Test-time Adaptation for Better Adversarial Robustness
ICLR 2023(2023)
摘要
Standard adversarial training and its variants have been widely adopted in practice to achieve robustness against adversarial attacks. However, we show in this work that such an approach does not necessarily achieve near optimal generalization performance on test samples. Specifically it is shown that under suitable assumptions, Bayesian optimal robust estimator requires test-time adaptation, and such adaptation can lead to significant performance boost over standard adversarial training. Motivated by this observation, we propose a practically easy to implement method to improve the generalization performance of adversarially-trained networks via an additional self-supervised test-time adaptation step. We further employs a meta adversarial training method to find a good starting point for test-time adaptation, which incorporates the test-time adaptation procedure into the training phase and it strengthens the correlation between the pre-text tasks in self-supervised learning and the original classification task. Extensive empirical experiments on CIFAR10, STL10 and Tiny ImageNet using several different self-supervised tasks show that our method consistently improves the robust accuracy of standard adversarial training under different white-box and black-box attack strategies.
更多查看译文
AI 理解论文
溯源树
样例
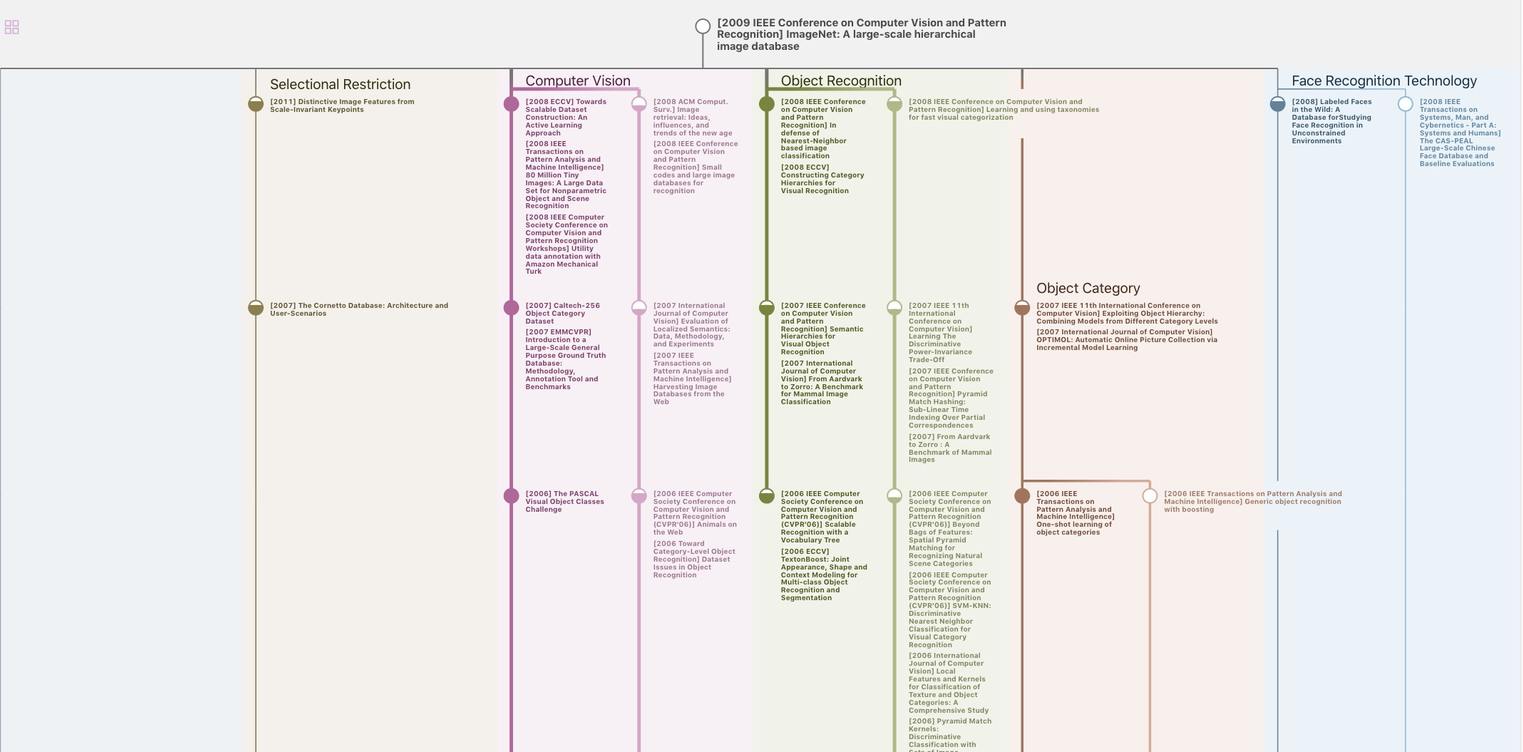
生成溯源树,研究论文发展脉络
Chat Paper
正在生成论文摘要