Upscaling of Latent Heat Flux in Heihe River Basin Based on Transfer Learning Model
REMOTE SENSING(2023)
Abstract
Latent heat flux (LE) plays an essential role in the hydrological cycle, surface energy balance, and climate change, but the spatial resolution of site-scale LE extremely limits its application potential over a regional scale. To overcome the limitation, five transfer learning models were constructed based on artificial neural networks (ANNs), random forests (RFs), extreme gradient boosting (XGBoost), support vector machine (SVM), and light gradient boosting machine (LightGBM) to upscale LE from site scale to regional scale in Heihe River basin (HRB). The instance-transfer approach that utilizes data samples outside of HRB was used in the transfer learning models. Moreover, the Bayesian-based three-cornered hat (BTCH) method was used to fuse the best three upscaling results from ANN, RF, and XGBoost models to improve the accuracy of the results. The results indicated that the transfer learning models perform best when the transfer ratio (the data samples ratio between external and HRB dataset) was 0.6. Specifically, the coefficient of determination (R-2) and root mean squared errors (RMSE) of LE upscaled by ANN model was improved or reduced by 6% or 17% than the model without external data. Furthermore, the BTCH method can effectively improve the performance of single transfer learning model with the highest accuracy (R-2 = 0.83, RMSE = 18.84 W/m(2)). Finally, the LE upscaling model based on transfer learning model demonstrated great potential in HRB, which may be applicable to similar research in other regions.
MoreTranslated text
Key words
artificial neural networks,random forests,extreme gradient boosting,transfer learning,product ensemble
AI Read Science
Must-Reading Tree
Example
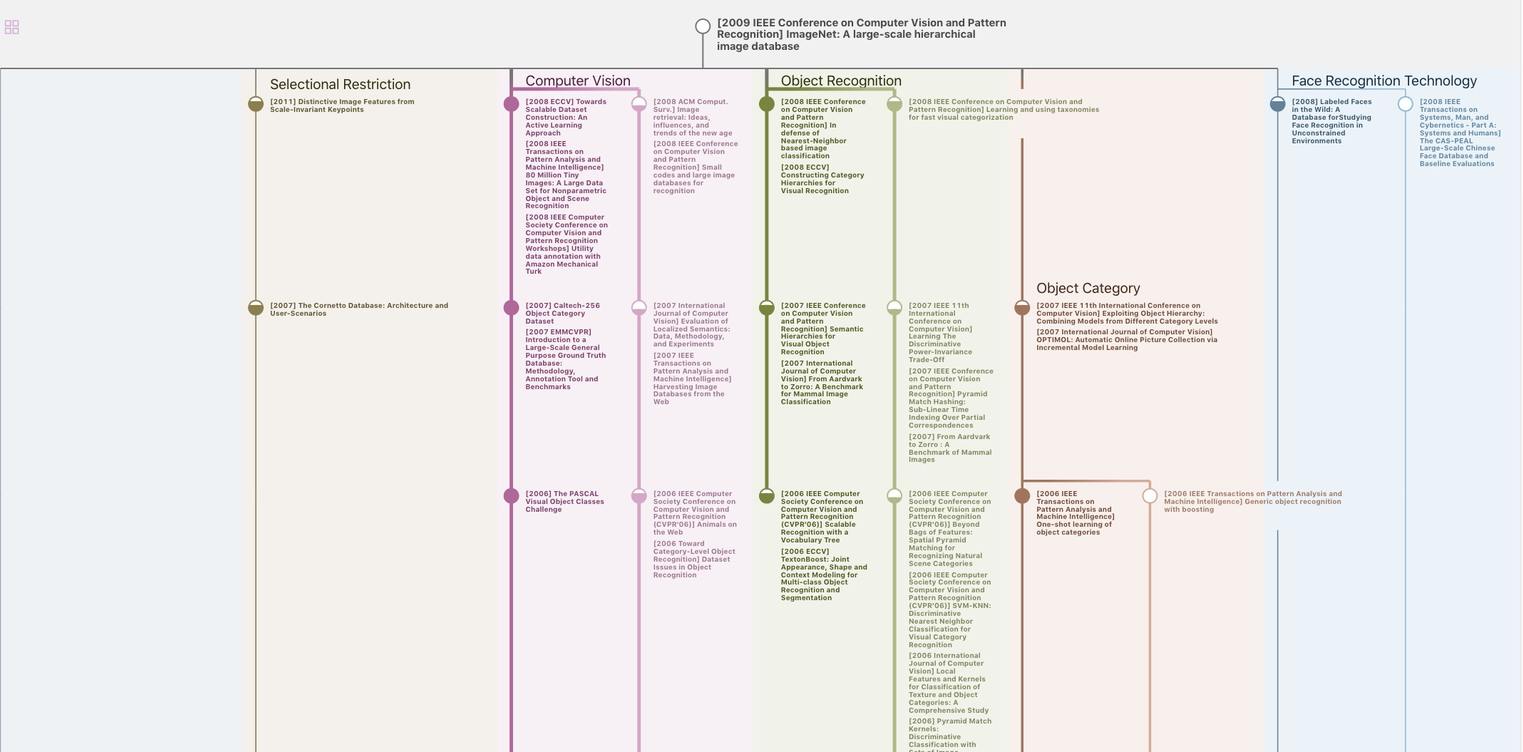
Generate MRT to find the research sequence of this paper
Chat Paper
Summary is being generated by the instructions you defined