CBP-QSNN: Spiking Neural Networks Quantized Using Constrained Backpropagation
IEEE JOURNAL ON EMERGING AND SELECTED TOPICS IN CIRCUITS AND SYSTEMS(2023)
摘要
Spiking Neural Networks (SNNs) support sparse event-based data processing at high power efficiency when implemented in event-based neuromorphic processors. However, the limited on- chip memory capacity of neuromorphic processors strictly delimits the depth and width of SNNs implemented. A direct solution is the use of quantized SNNs (QSNNs) in place of SNNs with FP32 weights. To this end, we propose a method to quantize the weights using constrained backpropagation (CBP) with the Lagrangian function (conventional loss function plus well-defined weight-constraint functions) as an objective function. This work utilizes CBP as a post-training algorithm for deep SNNs pre-trained using various state-of-the-art methods including direct training (TSSL-BP, STBP, and surrogate gradient) and DNN-to-SNN conversion (SNN-Calibration), validating CBP as a general framework for QSNNs. CBP-QSNNs highlight their high accuracy insomuch as the degradation of accuracy on CIFAR-10, DVS128 Gesture, and CIFAR10-DVS in the worst case is less than 1%. Particularly, CBP-QSNNs for SNN-Calibration-pretrained SNNs on CIFAR-100 highlight an unexpected large increase in accuracy by 3.72% while using small weight-memory (3.5% of the FP32 case).
更多查看译文
关键词
Quantized spiking neural network,constrained backpropagation,binary weight,Lagrange multiplier method,weight constraint
AI 理解论文
溯源树
样例
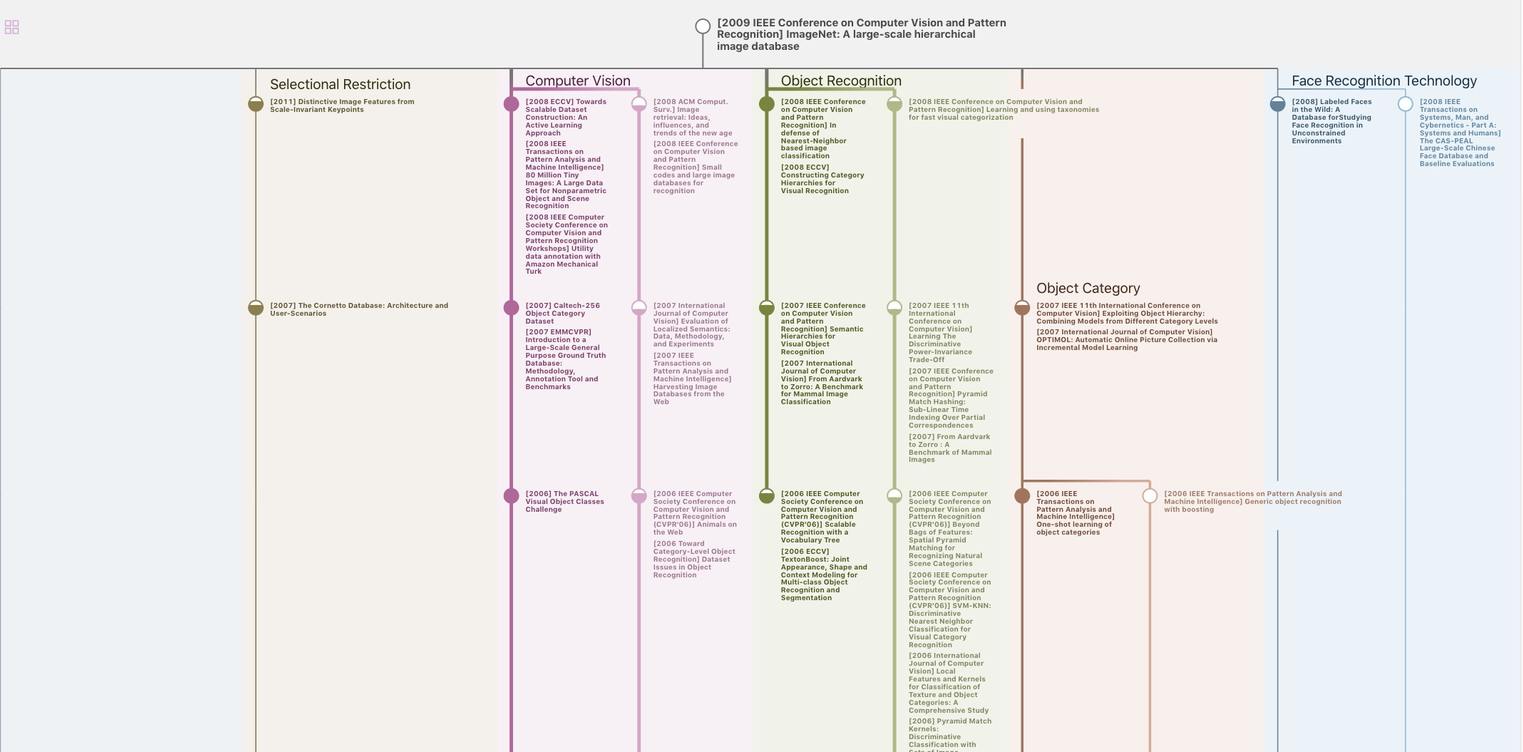
生成溯源树,研究论文发展脉络
Chat Paper
正在生成论文摘要