RD-DPP: Rate-Distortion Theory Meets Determinantal Point Process to Diversify Learning Data Samples
CoRR(2023)
摘要
In some practical learning tasks, such as traffic video analysis, the number of available training samples is restricted by different factors, such as limited communication bandwidth and computation power; therefore, it is imperative to select diverse data samples that contribute the most to the quality of the learning system. One popular approach to selecting diverse samples is Determinantal Point Process (DPP). However, it suffers from a few known drawbacks, such as restriction of the number of samples to the rank of the similarity matrix, and not being customizable for specific learning tasks (e.g., multi-level classification tasks). In this paper, we propose a new way of measuring task-oriented diversity based on the Rate-Distortion (RD) theory, appropriate for multi-level classification. To this end, we establish a fundamental relationship between DPP and RD theory, which led to designing RD-DPP, an RD-based value function to evaluate the diversity gain of data samples. We also observe that the upper bound of the diversity of data selected by DPP has a universal trend of phase transition that quickly approaches its maximum point, then slowly converges to its final limits, meaning that DPP is beneficial only at the beginning of sample accumulation. We use this fact to design a bi-modal approach for sequential data selection.
更多查看译文
关键词
determinantal point process,learning,rd-dpp,rate-distortion
AI 理解论文
溯源树
样例
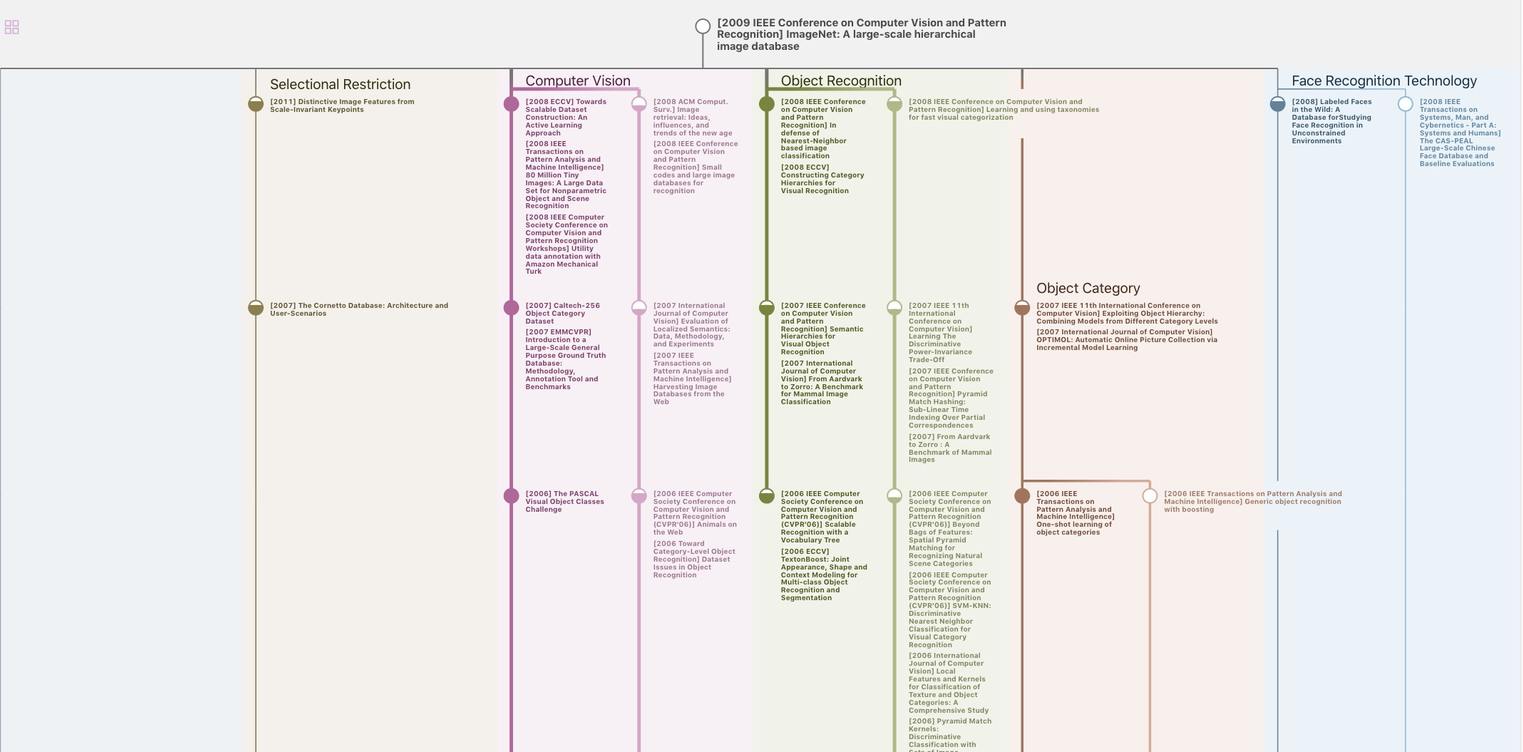
生成溯源树,研究论文发展脉络
Chat Paper
正在生成论文摘要