Monitoring and control of biological additive manufacturing using machine learning
Journal of Intelligent Manufacturing(2024)
摘要
The goal of this work is the flaw-free, industrial-scale production of biological additive manufacturing of tissue constructs (Bio-AM). In pursuit of this goal, the objectives of this work in the context of extrusion-based Bio-AM of bone tissue constructs are twofold: (1) detect flaw formation using data from in-situ infrared thermocouple sensors; and (2) prevent flaw formation through preemptive process control. In realizing the first objective, data signatures acquired from in-situ sensors were analyzed using several machine learning approaches to ascertain critical quality metrics, such as print regime, strand width, strand height, and strand fusion severity. These quality metrics are intended to capture the process state at the basic 1D strand-level to the 2D layer-level. For this purpose, machine learning models were trained to classify and predict flaw formation. These models predicted print quality features with accuracy nearing 90%. In connection with the second objective, the previously trained machine learning models were used to preempt flaw formation by changing the process parameters (print velocity) during deposition—a form of feedforward control. With the feedforward process control, strand width heterogeneity was statistically significantly reduced, reducing the strand width difference between strand halves to less than 50 µm. Using this integrated process monitoring, detection, and control approach, we demonstrate consistent, repeatable production of Bio-AM constructs.
更多查看译文
关键词
3D printing,Bone tissue,Poly(caprolactone) (PCL)-hydroxyapatite (HAp) composites,In-situ sensing
AI 理解论文
溯源树
样例
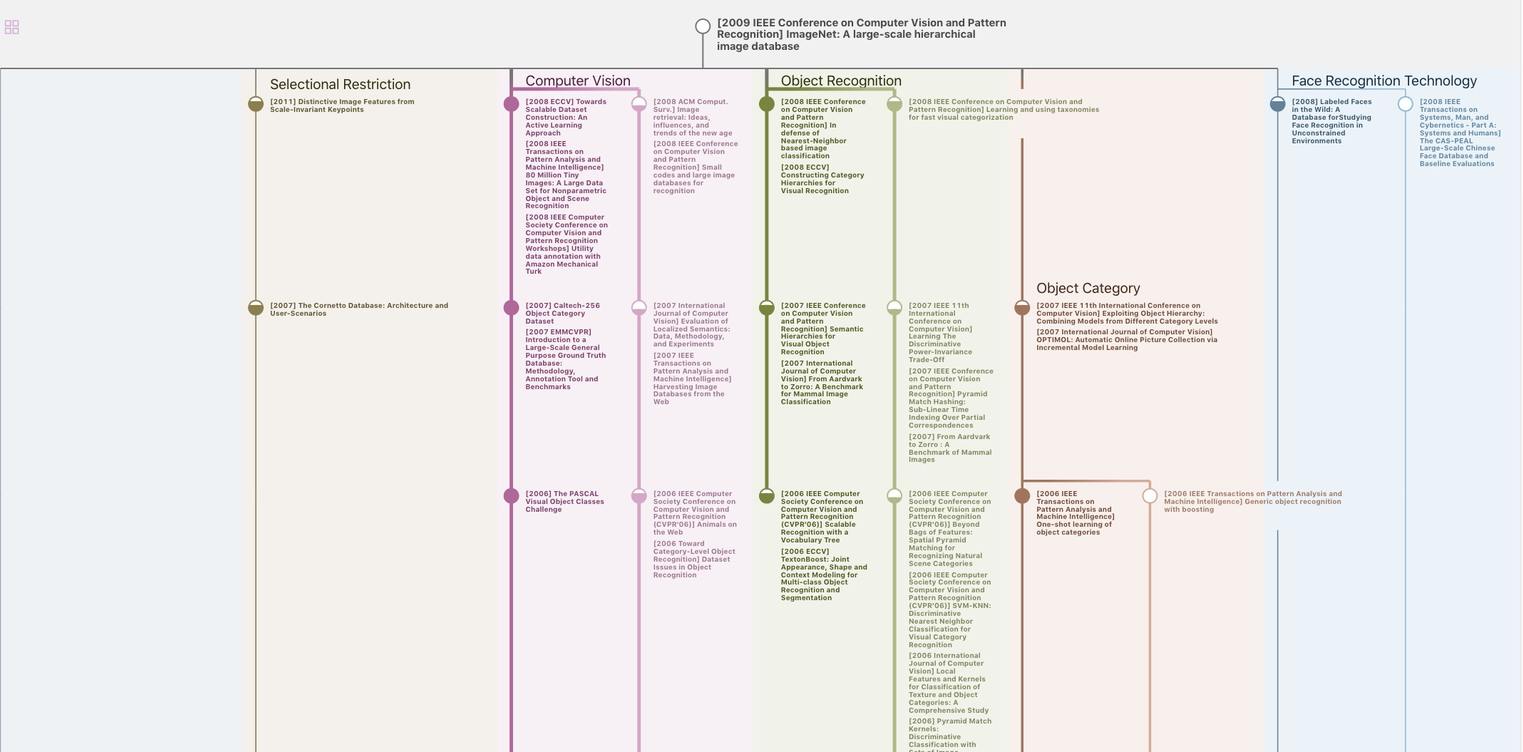
生成溯源树,研究论文发展脉络
Chat Paper
正在生成论文摘要