Model-Agnostic Decentralized Collaborative Learning for On-Device POI Recommendation
PROCEEDINGS OF THE 46TH INTERNATIONAL ACM SIGIR CONFERENCE ON RESEARCH AND DEVELOPMENT IN INFORMATION RETRIEVAL, SIGIR 2023(2023)
摘要
As an indispensable personalized service in Location-based Social Networks (LBSNs), the next Point-of-Interest (POI) recommendation aims to help people discover attractive and interesting places. Currently, most POI recommenders are based on the conventional centralized paradigm that heavily relies on the cloud to train the recommendation models with large volumes of collected users' sensitive check-in data. Although a few recent works have explored on-device frameworks for resilient and privacy-preserving POI recommendations, they invariably hold the assumption of model homogeneity for parameters/gradients aggregation and collaboration. However, users' mobile devices in the real world have various hardware configurations (e.g., compute resources), leading to heterogeneous on-device models with different architectures and sizes. In light of this, We propose a novel on-device POI recommendation framework, namely Model-Agnostic Collaborative learning for ondevice POI recommendation (MAC), allowing users to customize their own model structures (e.g., dimension & number of hidden layers). To counteract the sparsity of on-device user data, we propose to pre-select neighbors for collaboration based on physical distances, category-level preferences, and social networks. To assimilate knowledge from the above-selected neighbors in an efficient and secure way, we adopt the knowledge distillation framework with mutual information maximization. Instead of sharing sensitive models/gradients, clients in MAC only share their soft decisions on a preloaded reference dataset. To filter out low-quality neighbors, we propose two sampling strategies, performance-triggered sampling and similarity-based sampling, to speed up the training process and obtain optimal recommenders. In addition, we design two novel approaches to generate more effective reference datasets while protecting users' privacy. Extensive experiments on two datasets have shown the superiority of MAC over advanced baselines.
更多查看译文
关键词
Point-of-Interest Recommendation,Decentralized Collaborative Learning,Model-Agnostic
AI 理解论文
溯源树
样例
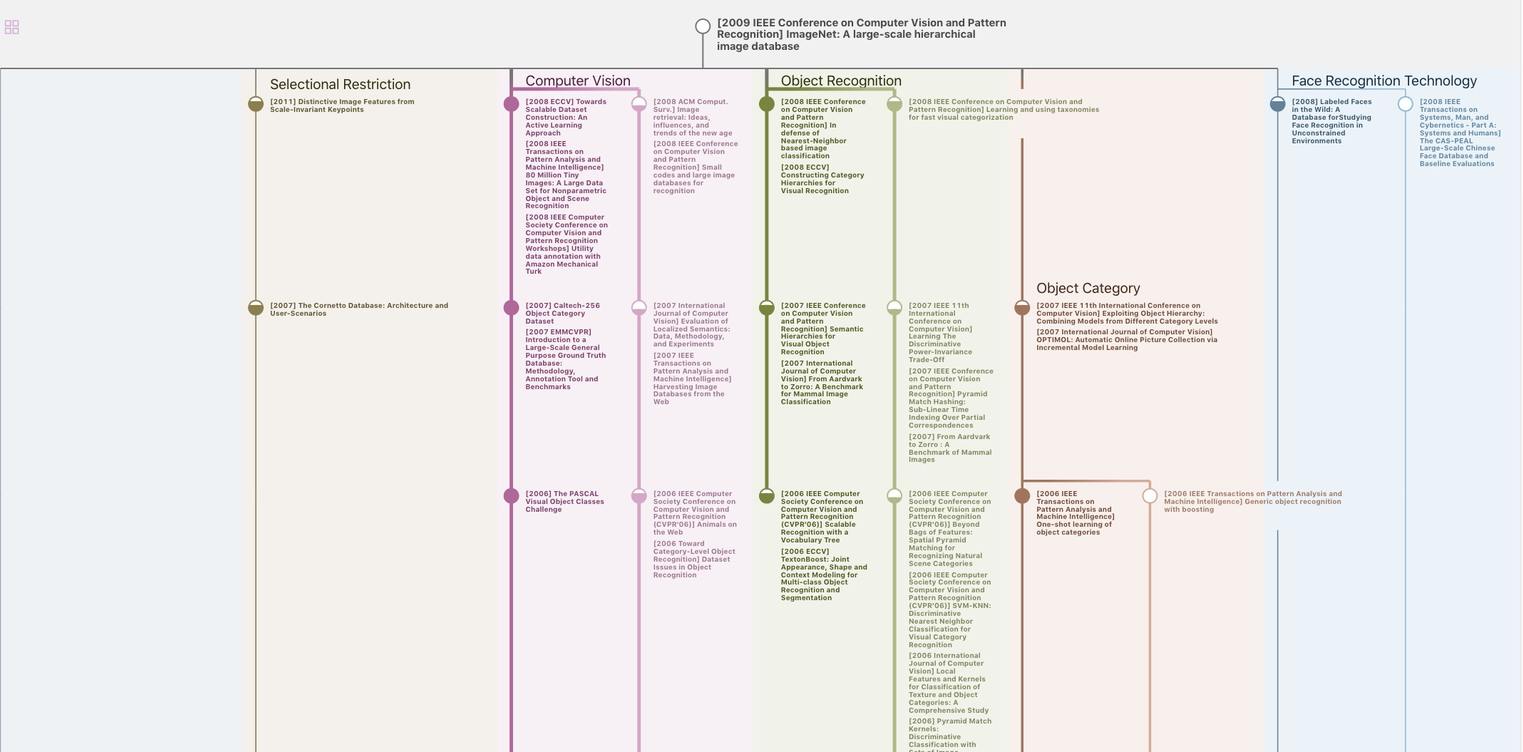
生成溯源树,研究论文发展脉络
Chat Paper
正在生成论文摘要