Cross Refinement Techniques for Markerless Human Motion Capture
ACM Transactions on Multimedia Computing, Communications, and Applications(2020)
摘要
This article presents a global 3D human pose estimation method for markerless motion capture. Given two calibrated images of a person, it first obtains the 2D joint locations in the images using a pre-trained 2D Pose CNN, then constructs the 3D pose based on stereo triangulation. To improve the accuracy and the stability of the system, we propose two efficient optimization techniques for the joints. The first one, called cross-view refinement, optimizes the joints based on epipolar geometry. The second one, called cross-joint refinement, optimizes the joints using bone-length constraints. Our method automatically detects and corrects the unreliable joint, and consequently is robust against heavy occlusion, symmetry ambiguity, motion blur, and highly distorted poses. We evaluate our method on a number of benchmark datasets covering indoors and outdoors, which showed that our method is better than or on par with the state-of-the-art methods. As an application, we create a 3D human pose dataset using the proposed motion capture system, which contains about 480K images of both indoor and outdoor scenes, and demonstrate the usefulness of the dataset for human pose estimation.
更多查看译文
关键词
Human pose estimation,camera calibration,convolutional neural network,epipolar geometry
AI 理解论文
溯源树
样例
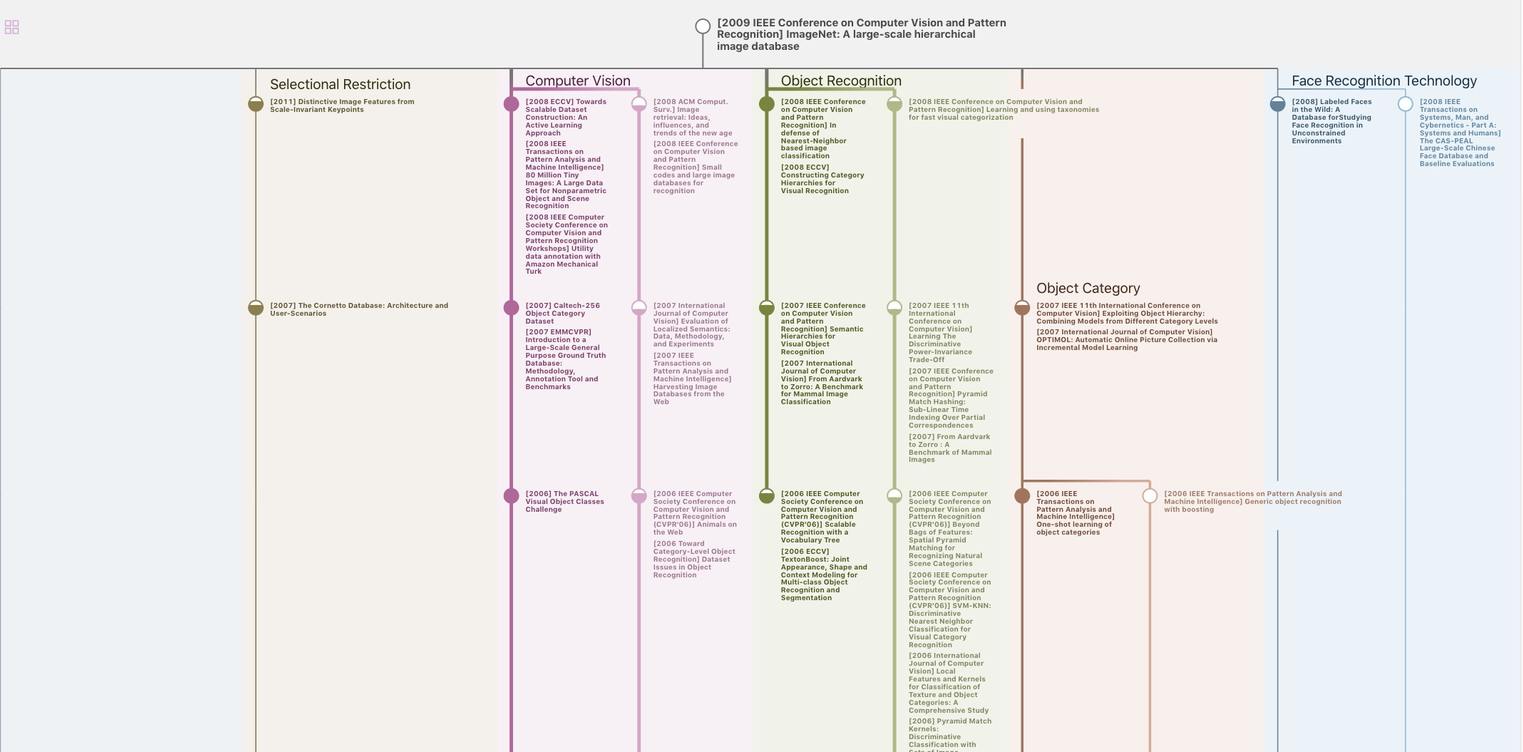
生成溯源树,研究论文发展脉络
Chat Paper
正在生成论文摘要