Online Continual Learning for Progressive Distribution Shift (OCL-PDS): A Practitioner's Perspective
ICLR 2023(2023)
摘要
We introduce the novel OCL-PDS problem - Online Continual Learning for Progressive Distribution Shift. PDS refers to the subtle, gradual, and continuous distribution shift that widely exists in modern deep learning applications. It is widely observed in industry that PDS can cause significant performance drop. While previous work in continual learning and domain adaptation addresses this problem to some extent, our investigations from the practitioner's perspective reveal flawed assumptions that limit their applicability on daily challenges faced in real-world scenarios, and this work aims to close the gap between academic research and industry. For this new problem, we build 4 new benchmarks from the Wilds dataset, and implement 12 algorithms and baselines including both supervised and semi-supervised methods, which we test extensively on the new benchmarks. We hope that this work can provide practitioners with tools to better handle realistic PDS, and help scientists design better OCL algorithms.
更多查看译文
关键词
Distribution shift,Continual learning,Domain Adaptation,Semi-supervised learning,Model drift,Online learning,Benchmark
AI 理解论文
溯源树
样例
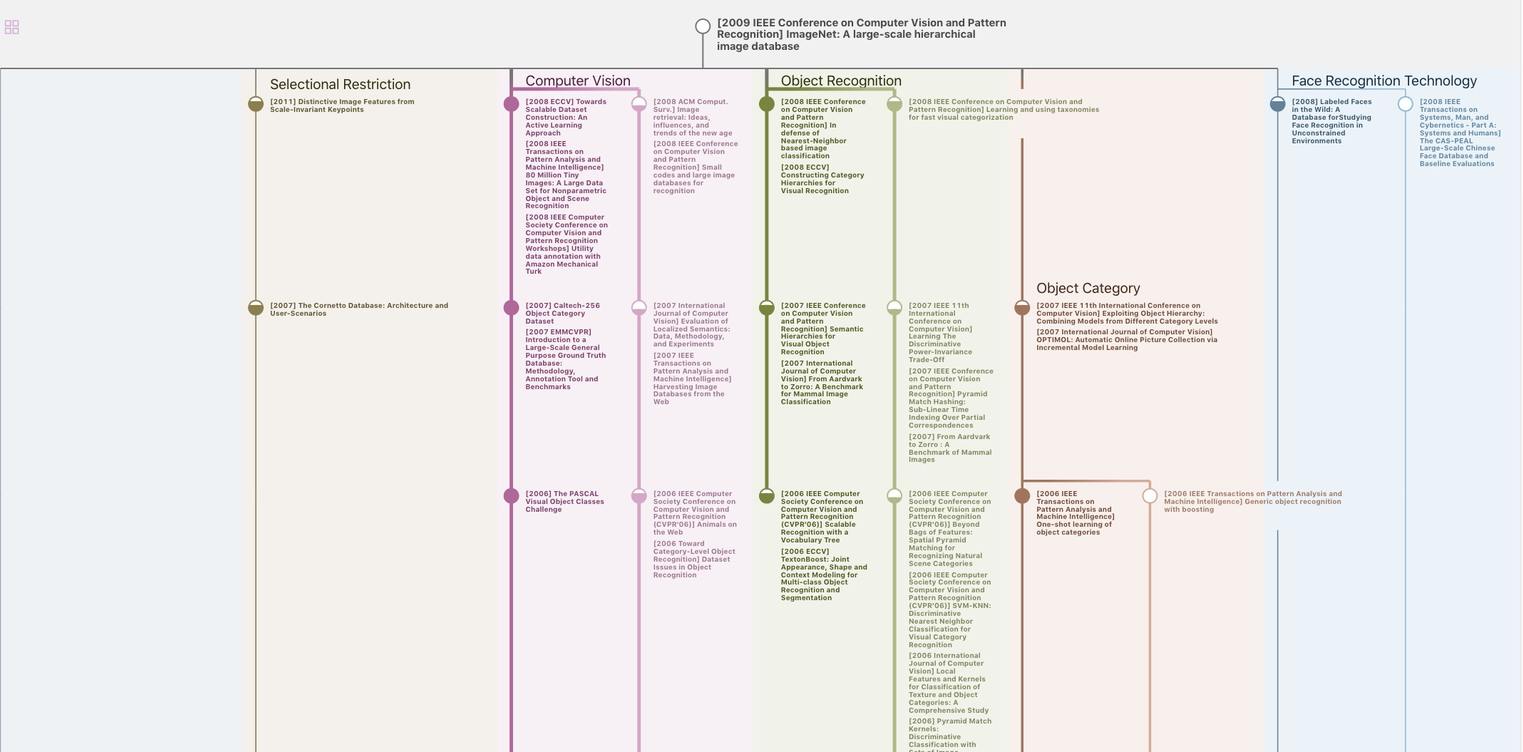
生成溯源树,研究论文发展脉络
Chat Paper
正在生成论文摘要