IBSA_Net: A Network for Tomato Leaf Disease Identification Based on Transfer Learning with Small Samples
Applied Sciences(2023)
摘要
Tomatoes are a crop of significant economic importance, and disease during growth poses a substantial threat to yield and quality. In this paper, we propose IBSA_Net, a tomato leaf disease recognition network that employs transfer learning and small sample data, while introducing the Shuffle Attention mechanism to enhance feature representation. The model is optimized by employing the IBMax module to increase the receptive field and adding the HardSwish function to the ConvBN layer to improve stability and speed. To address the challenge of poor generalization of models trained on public datasets to real environment datasets, we developed an improved PlantDoc++ dataset and utilized transfer learning to pre-train the model on PDDA and PlantVillage datasets. The results indicate that after pre-training on the PDDA dataset, IBSA_Net achieved a test accuracy of 0.946 on a real environment dataset, with an average precision, recall, and F1-score of 0.942, 0.944, and 0.943, respectively. Additionally, the effectiveness of IBSA_Net in other crops is verified. This study provides a dependable and effective method for recognizing tomato leaf diseases in real agricultural production environments, with the potential for application in other crops.
更多查看译文
关键词
tomato,transfer learning,small sample,IBSA_Net,disease recognition
AI 理解论文
溯源树
样例
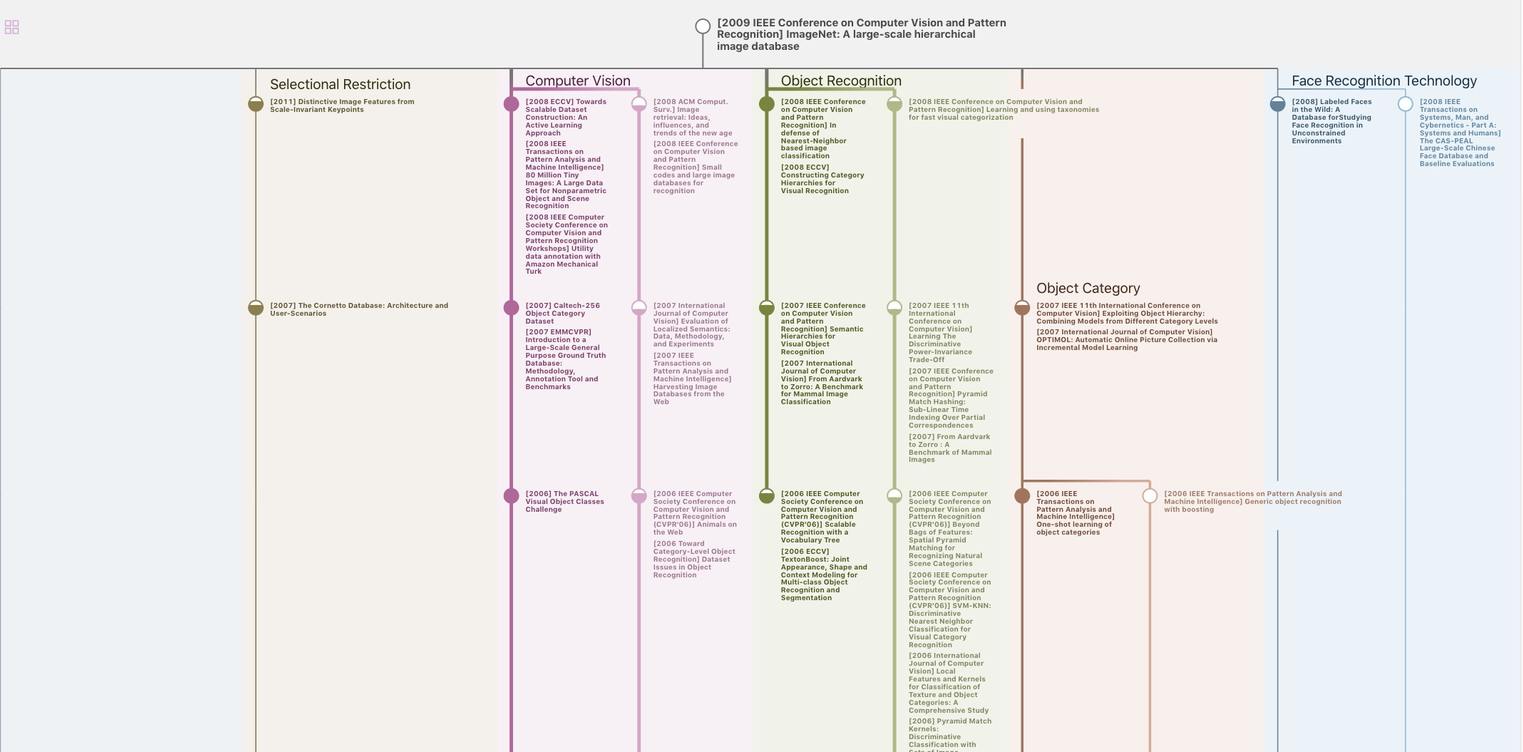
生成溯源树,研究论文发展脉络
Chat Paper
正在生成论文摘要