Towards preserving word order importance through FORCED INVALIDATION
17TH CONFERENCE OF THE EUROPEAN CHAPTER OF THE ASSOCIATION FOR COMPUTATIONAL LINGUISTICS, EACL 2023(2023)
摘要
Large pre-trained language models such as BERT have been widely used as a framework for natural language understanding (NLU) tasks. However, recent findings have revealed that pre-trained language models are insensitive to word order. The performance on NLU tasks remains unchanged even after randomly permuting the word of a sentence, where crucial syntactic information is destroyed. To help preserve the importance of word order, we propose a simple approach called FORCED INVALIDATION (FI): forcing the model to identify permuted sequences as invalid samples. We perform an extensive evaluation of our approach on various English NLU and QA based tasks over BERT-based and attention-based models over word embeddings. Our experiments demonstrate that FI significantly improves the sensitivity of the models to word order.(1)
更多查看译文
关键词
word order importance,forced invalidation
AI 理解论文
溯源树
样例
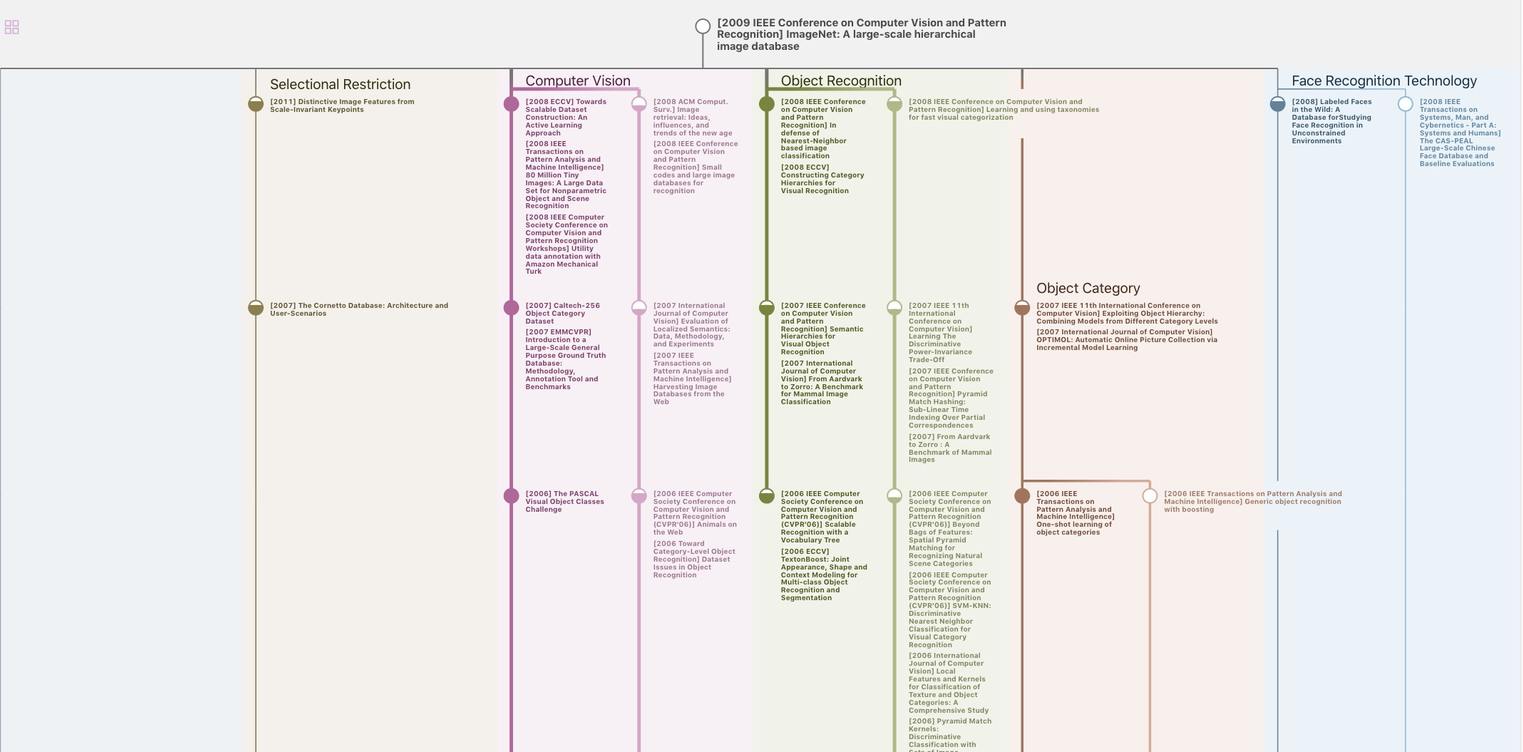
生成溯源树,研究论文发展脉络
Chat Paper
正在生成论文摘要