MemoNav: Working Memory Model for Visual Navigation
ICLR 2023(2023)
摘要
We present MemoNav, a novel memory model for image-goal navigation, which utilizes a working memory-inspired pipeline to improve navigation performance. Specifically, the node features on the topological map are stored in the short-term memory (STM), as these features are dynamically updated. The MemoNav retains the informative fraction of the STM via a forgetting module to improve navigation efficiency. To learn a global representation of 3D scenes, we introduce long-term memory (LTM) that continuously aggregates the STM. Afterward, a graph attention module encodes the retained STM and the LTM to generate working memory (WM). After encoding, the WM contains the informative features in the retained STM and the scene-level feature in the LTM and is finally used to generate actions. Consequently, the synergy of these three types of memory increases navigation performance by selectively retaining goal-relevant information and learning a high-level scene feature. When evaluated on multi-goal tasks, the MemoNav outperforms the SoTA methods at all difficulty levels in both Gibson and Matterport3D scenes. The MemoNav also achieves consistent improvements on traditional 1-goal tasks. Moreover, the qualitative results show that our model is less likely to be trapped in a deadlock.
更多查看译文
关键词
Image-Goal Navigation,Memory mechanism,Embodied visual navigation,Embodied AI
AI 理解论文
溯源树
样例
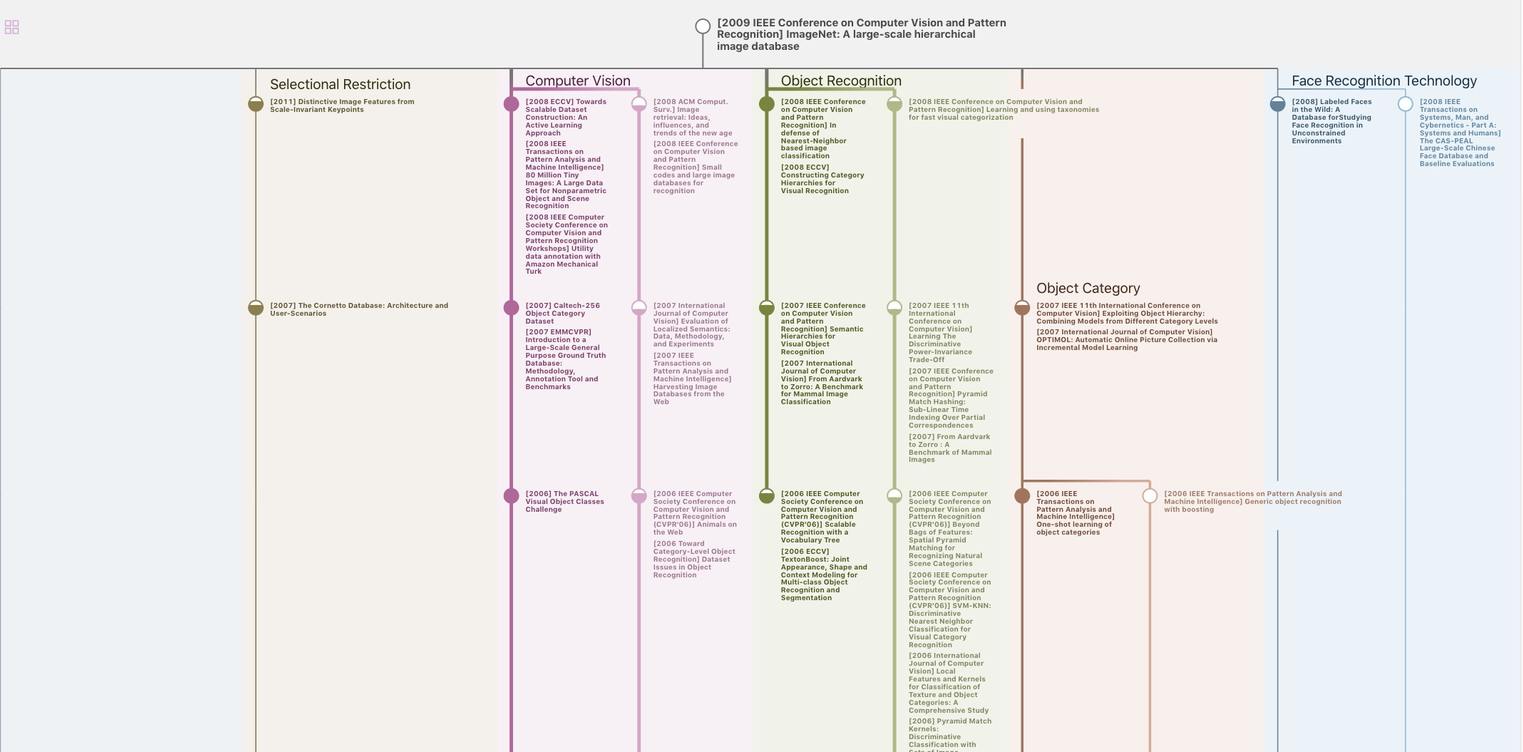
生成溯源树,研究论文发展脉络
Chat Paper
正在生成论文摘要