Variational Imbalanced Regression
ICLR 2023(2023)
摘要
Existing regression models tend to fall short in both accuracy and uncertainty estimation when the label distribution is imbalanced. In this paper, we propose a probabilistic deep learning model, dubbed variational imbalanced regression (VIR), which not only performs well in imbalanced regression but naturally produces reasonable uncertainty estimation as a byproduct. Different from typical variational autoencoders assuming I.I.D. representation (a data point's representation is not directly affected by other data points), our VIR borrows data with similar regression labels to compute the latent representation's variational distribution; furthermore, different from deterministic regression models producing point estimates, VIR predicts the entire normal-inverse-gamma distributions and modulates the associated conjugate distributions to impose probabilistic reweighting on the imbalanced data, thereby providing better uncertainty estimation. Experiments in several real-world datasets show that our VIR can outperform state-of-the-art imbalanced regression models in terms of both accuracy and uncertainty estimation.
更多查看译文
关键词
probabilistic methods,variational inference,imbalanced regression,uncertainty estimation
AI 理解论文
溯源树
样例
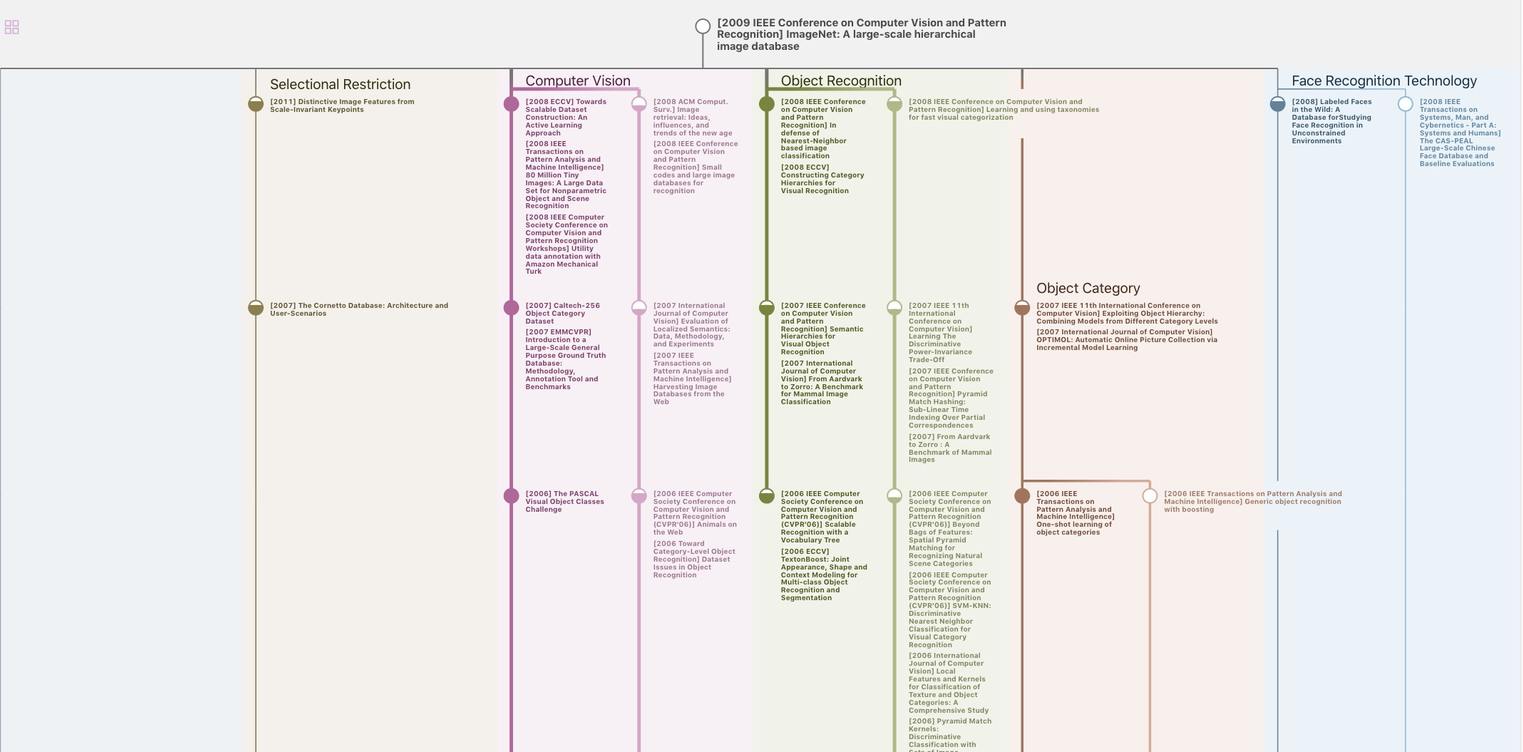
生成溯源树,研究论文发展脉络
Chat Paper
正在生成论文摘要