Selective Sensing of Volatile Organic Compounds Using an Electrostatically Formed Nanowire Sensor Based on Automatic Machine Learning
ACS SENSORS(2023)
摘要
With the development of Internet of Things technology, various sensors are under intense development. Electrostatically formed nanowire (EFN) gas sensors are multigate Si sensors based on CMOS technology and have the unique advantages of ultralow power consumption and very large-scale integration (VLSI) compatibility for mass production. In order to achieve selectivity, machine learning is required to accurately identify the detected gas. In this work, we introduce automatic learning technology, by which the common algorithms are sorted and applied to the EFN gas sensor. The advantages and disadvantages of the top four tree-based model algorithms are discussed, and the unilateral training models are ensembled to further improve the accuracy of the algorithm. The analyses of two groups of experiments show that the CatBoost algorithm has the highest evaluation index. In addition, the feature importance of the classification is analyzed from the physical meaning of electrostatically formed nanowire dimensions, paving the way for model fusion and mechanism exploration.
更多查看译文
关键词
automatic learning,machine learning,electrostatically formed nanowires,selectivity,volatile organic compounds,sensor
AI 理解论文
溯源树
样例
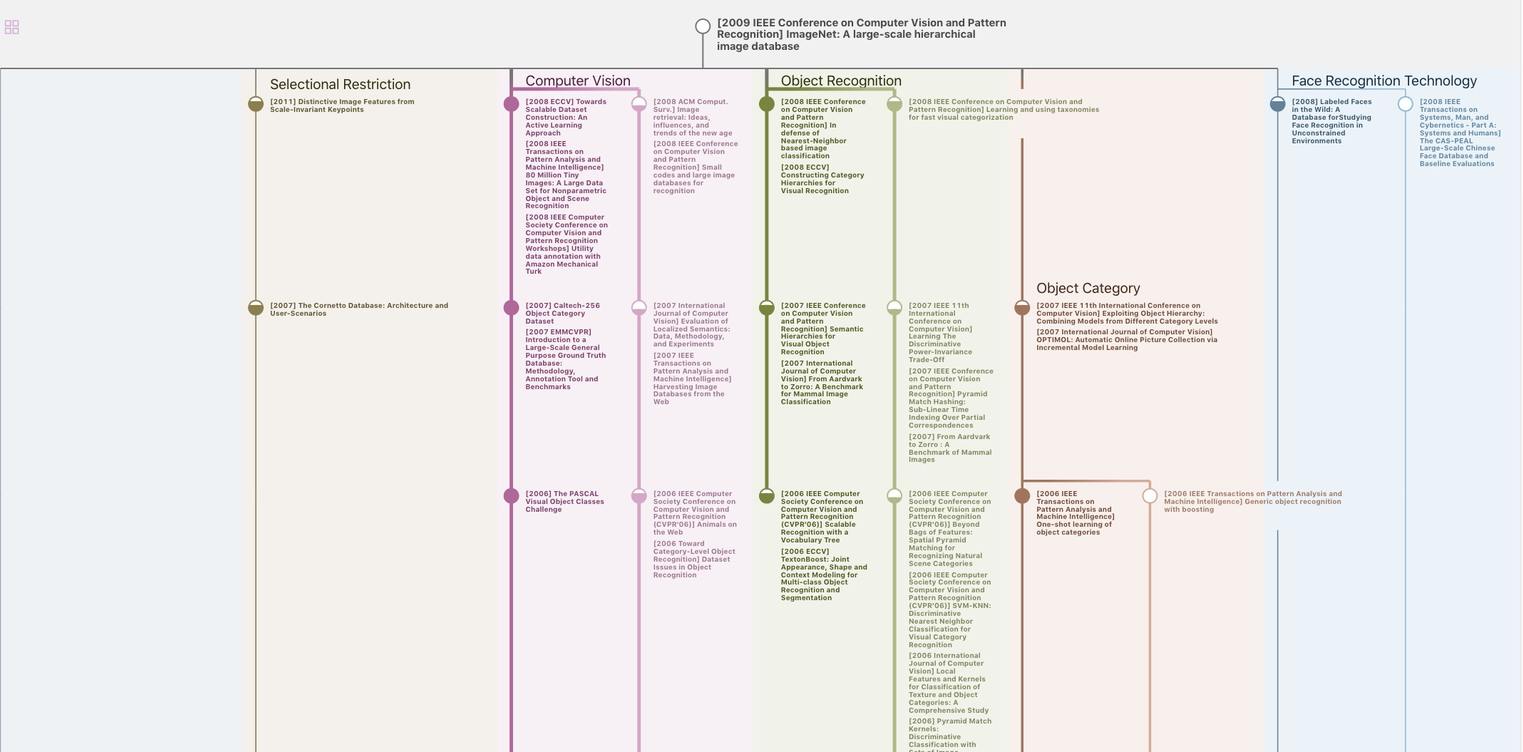
生成溯源树,研究论文发展脉络
Chat Paper
正在生成论文摘要