Newton Losses: Efficiently Including Second-Order Information into Gradient Descent
ICLR 2023(2023)
摘要
We present Newton losses, a method for incorporating second-order information of losses by approximating them with quadratic functions. The presented method is applied only to the loss function and allows training the neural network with gradient descent. As loss functions are usually substantially cheaper to compute than the neural network, Newton losses can be used at a relatively small additional cost. We find that they yield superior performance, especially when applied to non-convex and hard-to-optimize loss functions such as algorithmic losses, which have been popularized in recent research.
更多查看译文
关键词
differentiable algorithms,backpropagation,differentiable
AI 理解论文
溯源树
样例
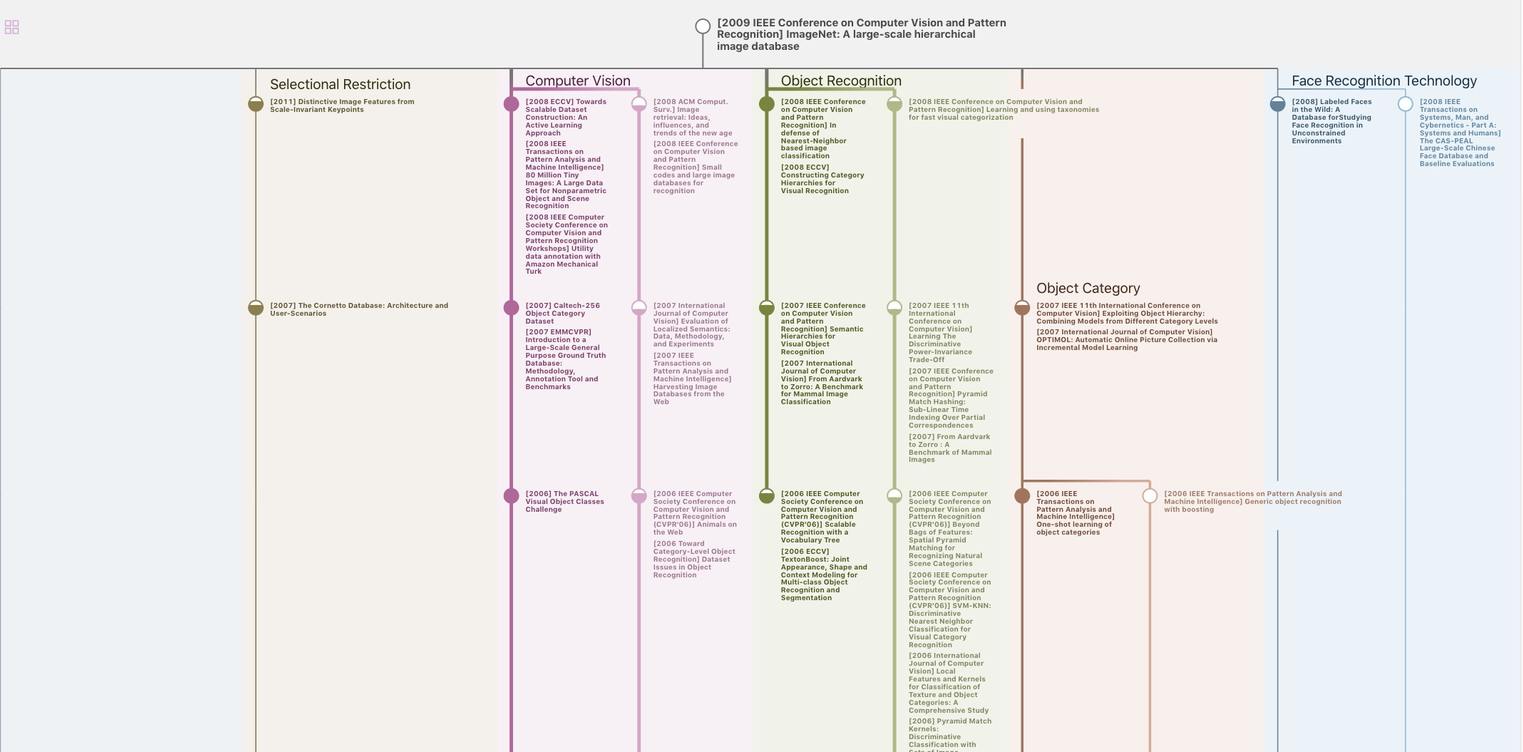
生成溯源树,研究论文发展脉络
Chat Paper
正在生成论文摘要