Very Large Scale Multi-Agent Reinforcement Learning with Graph Attention Mean Field
ICLR 2023(2023)
摘要
With recent advances in reinforcement learning, we have witnessed countless successes of intelligent agents in various domains. Especially, multi-agent reinforcement learning (MARL) is suitable for many real-world scenarios and has vast potential applications. However, typical MARL methods can only handle tens of agents, leaving scenarios with up to hundreds or even thousands of agents almost unexplored. There exist two key challenges in scaling up the number of agents: (1) agent-agent interactions are critical in multi-agent systems while the number of interactions grows quadratically with the number of agents, causing great computational complexity and difficulty in strategies-learning; (2) the strengths of interactions vary among agents and over time, making it difficult to precisely model such interactions. In this paper, we propose the Graph Attention Mean Field (GAT-MF) method, where we convert agent-agent interactions into interactions between each agent and a weighted mean field, greatly reducing the computational complexity. We mathematically prove the correctness of this conversion. We design a graph attention mechanism to automatically capture the different and time-varying strengths of interactions, ensuring the ability of our method to precisely model interactions among the agents. We conduct extensive experiments in both manual and real-world scenarios with up to more than 3000 agents, demonstrating that comparing existing MARL methods, our method reaches superior performance and 9.4 times computational efficiency.
更多查看译文
关键词
Multi-agent reinforcement learning,large-scale problems,graph attention,mean field
AI 理解论文
溯源树
样例
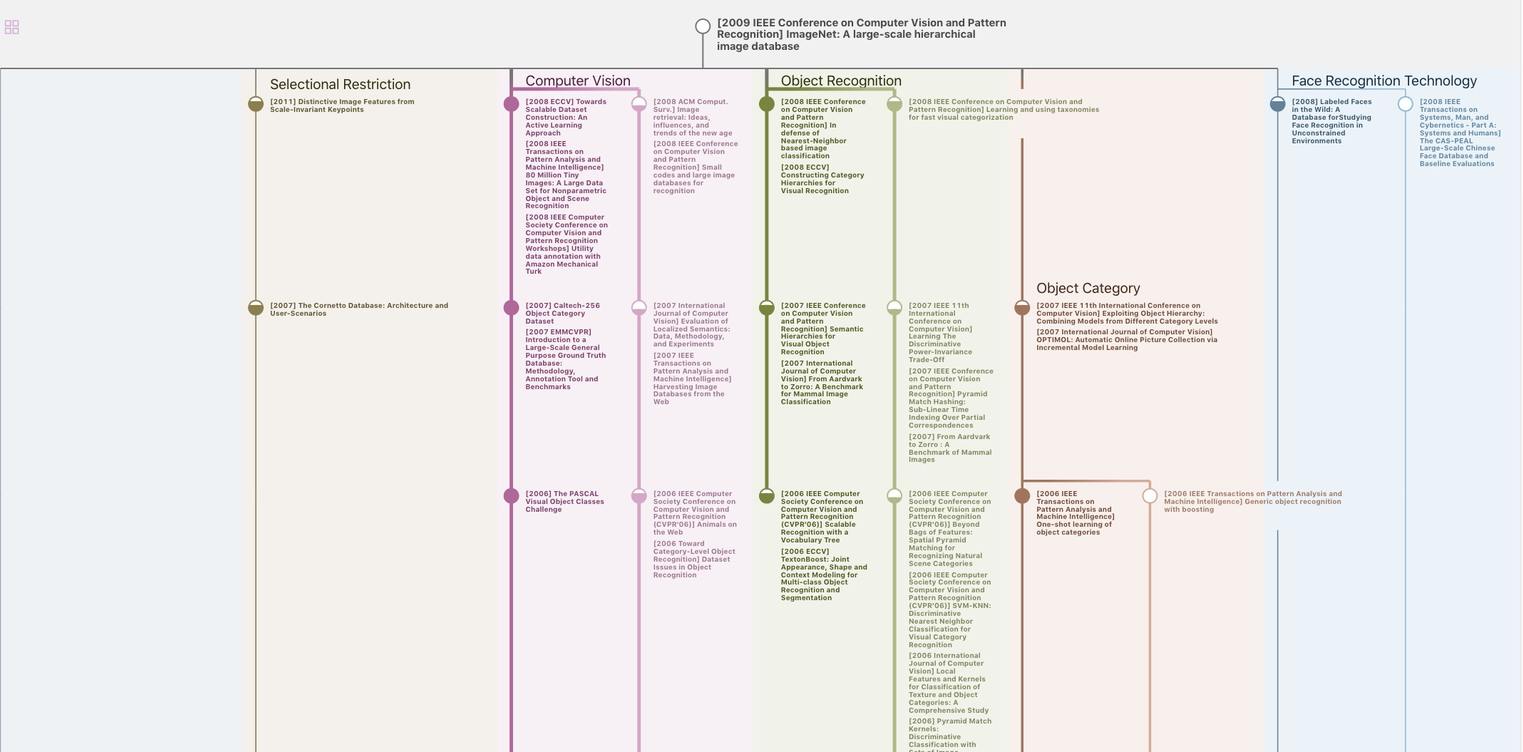
生成溯源树,研究论文发展脉络
Chat Paper
正在生成论文摘要