RTFusion: A Multimodal Fusion Network with Significant Information Enhancement
Journal of digital imaging(2023)
摘要
Multimodal medical fusion images are important for clinical diagnosis because they can better reflect the location of disease and provide anatomically detailed information. Existing medical image fusion methods can cause significant information loss in fusion images to varying degrees. Therefore, we designed a residual transformer fusion network (RTFusion): a multimodal fusion network with significant information enhancement. We use the residual transformer to make the image information interact remotely to ensure the global information of the image and use the residual structure to enhance the feature information to prevent information loss. Then the channel attention and spatial attention module (CASAM) is added to the fusion process to enhance the significant information of the fusion image, and the feature interaction module is used to promote the interaction of specific information of the source image. Finally, the loss function of the block calculation is designed to drive the fusion network to retain rich texture details, structural information, and color information, to optimize the subjective visual effect of the image. Extensive experiments show that our method can better recover the significant information of the source image and outperform other advanced methods in subjective visual description and objective metric evaluation. In particular, the color information and texture information are balanced to enhance the visual effect of the fused image.
更多查看译文
关键词
Image fusion,Significant information,Residual transformer,Channel attention and spatial attention module
AI 理解论文
溯源树
样例
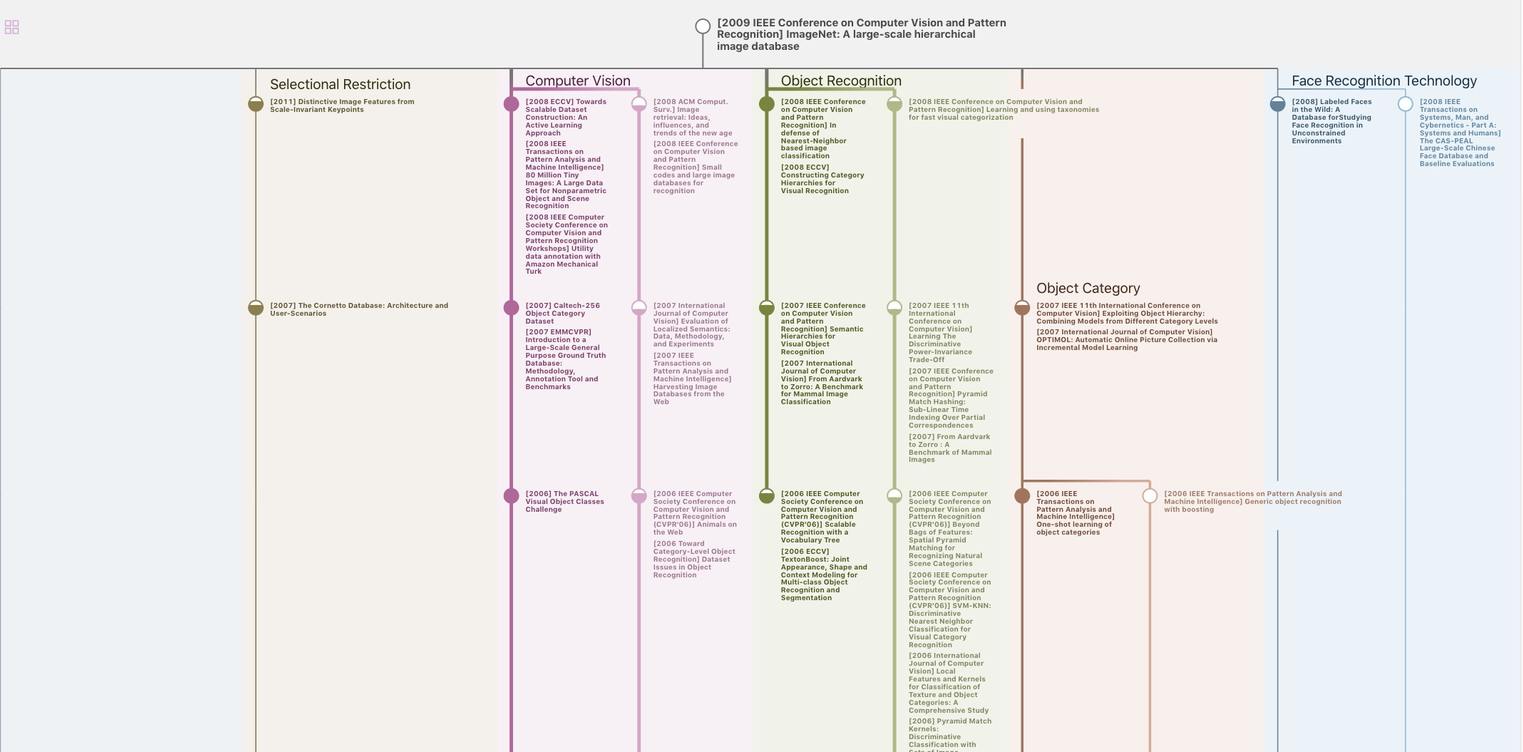
生成溯源树,研究论文发展脉络
Chat Paper
正在生成论文摘要