Cluster-Based Toxicity Estimation of Osteoradionecrosis via Unsupervised Machine Learning: Moving Beyond Single Dose-Parameter Normal Tissue Complication Probability by Using Whole Dose-Volume Histograms for Cohort Risk Stratification
International Journal of Radiation Oncology*Biology*Physics(2024)
摘要
Purpose
Given the limitations of extant models for normal tissue complication probability estimation for osteoradionecrosis (ORN) of the mandible, the purpose of this study was to enrich statistical inference by exploiting structural properties of data and provide a clinically reliable model for ORN risk evaluation through an unsupervised-learning analysis that incorporates the whole radiation dose distribution on the mandible.
Materials and Methods
The analysis was conducted on retrospective data of 1,259 head and neck cancer (HNC) patients treated at XXX between 2005 and 2015. During a minimum 12-month post-therapy follow-up period, 173 patients in this cohort (13.7%) developed ORN (grades I to IV). The (structural) clusters of mandibular dose-volume histograms (DVHs) for these patients were identified using the K-means clustering method. A soft-margin support vector machine (SVM) was used to determine the cluster borders and partition the dose-volume space. The risk of ORN for each dose-volume region was calculated based on incidence rates and other clinical risk factors.
Results
The K-means clustering method identified six clusters among the DVHs. Based on the first five clusters, the dose-volume space was partitioned by the soft-margin SVM into distinct regions with different risk indices. The sixth cluster entirely overlapped with the others; the region of this cluster was determined by its envelopes. For each region, the ORN incidence rate per pre-radiation dental extraction status (a statistically significant, non-dose related risk factor for ORN) was reported as the corresponding risk index.
Conclusion
This study presents an unsupervised-learning analysis of a large-scale data set to evaluate the risk of mandibular ORN among HNC patients. The results provide a visual risk-assessment tool for ORN (based on the whole DVH and pre-radiation dental extraction status) as well as a range of constraints for dose optimization under different risk levels.
更多查看译文
关键词
Radiation injuries,Osteoradionecrosis,normal tissue complication probability,Clustering,Unsupervised machine learning
AI 理解论文
溯源树
样例
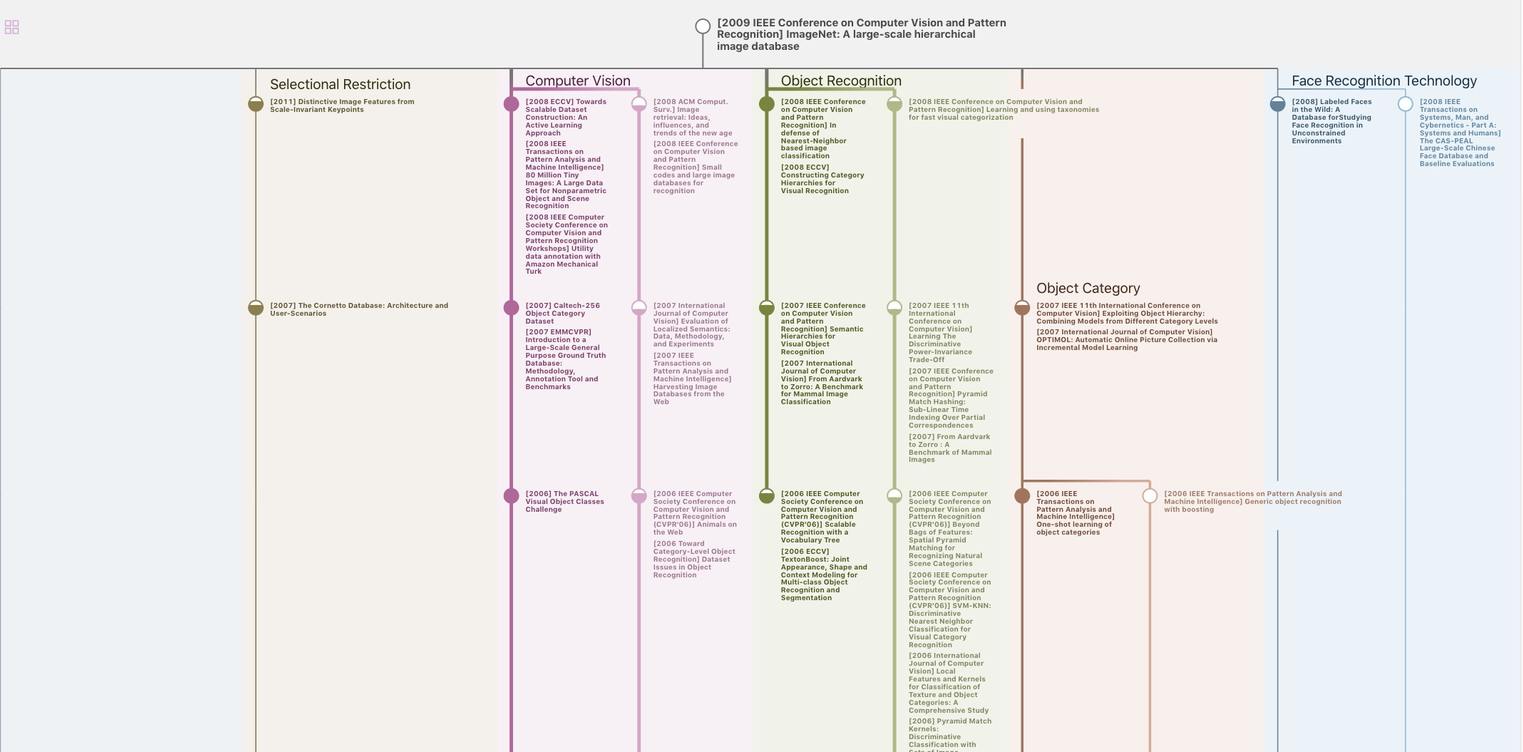
生成溯源树,研究论文发展脉络
Chat Paper
正在生成论文摘要