A New Paradigm for Federated Structure Non-IID Subgraph Learning
ICLR 2023(2023)
Abstract
Federated graph learning (FGL), a distributed training framework for graph neural networks (GNNs) has attracted much attention for breaking the centralized machine learning assumptions. Despite its effectiveness, the differences in data collection perspectives and quality lead to the challenges of heterogeneity, especially the domain-specific graph is partitioned into subgraphs in different institutions. However, existing FGL methods implement graph data augmentation or personalization with community split which follows the cluster homogeneity assumptions. Hence we investigate the above issues and suggest that subgraph heterogeneity is essentially the structure variations. From the observations on FGL, we first define the structure non-independent identical distribution (Non-IID) problem, which presents covariant shift challenges among client-wise subgraphs. Meanwhile, we propose a new paradigm for general federated data settings called Adaptive Federated Graph Learning (AdaFGL). The motivation behind it is to implement adaptive propagation mechanisms based on federated global knowledge and non-params label propagation. We conduct extensive experiments with community split and structure Non-IID settings, our approach achieves state-of-the-art performance on five benchmark datasets.
MoreTranslated text
Key words
graph neural network,federated learning,structure non-iid subgraphs
AI Read Science
Must-Reading Tree
Example
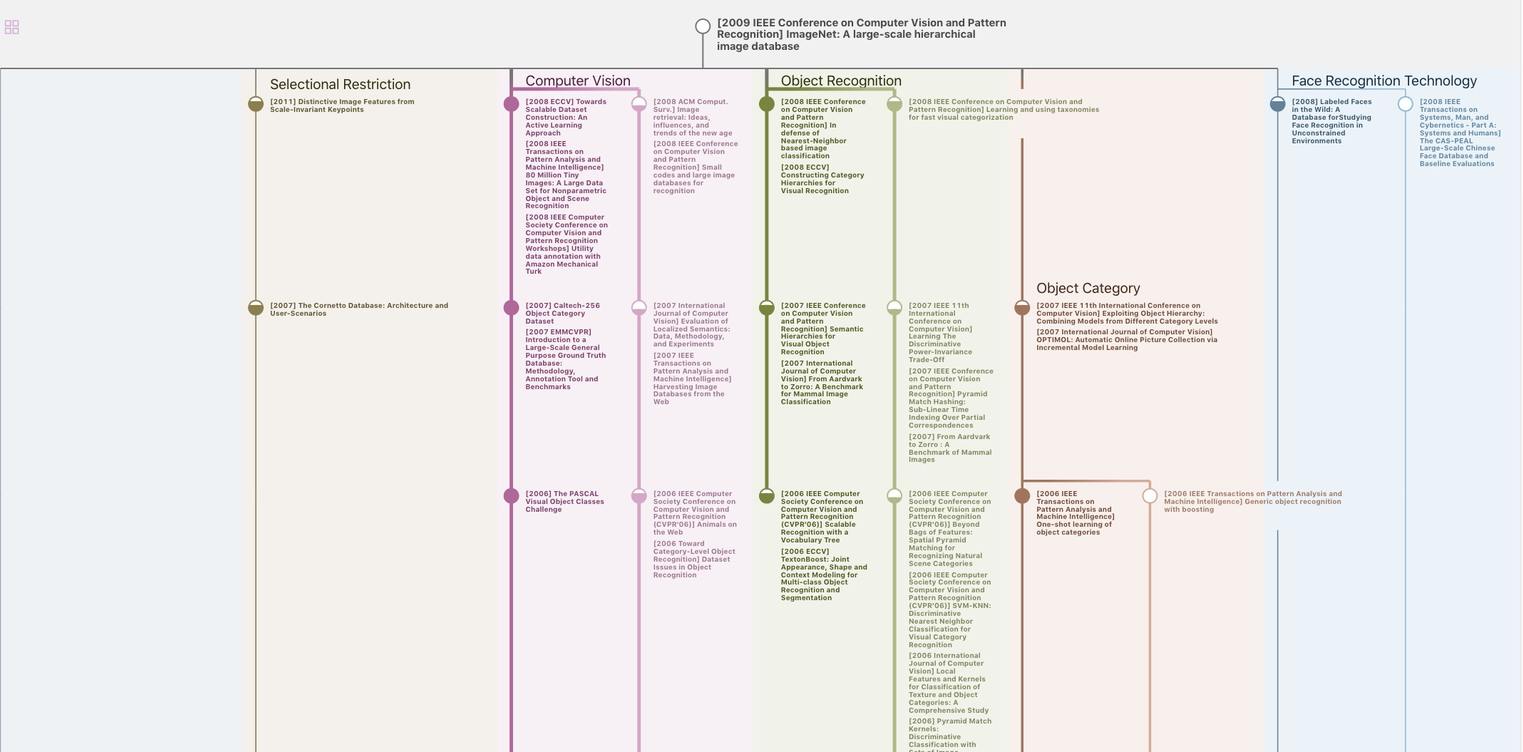
Generate MRT to find the research sequence of this paper
Chat Paper
Summary is being generated by the instructions you defined