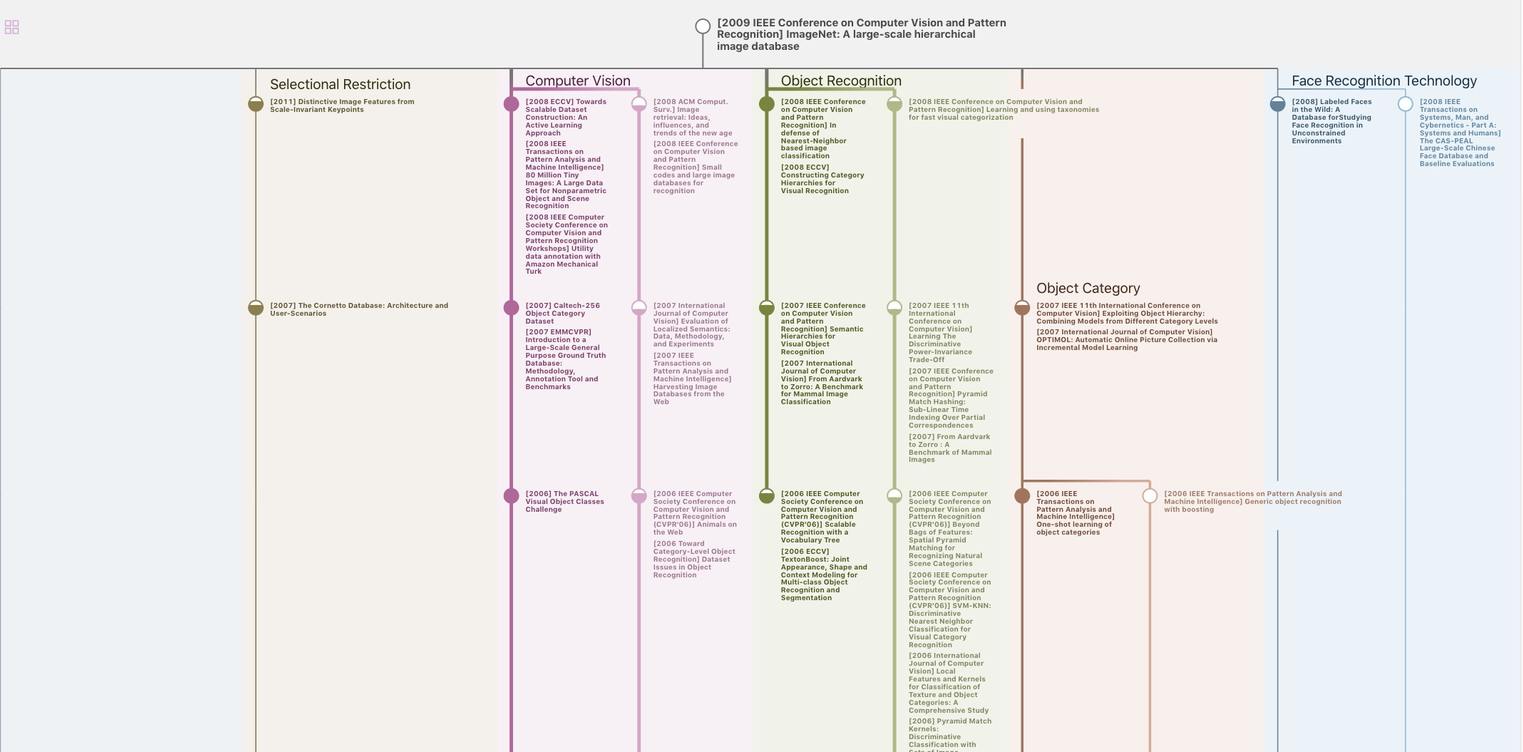
生成溯源树,研究论文发展脉络
Chat Paper
正在生成论文摘要