A Simple Proof of the Mixing of Metropolis-Adjusted Langevin Algorithm under Smoothness and Isoperimetry
CoRR(2023)
摘要
We study the mixing time of Metropolis-Adjusted Langevin algorithm (MALA) for sampling a target density on $\mathbb{R}^d$. We assume that the target density satisfies $\psi_\mu$-isoperimetry and that the operator norm and trace of its Hessian are bounded by $L$ and $\Upsilon$ respectively. Our main result establishes that, from a warm start, to achieve $\epsilon$-total variation distance to the target density, MALA mixes in $O\left(\frac{(L\Upsilon)^{\frac12}}{\psi_\mu^2} \log\left(\frac{1}{\epsilon}\right)\right)$ iterations. Notably, this result holds beyond the log-concave sampling setting and the mixing time depends on only $\Upsilon$ rather than its upper bound $L d$. In the $m$-strongly logconcave and $L$-log-smooth sampling setting, our bound recovers the previous minimax mixing bound of MALA~\cite{wu2021minimax}.
更多查看译文
关键词
mixing,smoothness,isoperimetry,metropolis-adjusted
AI 理解论文
溯源树
样例
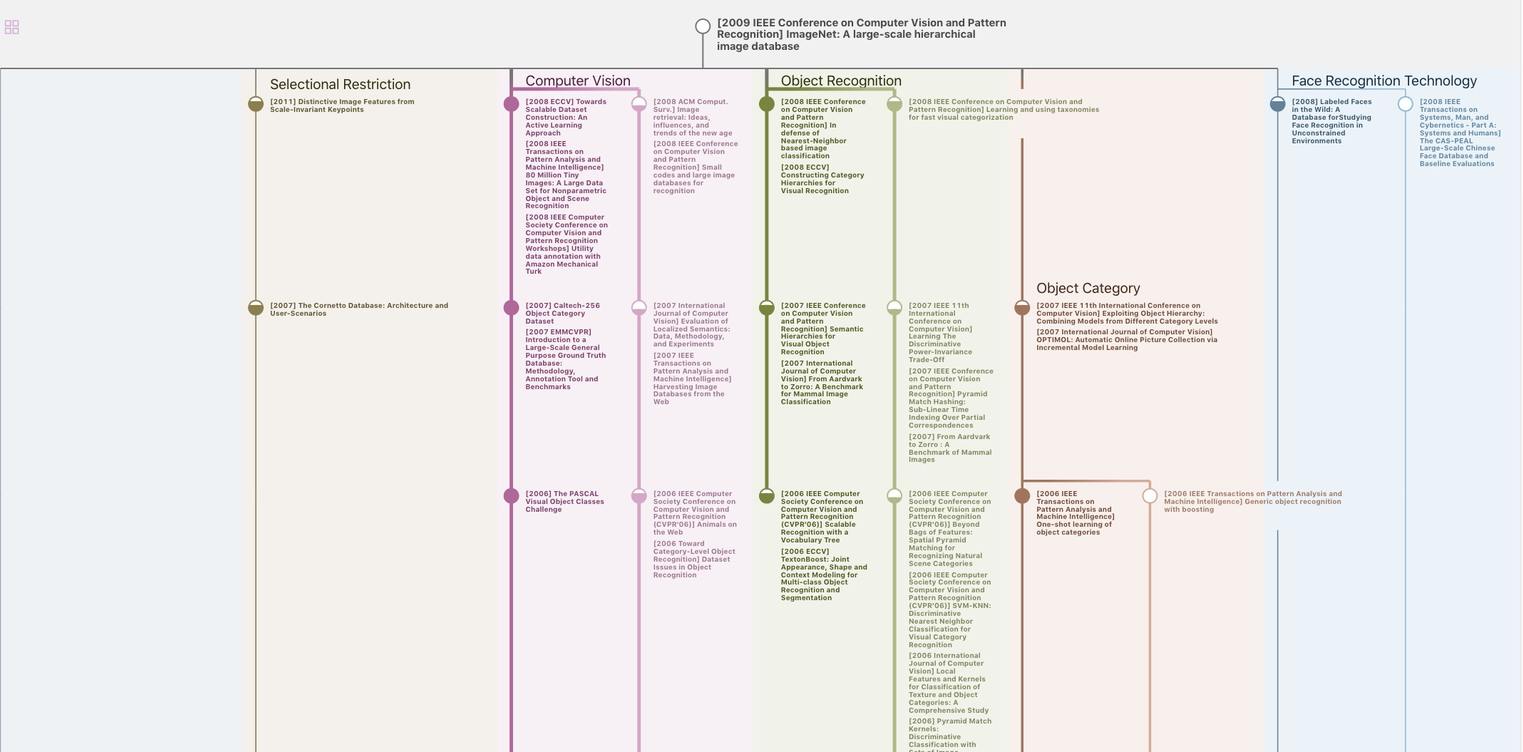
生成溯源树,研究论文发展脉络
Chat Paper
正在生成论文摘要