On the robustness of self-supervised models for generative spoken language modeling
ICLR 2023(2023)
摘要
Self-supervised representations have been extensively studied for discriminative and generative tasks. However, their robustness capabilities have not been extensively investigated. This work focuses on self-supervised representations for spoken generative language models. First, we empirically demonstrate how current state-of-the-art speech representation models lack robustness to basic signal variations that do not alter the spoken information. To overcome this, we propose an effective and efficient method to learn robust self-supervised speech representation for generative spoken language modeling. The proposed approach is based on applying a set of signal transformations to the speech signal and optimizing the model using an iterative pseudo-labeling scheme. Our method significantly improves over the evaluated baselines when considering encoding metrics. We additionally evaluate our method on the speech-to-speech translation task. We consider Spanish-English and French-English conversions and empirically demonstrate the benefits of following the proposed approach.
更多查看译文
关键词
robustness,speech,language modeling,spoken language modeling
AI 理解论文
溯源树
样例
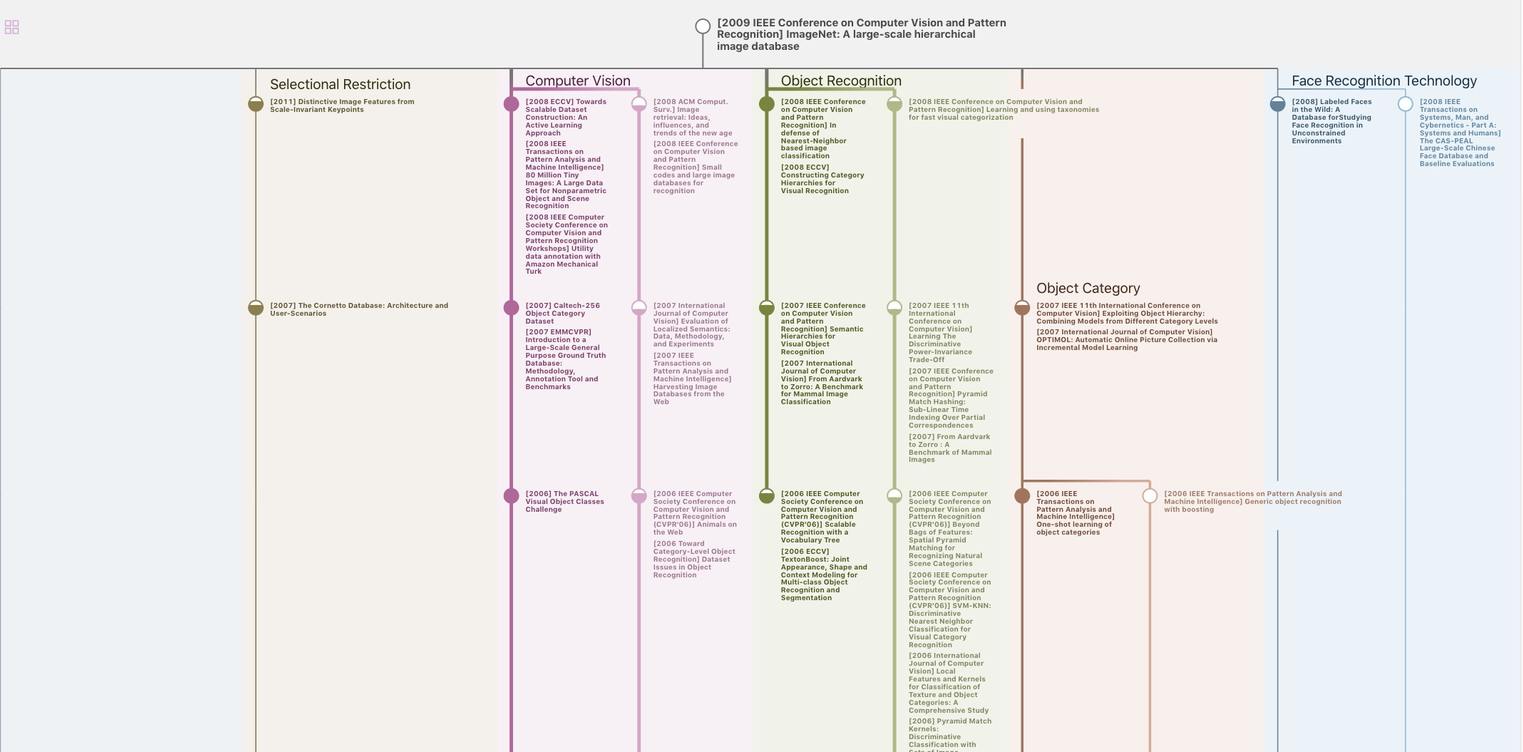
生成溯源树,研究论文发展脉络
Chat Paper
正在生成论文摘要