PointDP: Diffusion-driven Purification against 3D Adversarial Point Clouds
ICLR 2023(2023)
摘要
3D Point cloud is a critical data representation in many real-world applications, such as autonomous driving, robotics, and medical imaging. Although the success of deep learning further accelerates the adoption of 3D point clouds in the physical world, deep learning is notoriously vulnerable to adversarial attacks. Various defense solutions have been proposed to build robust models against adversarial attacks. In this work, we identify that the state-of-the-art empirical defense, adversarial training, has a major limitation in 3D point cloud models due to gradient obfuscation, resulting in significant degradation of robustness against strong attacks. To bridge the gap, we propose PointDP, a purification strategy that leverages diffusion models to defend against 3D adversarial attacks. Since PointDP does not rely on predefined adversarial examples for training, it can defend against diverse threats. We extensively evaluate PointDP on six representative 3D point cloud architectures and leverage sixteen strong and adaptive attacks to demonstrate its lower-bound robustness. Our evaluation shows that PointDP achieves significantly better (i.e., 12.6\%-40.3\%) adversarial robustness than state-of-the-art methods under strong attacks bounded by different $\ell_p$ norms.
更多查看译文
关键词
Adversarial Robustness,Point Cloud Classification,Diffusion Model
AI 理解论文
溯源树
样例
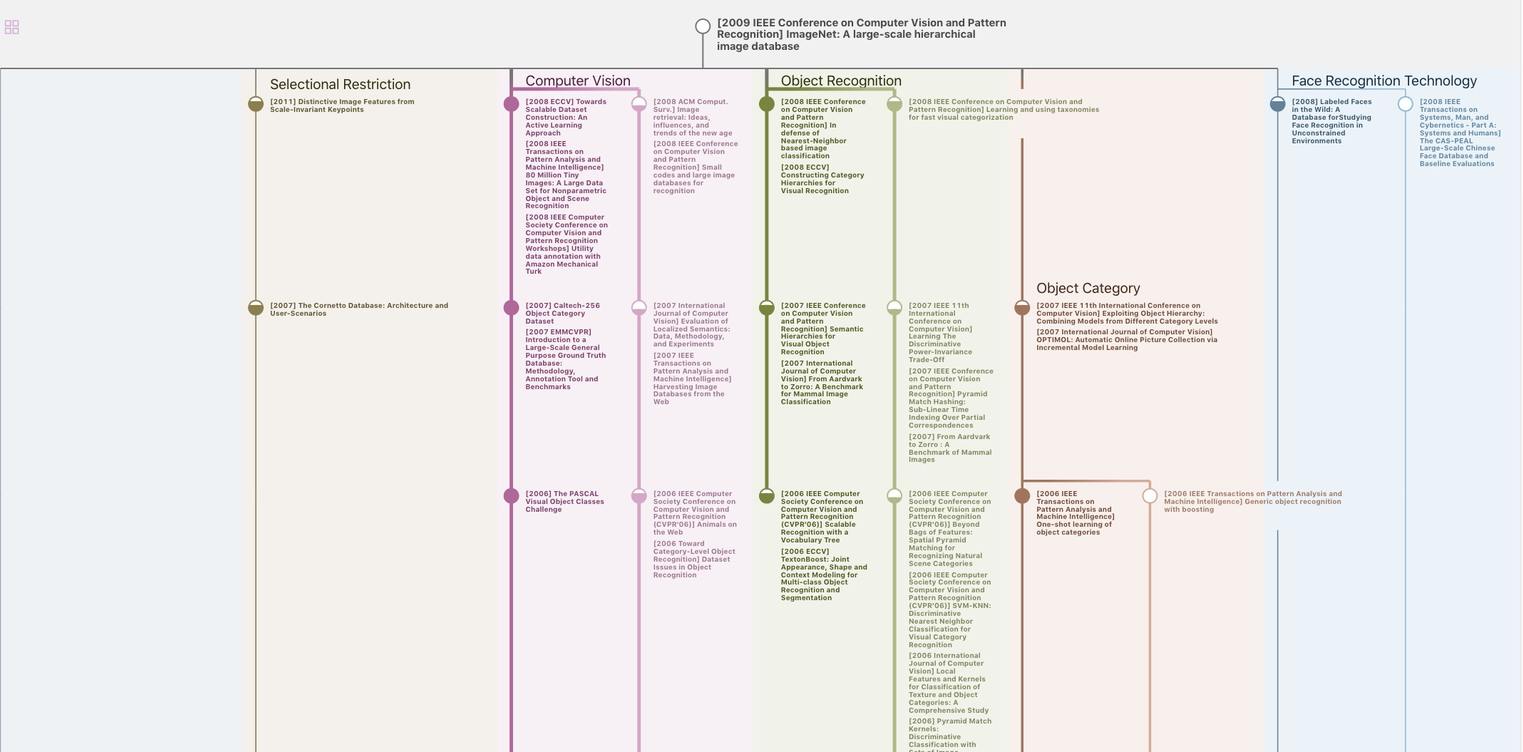
生成溯源树,研究论文发展脉络
Chat Paper
正在生成论文摘要