Building a Better Forecast: Reformulating the Ensemble Kalman Filter for Improved Applications to Volcano Deformation.
Earth and space science (Hoboken, N.J.)(2023)
摘要
As the volume of data collected at monitored volcanoes continues to expand, researchers will need quick, reliable, and automated methods of inverting those data into useful models of the underlying magma systems. Recently adapted from other fields for use in volcanology, the Ensemble Kalman Filter (EnKF) is one such inversion technique that has been used to produce several successful forecasts and hind-casts of volcanic unrest, correlating geodetic deformation with mechanical stresses around the magma reservoir. However, given the similarity in which changes to a reservoir's size and pressure are expressed at the surface, the filter can have trouble fully resolving magmatic conditions. In this study, we therefore test several different published variations of the EnKF workflow to produce an optimal configuration for use in future forecasting efforts. By generating synthetic observations of ground deformation under known conditions and then assimilating them through different implementations of the EnKF, we find that many variants favored in other fields underperform for this specific application. We conclude that correlations between model parameters that develop within the EnKF's Monte Carlo ensemble distort the filter's ability to correctly update the model state, causing the filter to systematically favor changes in some parameters over others and ultimately converge to a partially inaccurate solution. This effect can be somewhat mitigated by interrupting these parameter correlations, and the filter remains sensitive to many aspects of the magma system regardless. However, further research and novel approaches will be needed to truly optimize the EnKF for use in volcanology.
更多查看译文
关键词
EnKF,data assimilation,eruption forecasting,synthetic models,volcanology
AI 理解论文
溯源树
样例
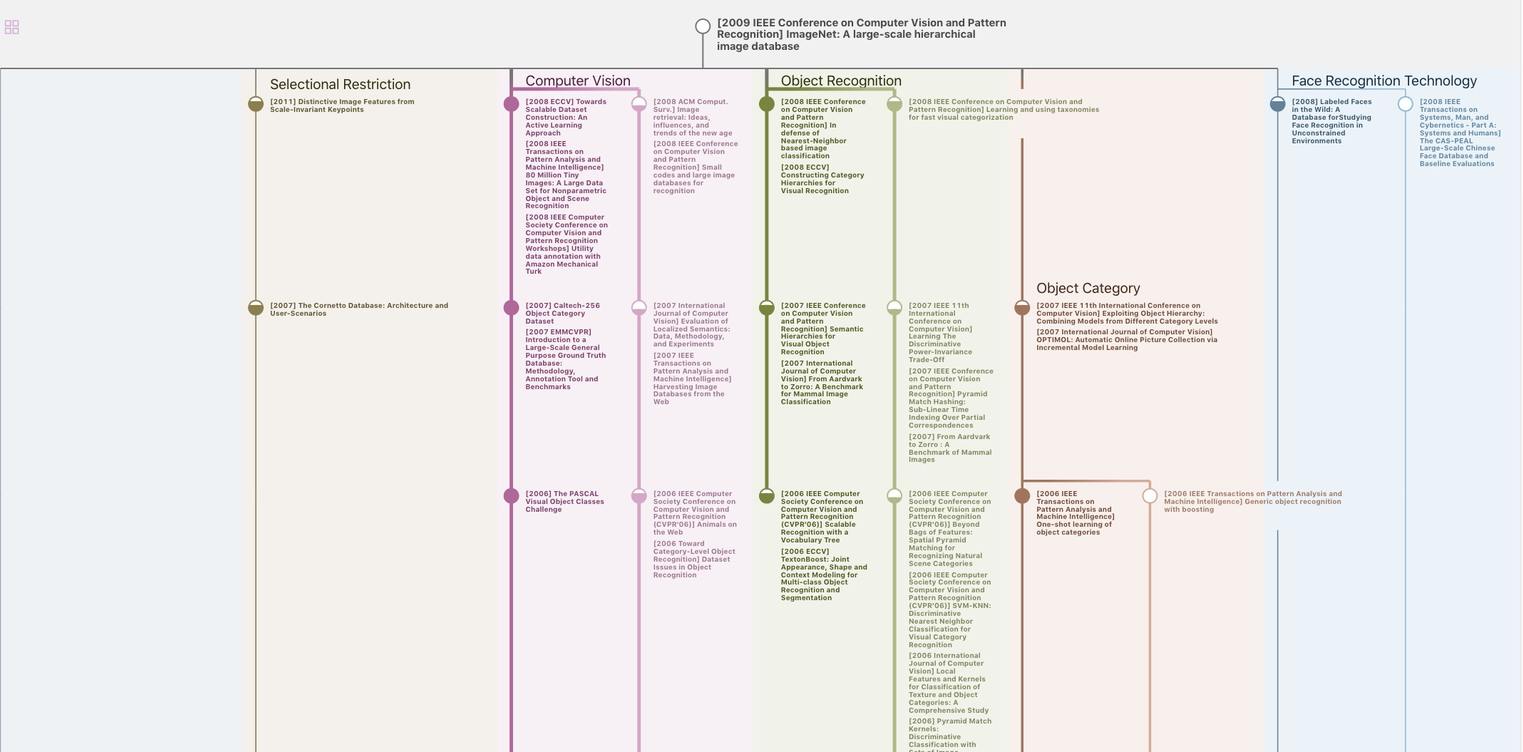
生成溯源树,研究论文发展脉络
Chat Paper
正在生成论文摘要