Nonlinear Effects of Landscape Patterns on Ecosystem Services at Multiple Scales Based on Gradient Boosting Decision Tree Models
Remote Sensing(2023)
摘要
Exploring the complex effects of landscape patterns on ecosystem services (ESs) has become increasingly important in offering scientific support for effective spatial planning and ecosystem management. However, there is a particular lack of research on the nonlinear effects of landscape patterns on ESs and scale dependence. Taking Huainan City (in China) as a case study, this study adopted the InVEST model to estimate four key ESs including carbon storage (CS), habitat quality (HQ), nitrogen export (NE), and water yield (WY). Then, we calculated the selected landscape metrics at multiple spatial scales. Furthermore, the gradient boosting decision tree (GBDT) model was developed to investigate the relative importance of landscape metrics in explaining ESs and their nonlinear interrelation. The results indicated that most of the selected landscape metrics were significantly correlated with ESs. The GBDT model, which can explore nonlinear relationships, performed better than the linear regression model in explaining the variations in ESs. The landscape-level metrics of the Shannon's diversity index (SHDI) and the contagion index (CONTAG) and the class-level metrics of the aggregation index (AI) and edge density (ED) were the most important variables that influenced ESs. The landscape metrics affected ESs within a certain range, and the nonlinear effects varied with scale.
更多查看译文
关键词
ecosystem service,landscape pattern,nonlinear effect,scale dependence,gradient boosting decision tree (GBDT)
AI 理解论文
溯源树
样例
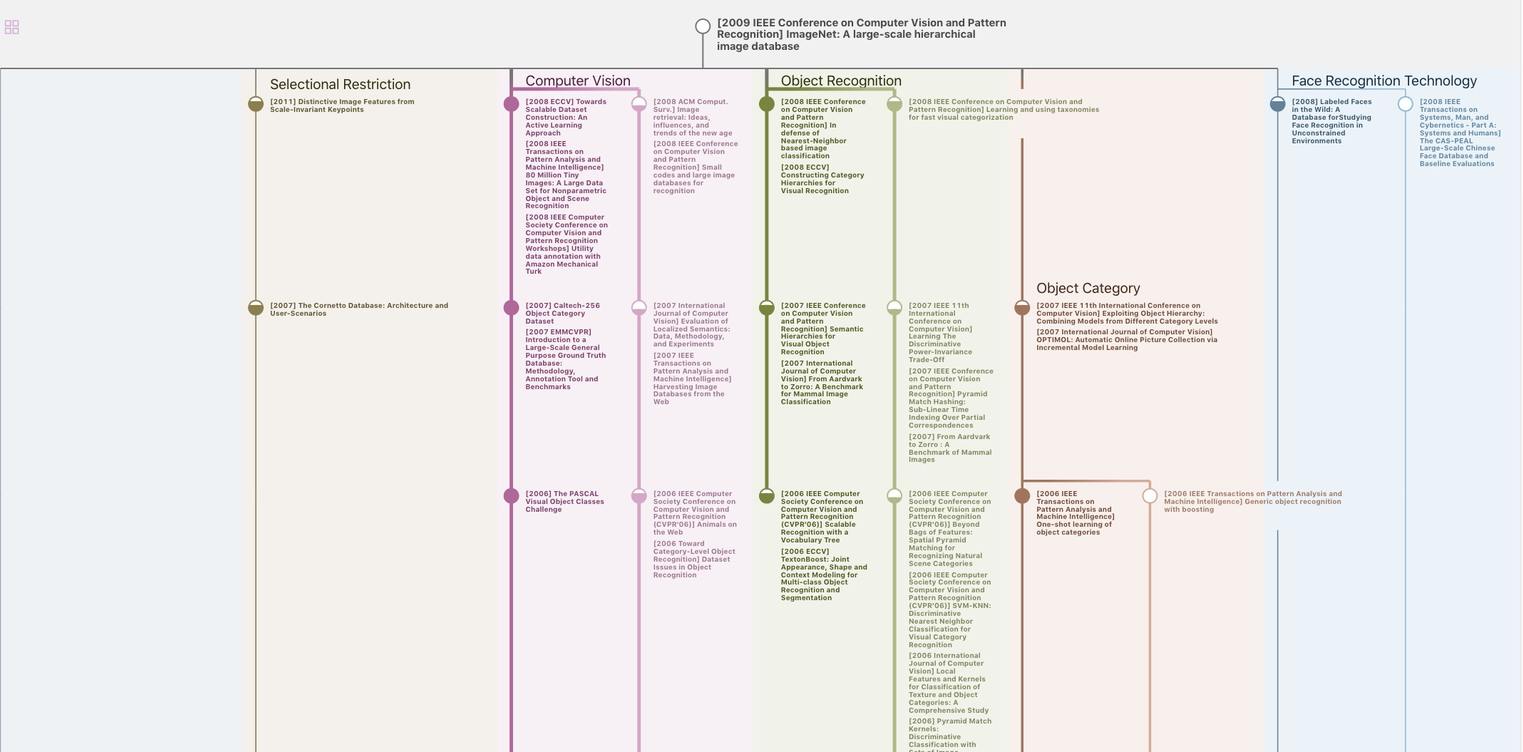
生成溯源树,研究论文发展脉络
Chat Paper
正在生成论文摘要