UnCRtainTS: Uncertainty Quantification for Cloud Removal in Optical Satellite Time Series
CoRR(2023)
摘要
Clouds and haze often occlude optical satellite images, hindering continuous, dense monitoring of the Earth's surface. Although modern deep learning methods can implicitly learn to ignore such occlusions, explicit cloud removal as pre-processing enables manual interpretation and allows training models when only few annotations are available. Cloud removal is challenging due to the wide range of occlusion scenarios -- from scenes partially visible through haze, to completely opaque cloud coverage. Furthermore, integrating reconstructed images in downstream applications would greatly benefit from trustworthy quality assessment. In this paper, we introduce UnCRtainTS, a method for multi-temporal cloud removal combining a novel attention-based architecture, and a formulation for multivariate uncertainty prediction. These two components combined set a new state-of-the-art performance in terms of image reconstruction on two public cloud removal datasets. Additionally, we show how the well-calibrated predicted uncertainties enable a precise control of the reconstruction quality.
更多查看译文
关键词
completely opaque cloud coverage,continuous monitoring,dense monitoring,Earth's surface,explicit cloud removal,haze,image reconstruction,manual interpretation,modern deep learning methods,multitemporal cloud removal,multivariate uncertainty prediction,occlusion scenarios-from scenes,optical satellite images,optical satellite time series,predicted uncertainties,public cloud removal datasets,reconstructed images,uncertainty quantification,UnCRtainTS
AI 理解论文
溯源树
样例
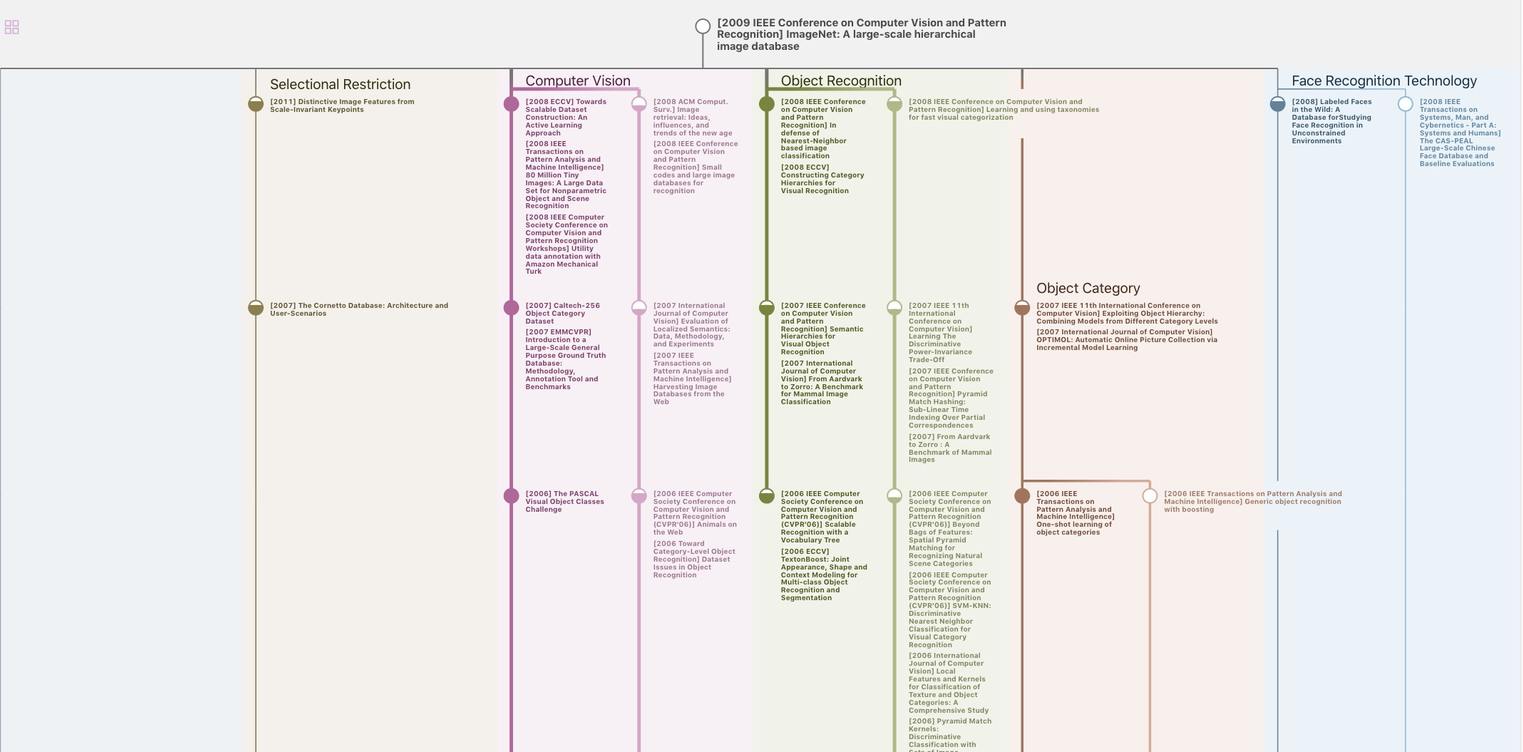
生成溯源树,研究论文发展脉络
Chat Paper
正在生成论文摘要