Interventional Rationalization.
ICLR 2023(2023)
Abstract
Selective rationalizations improve the explainability of neural networks by selecting a subsequence of the input (i.e., rationales) to explain the prediction results. Although existing methods have achieved promising results, they still suffer from adopting the spurious correlations in data (aka., shortcuts) to compose rationales and make predictions. Inspired by the causal theory, in this paper, we develop an interventional rationalization (Inter-RAT) to discover the causal rationales. Specifically, we first analyse the causalities among the input, rationales and results with a structural causal model. Then, we discover spurious correlations between input and rationales, and between rationales and results, respectively, by identifying the confounder in the causalities. Next, based on the backdoor adjustment, we propose a causal intervention method to remove the spurious correlations in input and rationales. Further, we discuss reasons why spurious correlations between the selected rationales and results exist by analysing the limitations of the sparsity constraint in the rationalization, and employ the causal intervention method to remove these correlations. Extensive experimental results on three real-world datasets clearly validate the effectiveness of our proposed method.
MoreTranslated text
Key words
rationalization,causal intervention
AI Read Science
Must-Reading Tree
Example
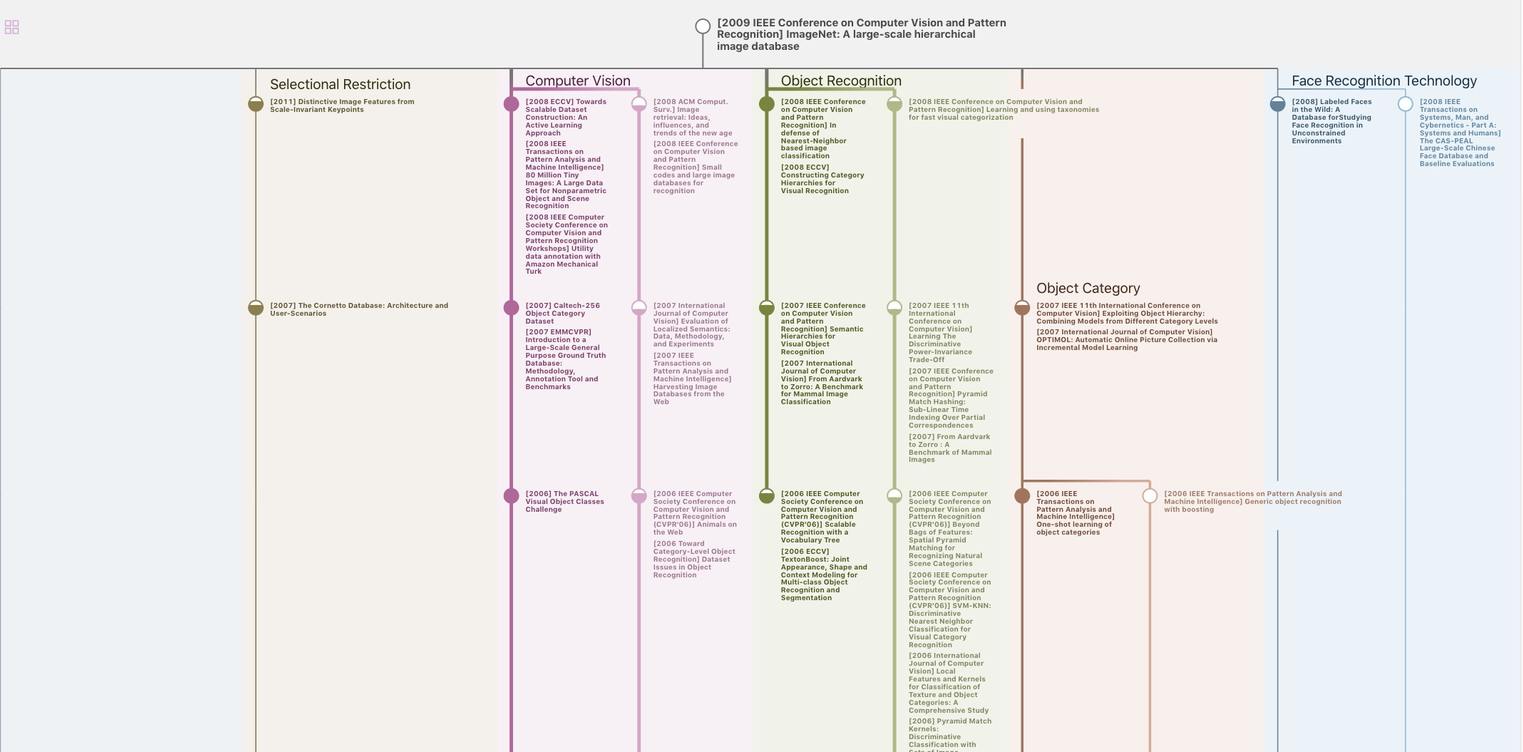
Generate MRT to find the research sequence of this paper
Chat Paper
Summary is being generated by the instructions you defined