Optimized ITS/CITS models for intervention evaluation considering the nonlinear impact of covariates
medRxiv (Cold Spring Harbor Laboratory)(2023)
摘要
There is a lack of approaches to evaluate the effectiveness of interventions when there are nonlinear impacts of covariates to the outcome series.
Based on the classic framework of ITS/CITS segmented regression, while considering autocorrelation of time series, we adopted a nonlinear dynamic modeling strategy (Hammerstein) to measure the nonlinear effects of covariates, and proposed four optimized models: ITS-A, CITS-A, ITS-HA, and CITS-HA. To compare the accuracy and precision in estimating the long-term impact of an intervention between the optimized and classic segmented models, we constructed a sequence generator to simulate the outcome series with actual characteristics. The relative error with respect to the true value was the accuracy indicator, and the width of the 95% CI and the truth value coverage rate of the corresponding 95% CI are the precision indicator for model assessments.
The relative error of impact evaluation in the four optimized models was 4.49 percentage points lower than that in the classic models, specifically ITS-A (14.34%) and ITS-HA (21.47%) relative to ITS (26.66%), CITS-A (16.57%), and CITS-HA (17.94%) relative to CITS (21.59%). The width of the 95% CI of point estimate of long-term impacts in the optimized models was 0.1261, which was expanded by 58.71% compared with 0.0875 for the classic model. However, the optimized models covered the true value in all test scenarios, whereas the coverage rates of the classic ITS and CITS models were 73.33% and 83.33%, respectively.
The optimized models are useful tools as they can assess the long-term impact of interventions with additional considerations for the nonlinear effects of covariates and allow for modeling of time-series autocorrelation and lag of intervention effects.
### Competing Interest Statement
The authors have declared no competing interest.
### Funding Statement
This work is supported by the National Natural Science Foundation of China (72074229).
### Author Declarations
I confirm all relevant ethical guidelines have been followed, and any necessary IRB and/or ethics committee approvals have been obtained.
Yes
I confirm that all necessary patient/participant consent has been obtained and the appropriate institutional forms have been archived, and that any patient/participant/sample identifiers included were not known to anyone (e.g., hospital staff, patients or participants themselves) outside the research group so cannot be used to identify individuals.
Yes
I understand that all clinical trials and any other prospective interventional studies must be registered with an ICMJE-approved registry, such as ClinicalTrials.gov. I confirm that any such study reported in the manuscript has been registered and the trial registration ID is provided (note: if posting a prospective study registered retrospectively, please provide a statement in the trial ID field explaining why the study was not registered in advance).
Yes
I have followed all appropriate research reporting guidelines, such as any relevant EQUATOR Network research reporting checklist(s) and other pertinent material, if applicable.
Yes
All data produced in the present study are available upon reasonable request to the authors
更多查看译文
关键词
intervention evaluation,its/cits models,covariates,nonlinear impact
AI 理解论文
溯源树
样例
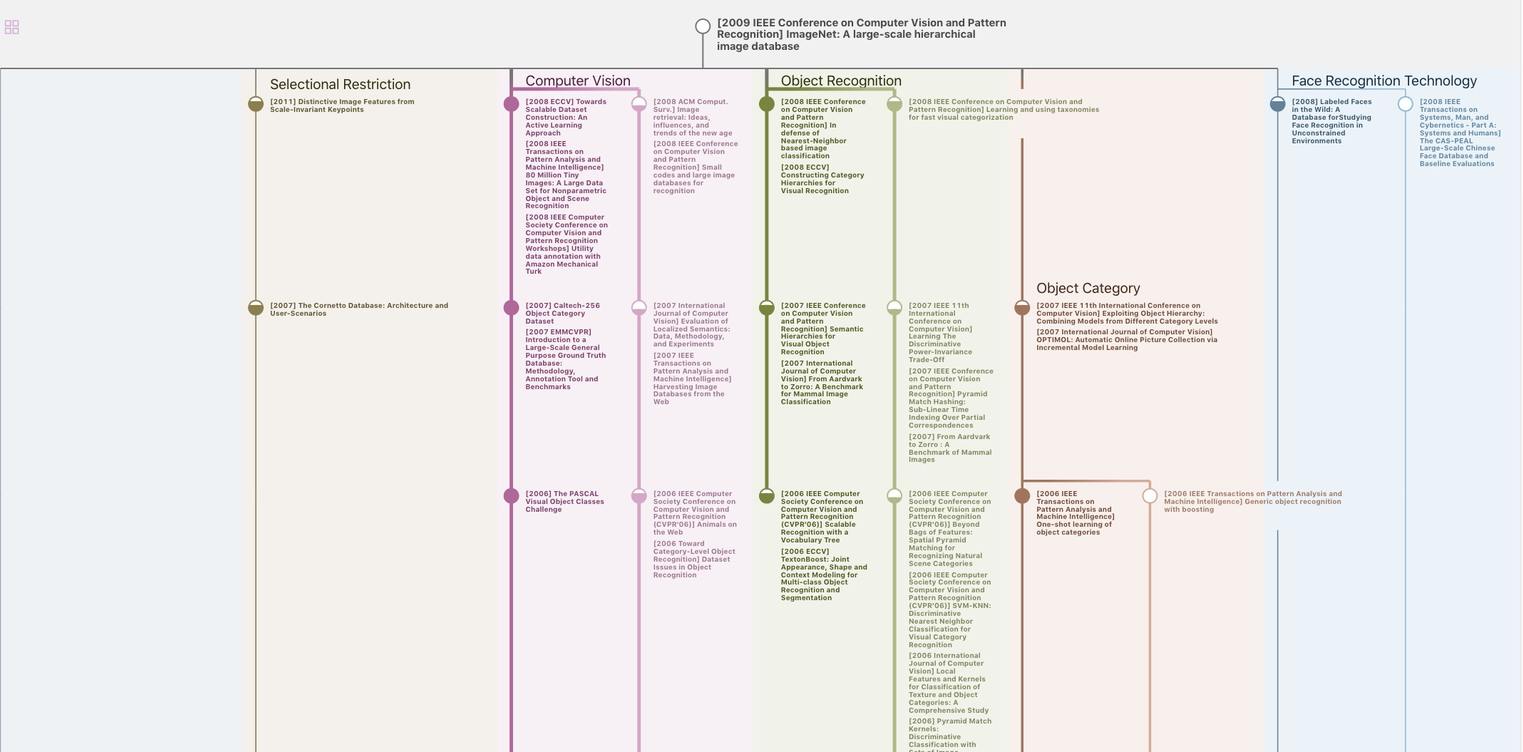
生成溯源树,研究论文发展脉络
Chat Paper
正在生成论文摘要