A methodology of phenotyping ICU patients from EHR data: High-fidelity, personalized, and interpretable phenotypes estimation
JOURNAL OF BIOMEDICAL INFORMATICS(2023)
摘要
Objective: Computing phenotypes that provide high-fidelity, time-dependent characterizations and yield per-sonalized interpretations is challenging, especially given the complexity of physiological and healthcare systems and clinical data quality. This paper develops a methodological pipeline to estimate unmeasured physiological parameters and produce high-fidelity, personalized phenotypes anchored to physiological mechanics from electronic health record (EHR). Methods: A methodological phenotyping pipeline is developed that computes new phenotypes defined with unmeasurable computational biomarkers quantifying specific physiological properties in real time. Working within the inverse problem framework, this pipeline is applied to the glucose-insulin system for ICU patients using data assimilation to estimate an established mathematical physiological model with stochastic optimiza-tion. This produces physiological model parameter vectors of clinically unmeasured endocrine properties, here insulin secretion, clearance, and resistance, estimated for individual patient. These physiological parameter vectors are used as inputs to unsupervised machine learning methods to produce phenotypic labels and discrete physiological phenotypes. These phenotypes are inherently interpretable because they are based on parametric physiological descriptors. To establish potential clinical utility, the computed phenotypes are evaluated with external EHR data for consistency and reliability and with clinician face validation. Results: The phenotype computation was performed on a cohort of 109 ICU patients who received no or short -acting insulin therapy, rendering continuous and discrete physiological phenotypes as specific computational biomarkers of unmeasured insulin secretion, clearance, and resistance on time windows of three days. Six, six, and five discrete phenotypes were found in the first, middle, and last three-day periods of ICU stays, respectively. Computed phenotypic labels were predictive with an average accuracy of 89%. External validation of discrete phenotypes showed coherence and consistency in clinically observable differences based on laboratory measurements and ICD 9/10 codes and clinical concordance from face validity. A particularly clinically impactful parameter, insulin secretion, had a concordance accuracy of 83%+/- 27%. Conclusion: The new physiological phenotypes computed with individual patient ICU data and defined by estimates of mechanistic model parameters have high physiological fidelity, are continuous, time-specific, per-sonalized, interpretable, and predictive. This methodology is generalizable to other clinical and physiological settings and opens the door for discovering deeper physiological information to personalize medical care.
更多查看译文
关键词
Phenotyping,Data assimilation,Physiological modeling,Electronic health record,Knowledge representation with machine,learning,Data mining
AI 理解论文
溯源树
样例
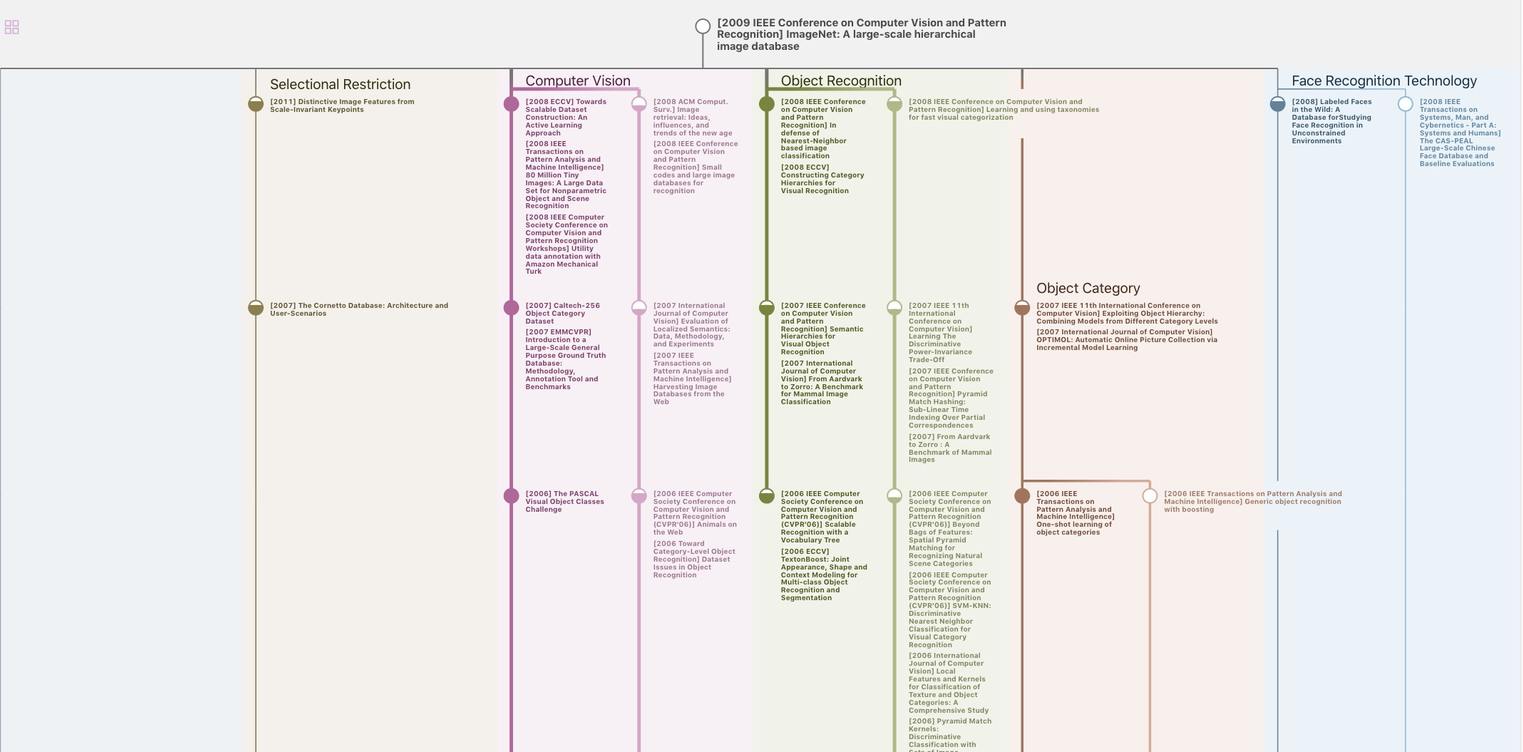
生成溯源树,研究论文发展脉络
Chat Paper
正在生成论文摘要