AdaDQH Optimizer: Evolving from Stochastic to Adaptive by Auto Switch of Precondition Matrix
ICLR 2023(2023)
Abstract
Adaptive optimizers (e.g., Adam) have achieved tremendous success in deep learning. The key component of the optimizer is the precondition matrix, which provides more gradient information and adjusts the step size of each gradient direction. Intuitively, the closer the precondition matrix approximates the Hessian, the faster convergence and better generalization the optimizer can achieve in terms of iterations. However, this performance improvement is usually accompanied by a huge increase in the amount of computation. In this paper, we propose a new optimizer called AdaDQH to achieve better generalization with acceptable computational overhead. The intuitions are the trade-off of the precondition matrix between computation time and approximation of Hessian, and the auto switch of the precondition matrix from Stochastic Gradient Descent (SGD) to the adaptive optimizer. We evaluate AdaDQH on public datasets of Computer Vision (CV), Natural Language Processing (NLP) and Recommendation Systems (RecSys). The experimental results reveal that, compared to the State-Of-The-Art (SOTA) optimizers, AdaDQH can achieve significantly better or highly competitive performance. Furthermore, we analyze how AdaDQH is able to auto switch from stochastic to adaptive and the actual effects in different scenes. The code is available in the supplemental material.
MoreTranslated text
Key words
adaptive optimizer,Hessian approximation,auto switch,precondition matrix,AdaDQH
AI Read Science
Must-Reading Tree
Example
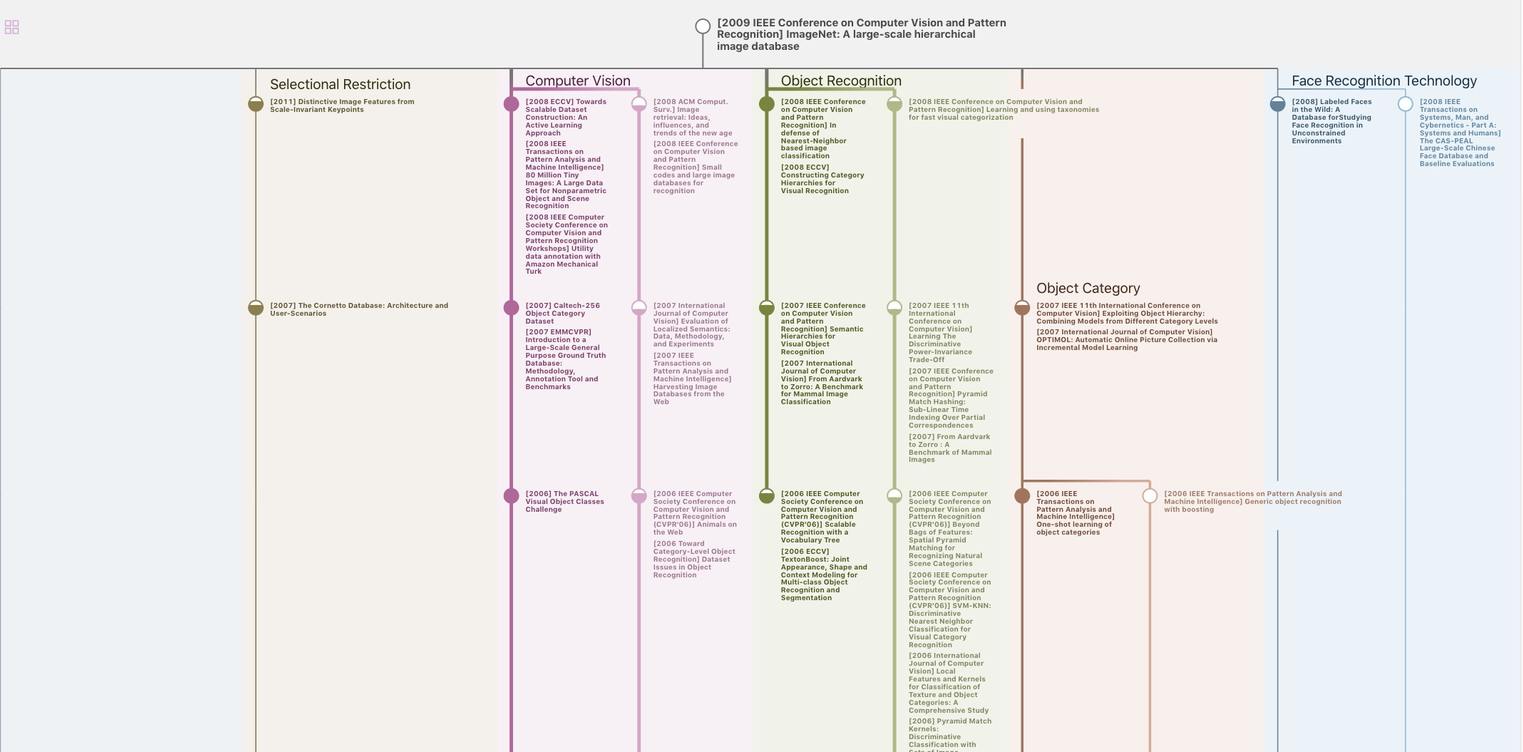
Generate MRT to find the research sequence of this paper
Chat Paper
Summary is being generated by the instructions you defined