Improving Semantic Segmentation of Roof Segments Using Large-Scale Datasets Derived from 3D City Models and High-Resolution Aerial Imagery
Remote Sensing(2023)
摘要
Advances in deep learning techniques for remote sensing as well as the increased availability of high-resolution data enable the extraction of more detailed information from aerial images. One promising task is the semantic segmentation of roof segments and their orientation. However, the lack of annotated data is a major barrier for deploying respective models on a large scale. Previous research demonstrated the viability of the deep learning approach for the task, but currently, published datasets are small-scale, manually labeled, and rare. Therefore, this paper extends the state of the art by presenting a novel method for the automated generation of large-scale datasets based on semantic 3D city models. Furthermore, we train a model on a dataset 50 times larger than existing datasets and achieve superior performance while applying it to a wider variety of buildings. We evaluate the approach by comparing networks trained on four dataset configurations, including an existing dataset and our novel large-scale dataset. The results show that the network performance measured as intersection over union can be increased from 0.60 for the existing dataset to 0.70 when the large-scale model is applied on the same region. The large-scale model performs superiorly even when applied to more diverse test samples, achieving 0.635. The novel approach contributes to solving the dataset bottleneck and consequently to improving semantic segmentation of roof segments. The resulting remotely sensed information is crucial for applications such as solar potential analysis or urban planning.
更多查看译文
关键词
CityGML,3D city models,aerial images,remote sensing,dataset,labeling
AI 理解论文
溯源树
样例
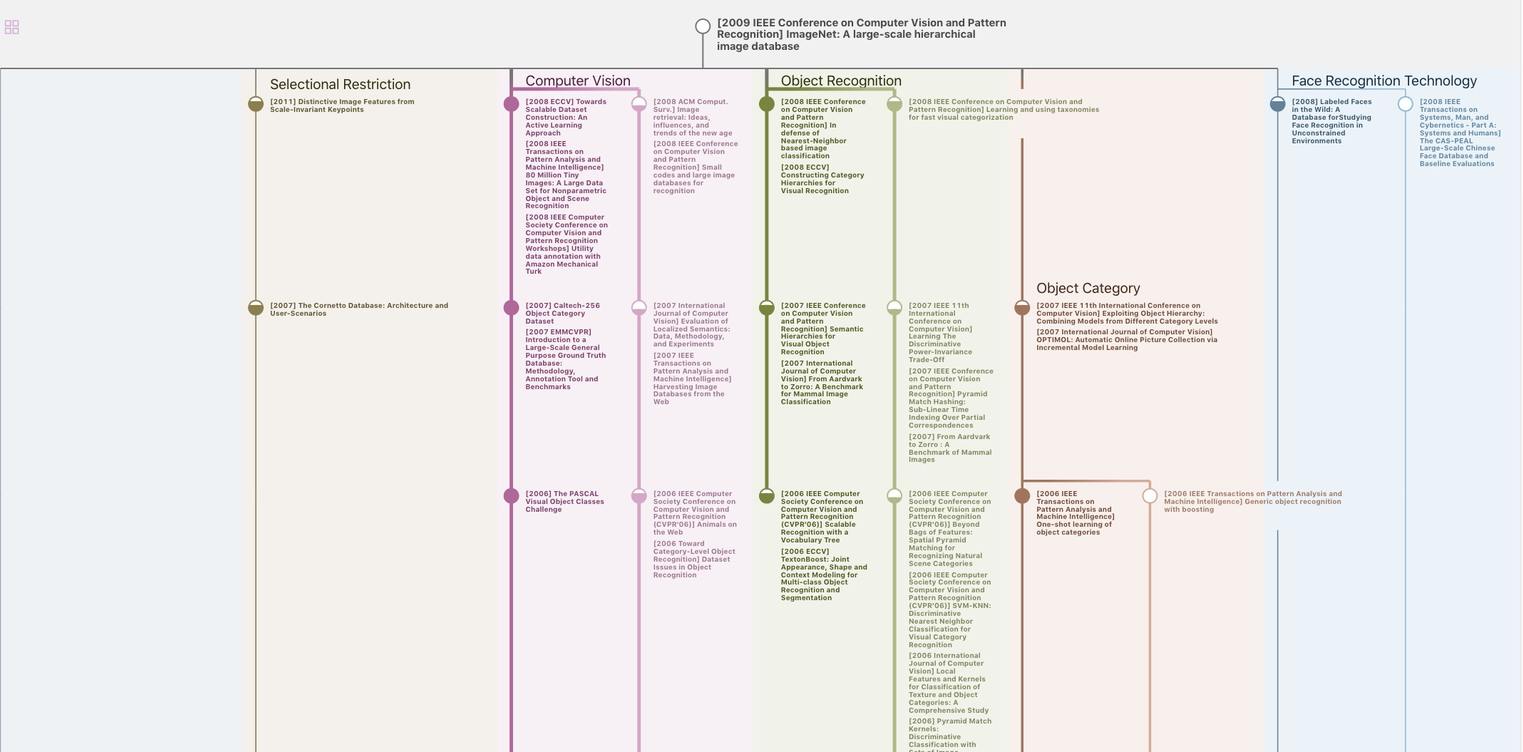
生成溯源树,研究论文发展脉络
Chat Paper
正在生成论文摘要