5613049 THE DIAGNOSTIC ACCURACY AND REPEATABILITY OF AN ARTIFICIAL INTELLIGENCE BASED SYSTEM TO OBTAIN AUTOMATED LIVER IRON CONCENTRATION MEASUREMENTS USING MAGNETIC RESONANCE IMAGING.
HemaSphere(2023)
AI 理解论文
溯源树
样例
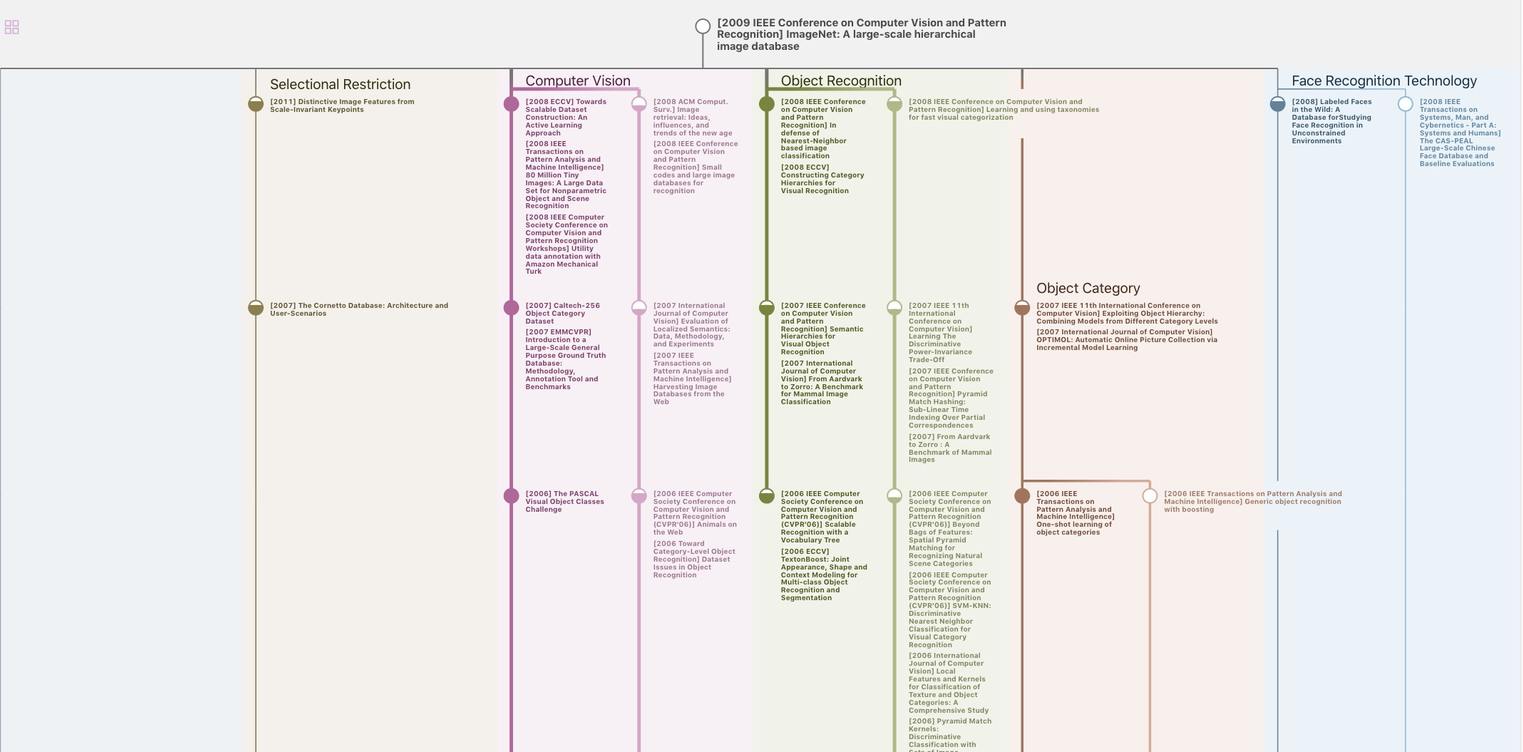
生成溯源树,研究论文发展脉络
Chat Paper
正在生成论文摘要