OFTER: An Online Pipeline for Time Series Forecasting
CoRR(2023)
摘要
We introduce OFTER, a time series forecasting pipeline tailored for mid-sized multivariate time series. OFTER utilizes the non-parametric models of k-nearest neighbors and Generalized Regression Neural Networks, integrated with a dimensionality reduction component. To circumvent the curse of dimensionality, we employ a weighted norm based on a modified version of the maximal correlation coefficient. The pipeline we introduce is specifically designed for online tasks, has an interpretable output, and is able to outperform several state-of-the art baselines. The computational efficacy of the algorithm, its online nature, and its ability to operate in low signal-to-noise regimes, render OFTER an ideal approach for financial multivariate time series problems, such as daily equity forecasting. Our work demonstrates that while deep learning models hold significant promise for time series forecasting, traditional methods carefully integrating mainstream tools remain very competitive alternatives with the added benefits of scalability and interpretability.
更多查看译文
关键词
forecasting,online pipeline,ofter,series
AI 理解论文
溯源树
样例
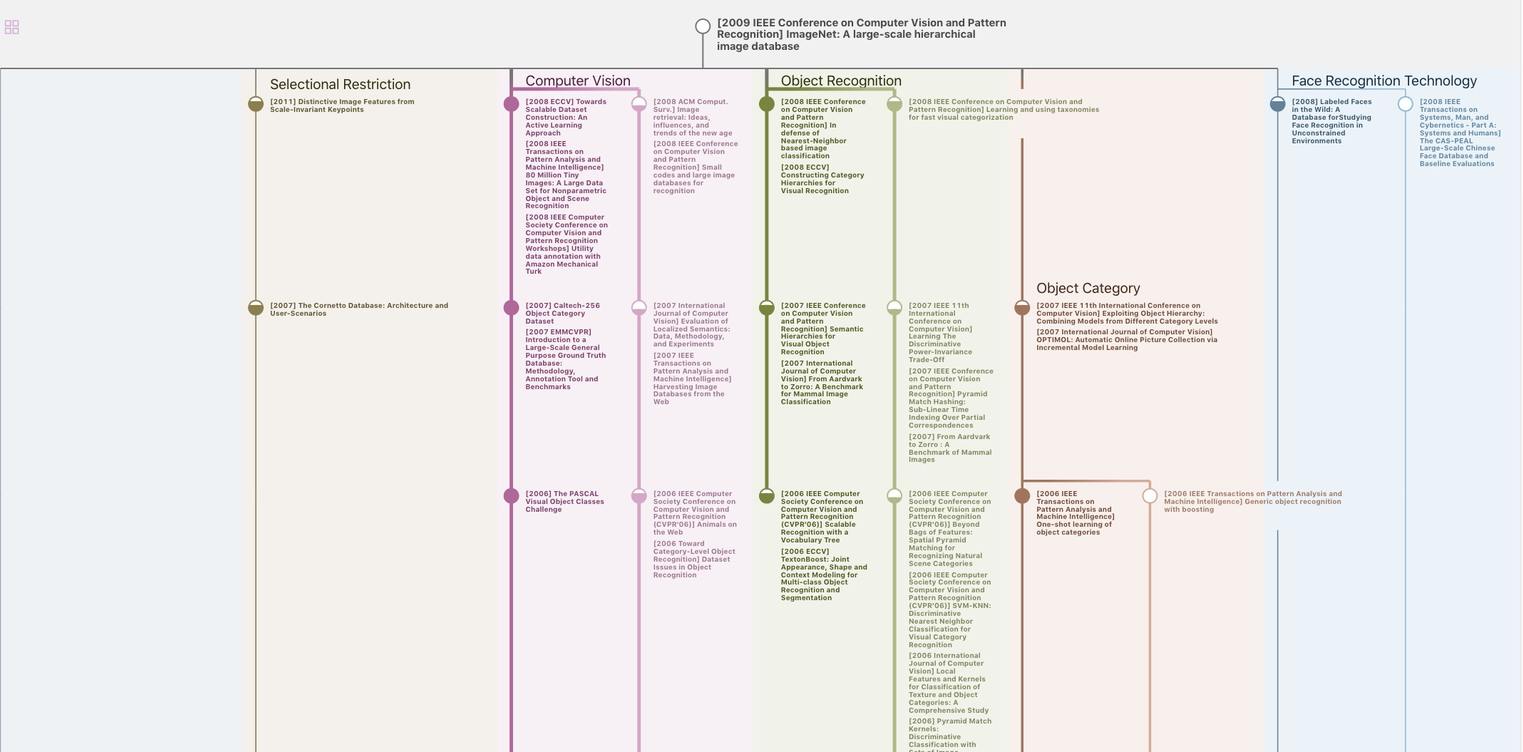
生成溯源树,研究论文发展脉络
Chat Paper
正在生成论文摘要