Optimizing Sensor Allocation against Attackers with Uncertain Intentions: A Worst-Case Regret Minimization Approach
CoRR(2023)
摘要
This paper is concerned with the optimal allocation of detection resources (sensors) to mitigate multi-stage attacks, in the presence of the defender's uncertainty in the attacker's intention. We model the attack planning problem using a Markov decision process and characterize the uncertainty in the attacker's intention using a finite set of reward functions -- each reward represents a type of the attacker. Based on this modeling framework, we employ the paradigm of the worst-case absolute regret minimization from robust game theory and develop mixed-integer linear program (MILP) formulations for solving the worst-case regret minimizing sensor allocation strategies for two classes of attack-defend interactions: one where the defender and attacker engage in a zero-sum game, and another where they engage in a non-zero-sum game. We demonstrate the effectiveness of our framework using a stochastic gridworld example.
更多查看译文
关键词
sensor allocation,attackers,intentions,worst-case
AI 理解论文
溯源树
样例
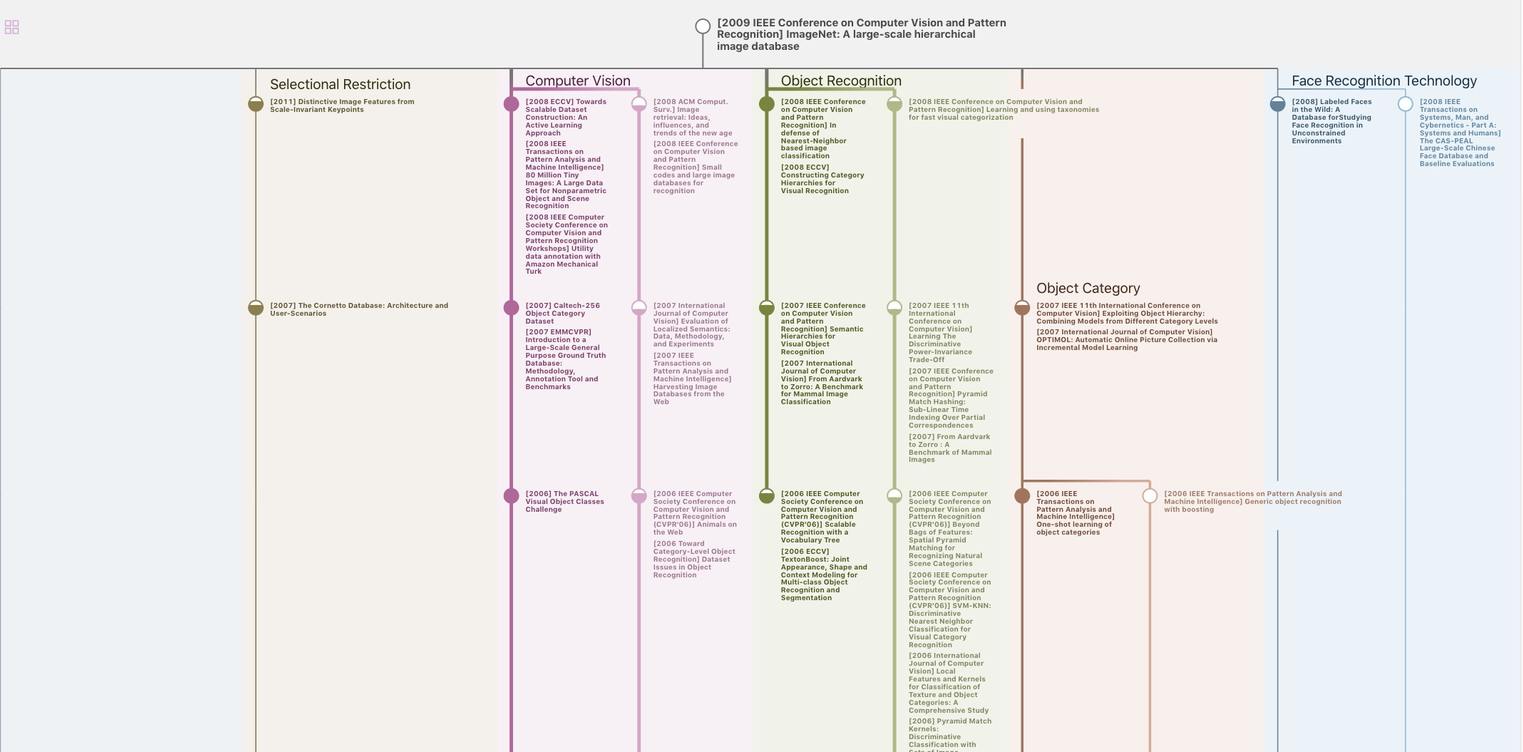
生成溯源树,研究论文发展脉络
Chat Paper
正在生成论文摘要