An Adaptive Entropy-Regularization Framework for Multi-Agent Reinforcement Learning
ICLR 2023(2023)
摘要
In this paper, we propose an adaptive entropy-regularization framework (ADER) for multi-agent reinforcement learning (RL) to learn the adequate amount of exploration for each agent based on the degree of required exploration. In order to handle instability arising from updating multiple entropy temperature parameters for multiple agents, we disentangle the soft value function into two types: one for pure reward and the other for entropy. By applying multi-agent value factorization to the disentangled value function of pure reward, we obtain a relevant metric to assess the necessary degree of exploration for each agent. Based on this metric, we propose the ADER algorithm based on maximum entropy RL, which controls the necessary level of exploration across agents over time by learning the proper target entropy for each agent. Experimental results show that the proposed scheme significantly outperforms current state-of-the-art multi-agent RL algorithms.
更多查看译文
关键词
Multi-Agent Reinforcement Learning,Entropy Regularization,Exploration-Exploitation Tradeoff
AI 理解论文
溯源树
样例
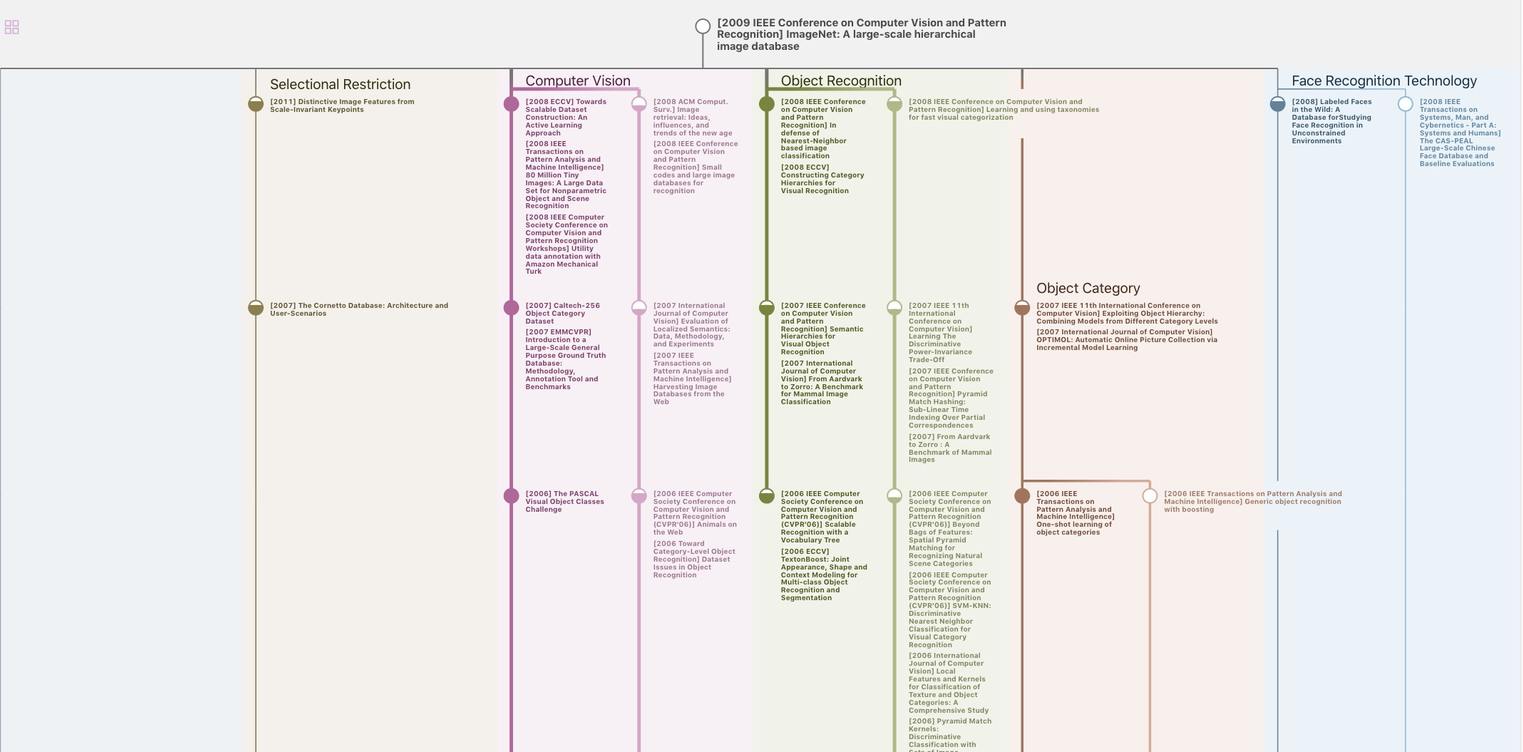
生成溯源树,研究论文发展脉络
Chat Paper
正在生成论文摘要