RECLIP: Resource-efficient CLIP by Training with Small Images
Trans. Mach. Learn. Res.(2023)
Abstract
We present RECLIP (Resource-efficient CLIP), a simple method that minimizes computational resource footprint for CLIP (Contrastive Language Image Pretraining). Inspired by the notion of coarse-to-fine in computer vision, we leverage small images to learn from large-scale language supervision efficiently, and finetune the model with high-resolution data in the end. Since the complexity of the vision transformer heavily depends on input image size, our approach significantly reduces the training resource requirements both in theory and in practice. Using the same batch size and training epoch, RECLIP achieves highly competitive zero-shot classification and image text retrieval accuracy with 6 to 8$\times$ less computational resources and 7 to 9$\times$ fewer FLOPs than the baseline. Compared to the state-of-the-art contrastive learning methods, RECLIP demonstrates 5 to 59$\times$ training resource savings while maintaining highly competitive zero-shot classification and retrieval performance. We hope this work will pave the path for the broader research community to explore language supervised pretraining in more resource-friendly settings.
MoreTranslated text
AI Read Science
Must-Reading Tree
Example
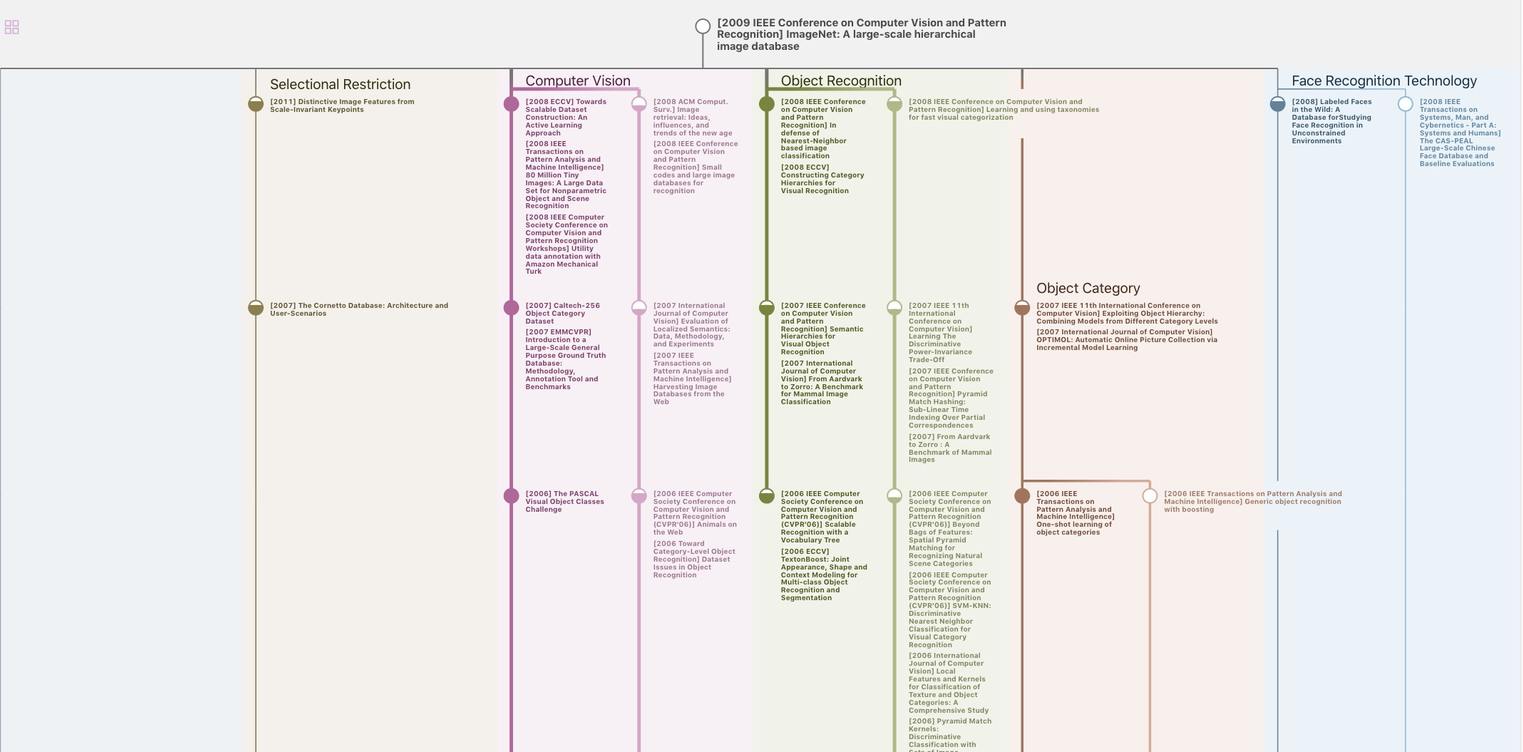
Generate MRT to find the research sequence of this paper
Chat Paper
Summary is being generated by the instructions you defined