Unleashing Mask: Explore the Intrinsic Out-of-distribution Detection Capability
ICLR 2023(2023)
摘要
Out-of-distribution (OOD) detection is an important aspect for safely deploying machine learning models in real-world applications. Previous approaches either design better scoring functions or utilize the knowledge from unknown outliers to equip the well-trained models with the ability of OOD detection. However, few of them explore to excavate the intrinsic OOD detection capability of a given model. In this work, we discover the existence of an intermediate stage of a model trained on in-distribution data having higher OOD detection performance than that of its final stage across different settings, and further identify the critical attribution to be learning with atypical samples. Based on such empirical insights, we propose a new method, Unleashing Mask (UM), to reveal the once-covered detection capability of a given model. To be specific, we utilize the mask to figure out the memorized atypical samples and fine-tune the model to forget them. Extensive experiments have been conducted to characterize and verify the effectiveness of our method.
更多查看译文
关键词
detection
AI 理解论文
溯源树
样例
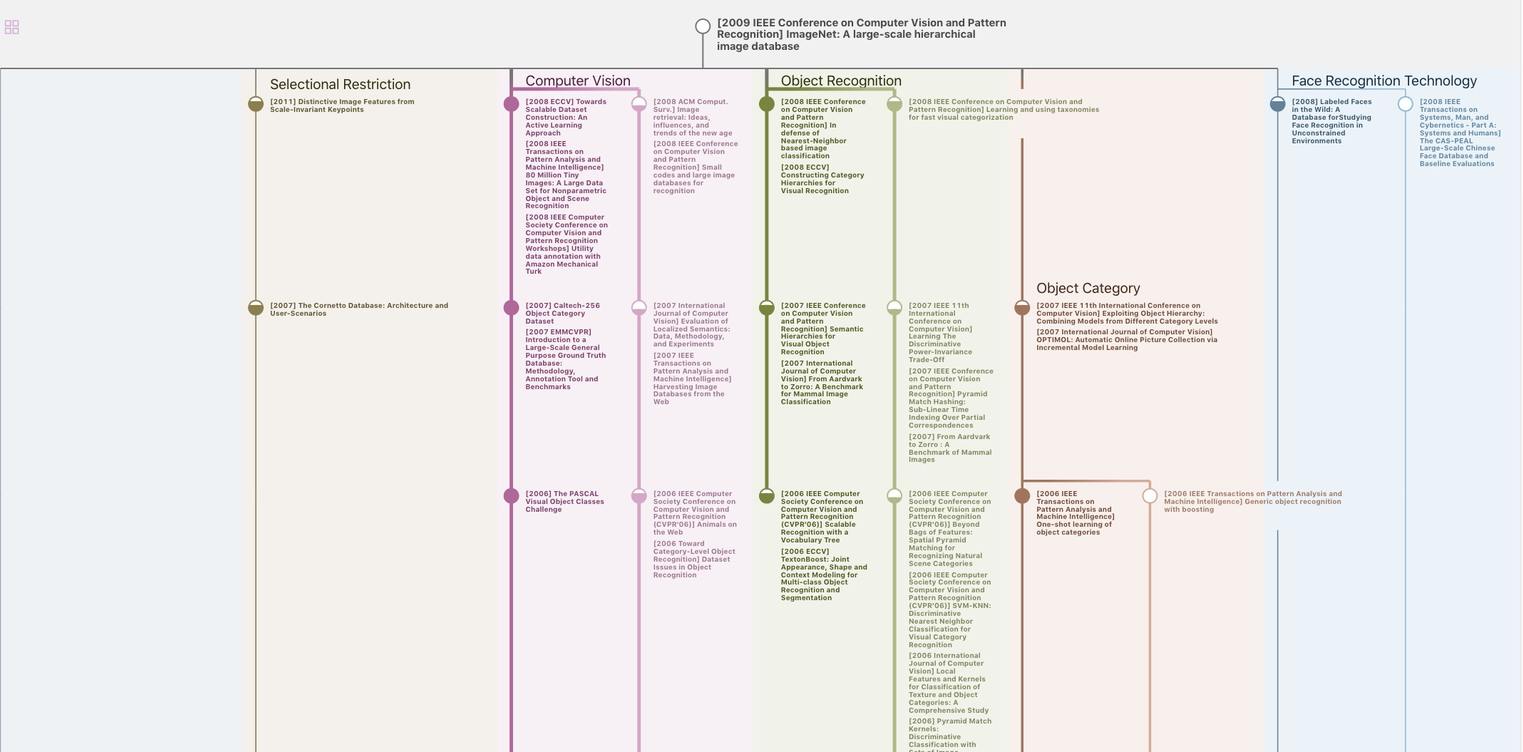
生成溯源树,研究论文发展脉络
Chat Paper
正在生成论文摘要