N-Student Learning: An Approach to Model Uncertainty and Combat Overfitting
ICLR 2023(2023)
摘要
This work presents N-Student Learning, a pseudo-label based multi-network training setup that can be applied to nearly any supervised learning architecture in order to help combat the problem of overfitting and control the way in which a network models uncertainty in the data. The effectiveness of N-Student Learning relies on the idea that a network's predictions on unseen data are largely independent of any instance-dependent noise in the labels. In N-Student Learning, each student network is assigned a subset of the training dataset such that no data point is in every student's training subset. Unbiased pseudo-labels can thus be generated for every data point in the training set by taking the predictions of appropriate student networks. Training on these unbiased pseudo-labels minimizes the extent to which each network overfits to instance-dependent noise in the data. Furthermore, based on prior knowledge of the domain, we can control how the networks learn to model uncertainty that is present in the dataset by adjusting the way that pseudo-labels are generated. While this method is largely inspired by the general problem of overfitting, a natural application is found in the problem of classification with noisy labels — a domain where overfitting is a significant concern. After developing intuition through a toy classification task, we proceed to demonstrate that N-Student Learning performs favorably on benchmark datasets when compared to state-of-the-art methods in the problem of classification with noisy labels.
更多查看译文
关键词
Noisy Labels,Pseudo-Labels,Overfitting,Supervised Learning
AI 理解论文
溯源树
样例
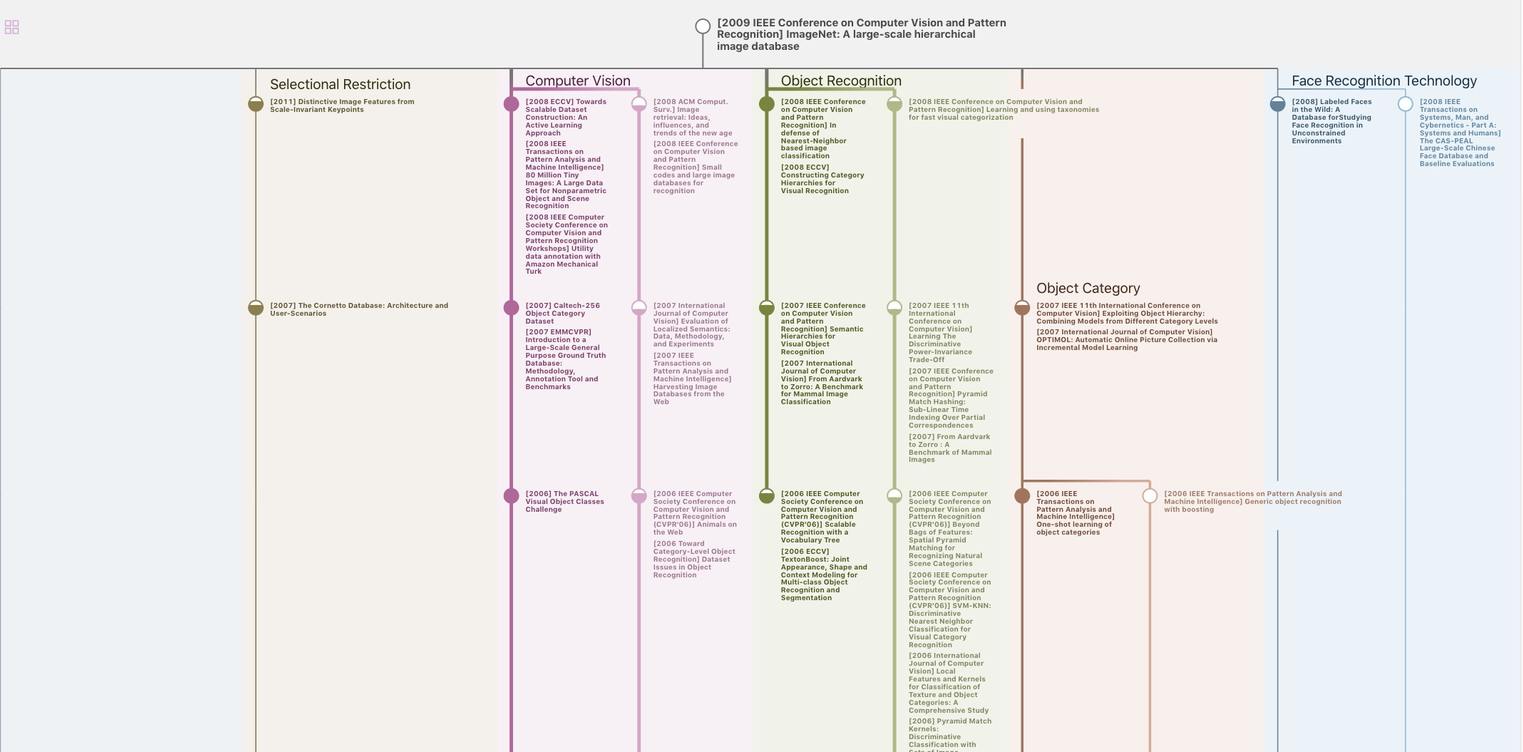
生成溯源树,研究论文发展脉络
Chat Paper
正在生成论文摘要