Echo of Neighbors: Privacy Amplification for Personalized Private Federated Learning with Shuffle Model
CoRR(2023)
摘要
Federated Learning, as a popular paradigm for collaborative training, is vulnerable against privacy attacks. Different privacy levels regarding users' attitudes need to be satisfied locally, while a strict privacy guarantee for the global model is also required centrally. Personalized Local Differential Privacy (PLDP) is suitable for preserving users' varying local privacy, yet only provides a central privacy guarantee equivalent to the worst-case local privacy level. Thus, achieving strong central privacy as well as personalized local privacy with a utility-promising model is a challenging problem. In this work, a general framework (APES) is built up to strengthen model privacy under personalized local privacy by leveraging the privacy amplification effect of the shuffle model. To tighten the privacy bound, we quantify the heterogeneous contributions to the central privacy user by user. The contributions are characterized by the ability of generating "echos" from the perturbation of each user, which is carefully measured by proposed methods Neighbor Divergence and Clip-Laplace Mechanism. Furthermore, we propose a refined framework (S-APES) with the post-sparsification technique to reduce privacy loss in high-dimension scenarios. To the best of our knowledge, the impact of shuffling on personalized local privacy is considered for the first time. We provide a strong privacy amplification effect, and the bound is tighter than the baseline result based on existing methods for uniform local privacy. Experiments demonstrate that our frameworks ensure comparable or higher accuracy for the global model.
更多查看译文
关键词
personalized private federated learning,privacy amplification,shuffle,neighbors
AI 理解论文
溯源树
样例
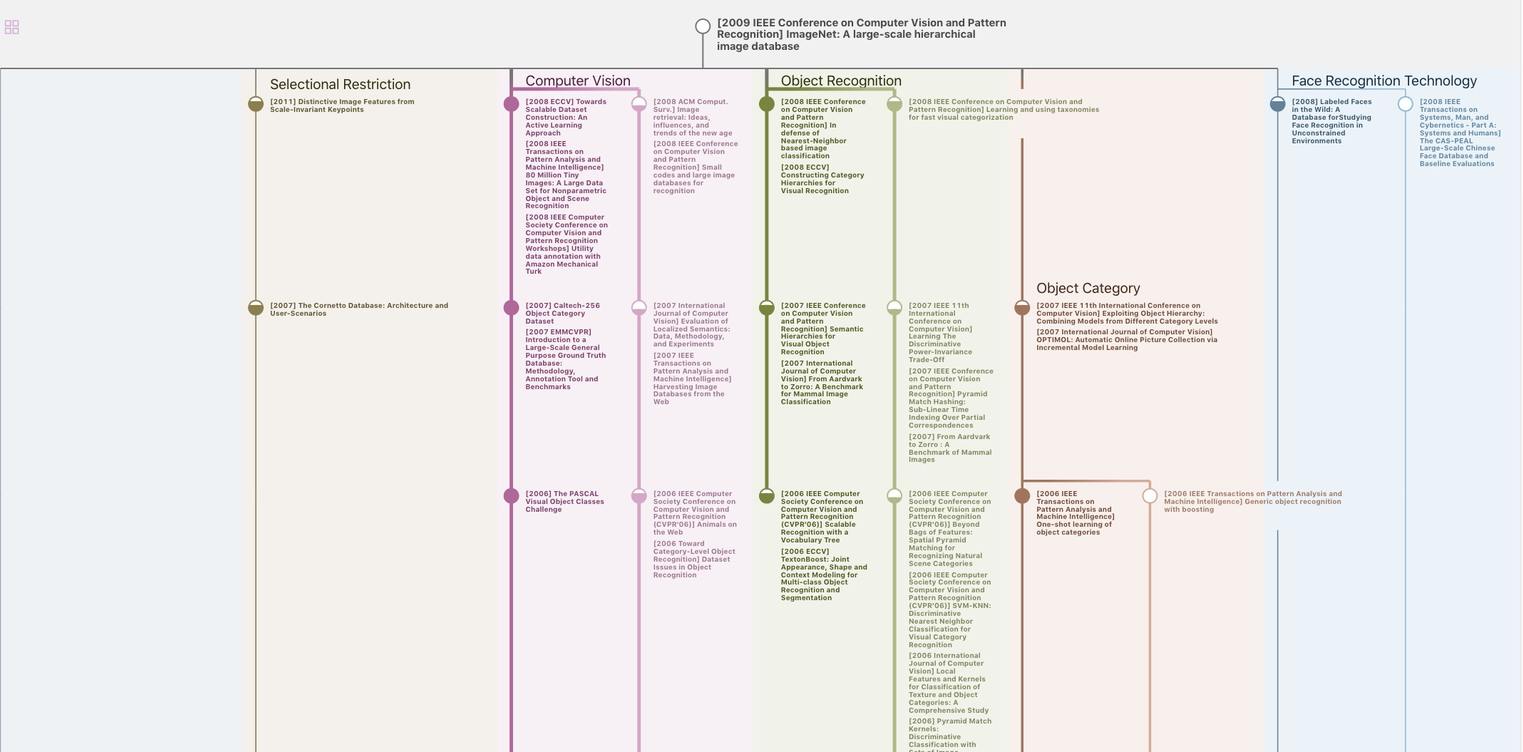
生成溯源树,研究论文发展脉络
Chat Paper
正在生成论文摘要