Hyperspectral Image Super-Resolution via Dual-Domain Network Based on Hybrid Convolution
IEEE TRANSACTIONS ON GEOSCIENCE AND REMOTE SENSING(2024)
Abstract
Hyperspectral images (HSIs) with high spatial resolution are challenging to obtain directly due to sensor limitations. Deep learning is able to provide an end-to-end reconstruction solution from low to high spatial resolution. Nevertheless, existing deep learning-based methods have two main drawbacks. First, deep networks with self-attention mechanisms often require a trade-off between internal resolution, model performance, and complexity, leading to the loss of fine-grained, high-resolution (HR) features. Second, there are visual discrepancies between the reconstructed HSI and the ground truth because they focus on spatial-spectral domain learning. In this article, a novel super-resolution (SR) algorithm for HSIs, named SRDNet, is proposed by using a dual-domain network with hybrid convolution and progressive upsampling to exploit both spatial-spectral and frequency information of the hyperspectral data. In this approach, we design a self-attentive pyramid structure (HSL) to capture interspectral self-similarity in the spatial domain, thereby increasing the receptive range of attention and improving the feature representation of the network. Additionally, we introduce a hyperspectral frequency loss (HFL) with dynamic weighting to optimize the model in the frequency domain and improve the perceptual quality of the HSI. Experimental results on three benchmark datasets show that SRDNet effectively improves the texture information of the HSI and outperforms state-of-the-art methods. The code is available at https://github.com/LTTdouble/SRDNet.
MoreTranslated text
Key words
Dual-domain network,frequency loss,hyperspectral image,self-attention mechanism,super-resolution (SR)
AI Read Science
Must-Reading Tree
Example
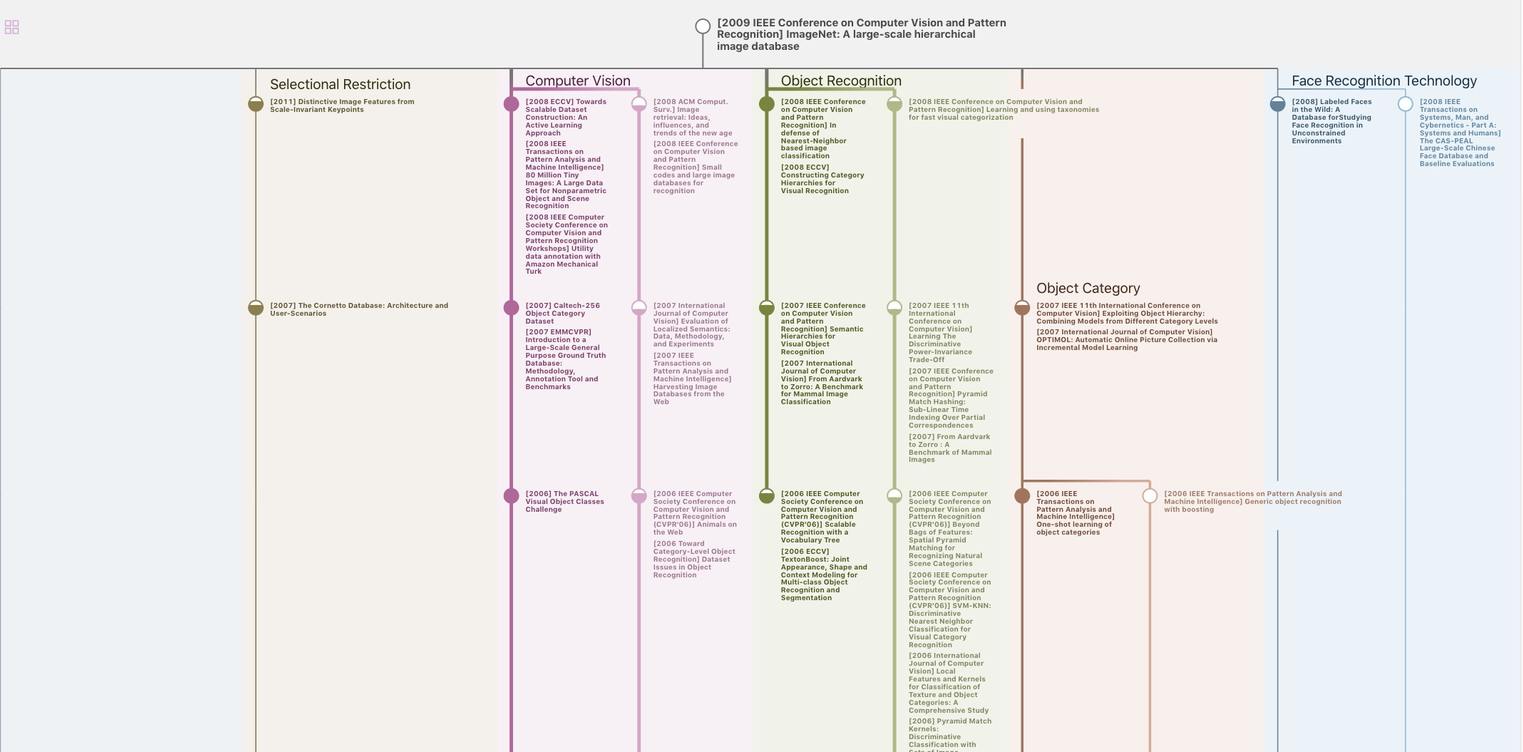
Generate MRT to find the research sequence of this paper
Chat Paper
Summary is being generated by the instructions you defined