NeuralPCG: Learning Preconditioner for Solving Partial Differential Equations with Graph Neural Network
ICLR 2023(2023)
摘要
Fast and accurate partial differential equation (PDE) solvers empower scientific and engineering research. Classic numerical solvers provide unparalleled accuracy but often require extensive computation time. Machine learning solvers are significantly faster but lack convergence and accuracy guarantees. We present Neural-Network-Preconditioned Conjugate Gradient, or NeuralPCG, a novel linear second-order PDE solver that combines the benefits of classic iterative solvers and machine learning approaches. Our key observation is that both neural-network PDE solvers and classic preconditioners excel at obtaining fast but inexact solutions. NeuralPCG proposes to use neural network models to \emph{precondition} PDE systems in classic iterative solvers. Compared with neural-network PDE solvers, NeuralPCG achieves converging and accurate solutions (e.g.,1e-12 precision) by construction. Compared with classic solvers, NeuralPCG is faster via data-driven preconditioners. We demonstrate the efficacy and generalizability of NeuralPCG by conducting extensive experiments on various 2D and 3D linear second-order PDEs.
更多查看译文
关键词
Physics Simulation,Graph Neural Network,Applied Mathematics
AI 理解论文
溯源树
样例
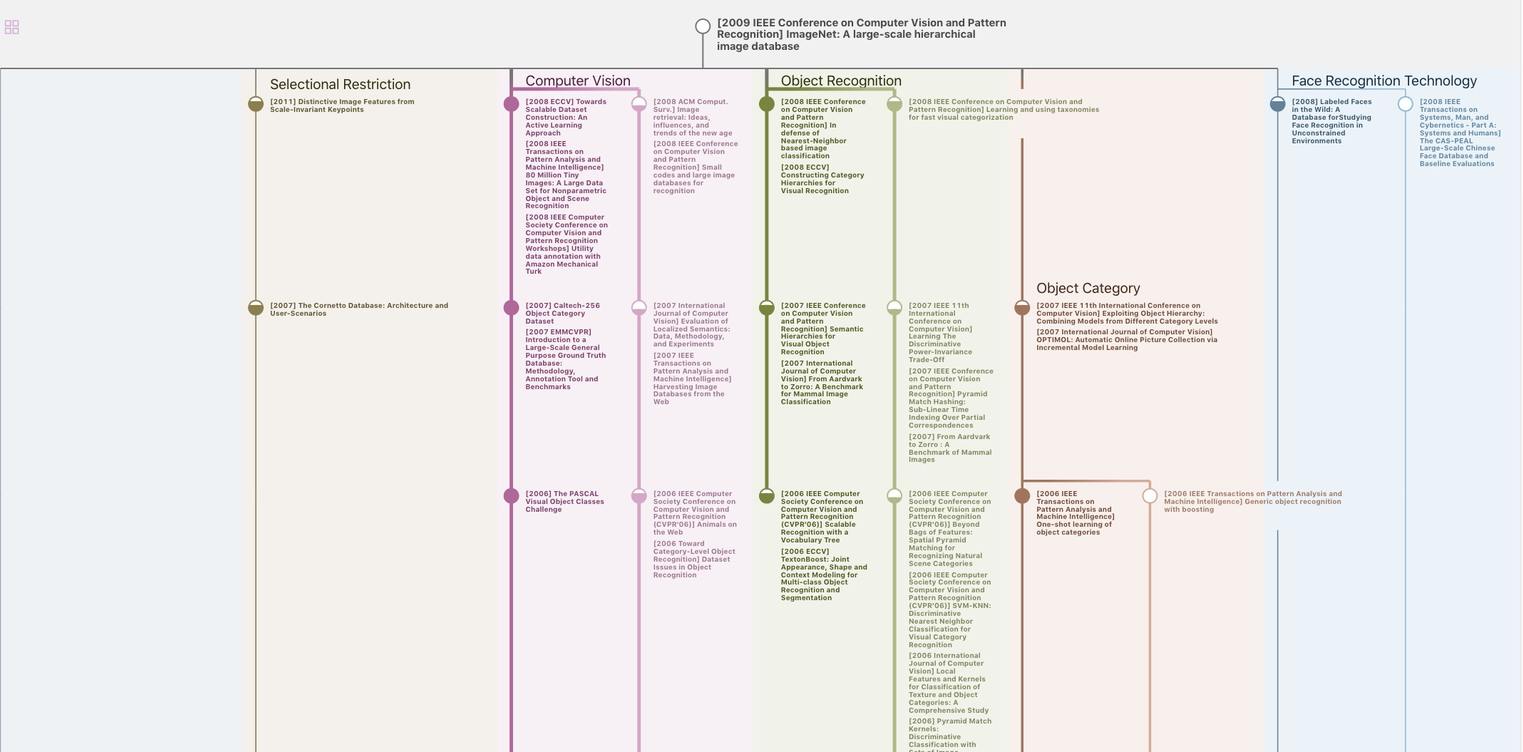
生成溯源树,研究论文发展脉络
Chat Paper
正在生成论文摘要