A Fuzzy Clustering Validity Index Induced by Triple Center Relation.
IEEE transactions on cybernetics(2023)
摘要
The existing clustering validity indexes (CVIs) show some difficulties to produce the correct cluster number when some cluster centers are close to each other, and the separation processing mechanism appears simple. The results are imperfect in case of noisy data sets. For this reason, in this study, we come up with a novel CVI for fuzzy clustering, referred to as the triple center relation (TCR) index. The originality of this index is twofold. On the one hand, a new fuzzy cardinality is built on the strength of the maximum membership degree, and a novel compactness formula is constructed by combining it with the within-class weighted squared error sum. On the other hand, starting from the minimum distance between different cluster centers, the mean distance as well as the sample variance of cluster centers in the statistical sense are further integrated. These three factors are combined by means of product to form a triple characterization of the relationship between cluster centers, and hence a 3-D expression pattern of separability is formed. Subsequently, the TCR index is put forward by combining the compactness formula with the separability expression pattern. By virtue of the degenerate structure of hard clustering, we show an important property of the TCR index. Finally, based on the fuzzy C -means (FCMs) clustering algorithm, experimental studies were conducted on 36 data sets (incorporating artificial and UCI data sets, images, the Olivetti face database). For comparative purposes, 10 CVIs were also considered. It has been found that the proposed TCR index performs best in finding the correct cluster number, and has excellent stability.
更多查看译文
关键词
Clustering validity index (CVI), compactness, fuzzy clustering, fuzzy c-means (FCMs), separability
AI 理解论文
溯源树
样例
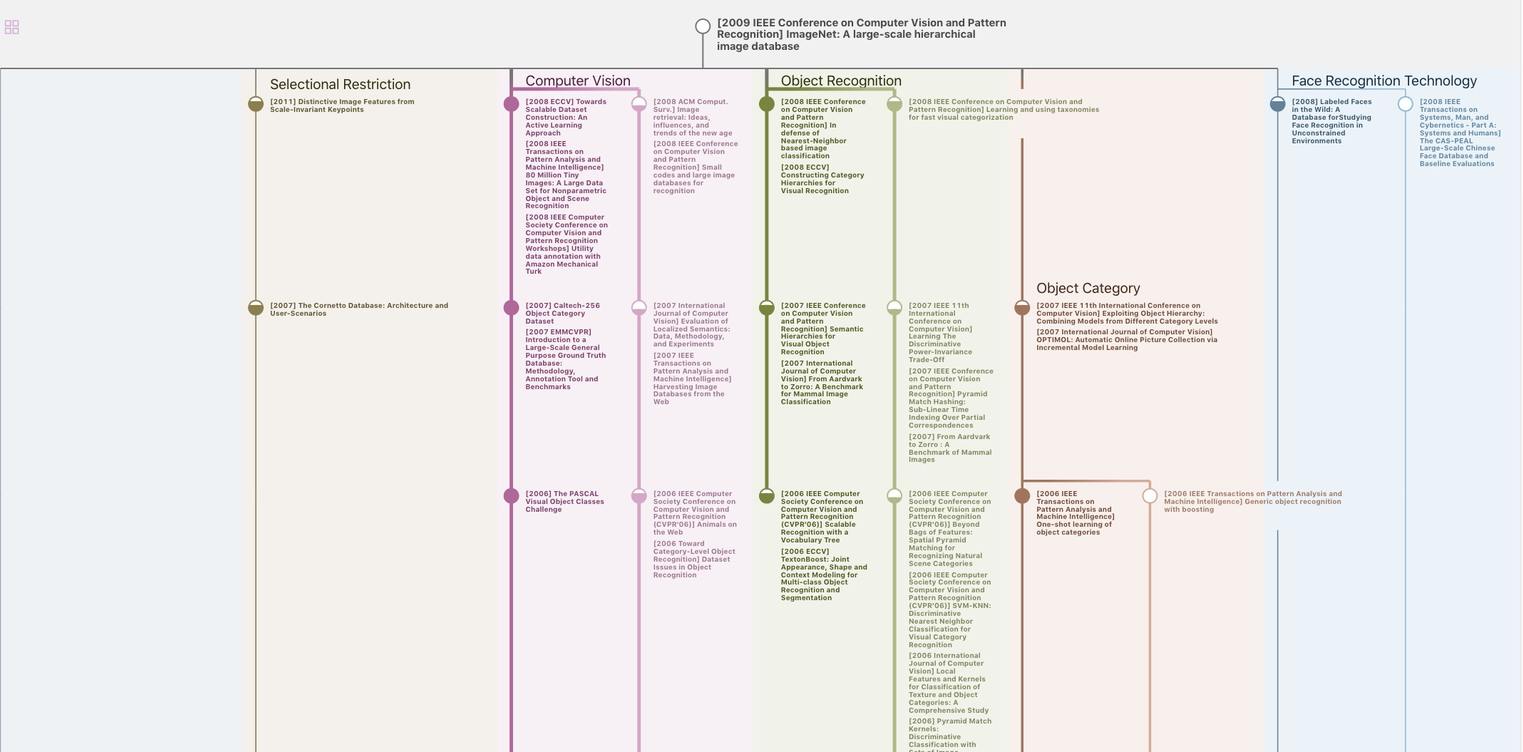
生成溯源树,研究论文发展脉络
Chat Paper
正在生成论文摘要